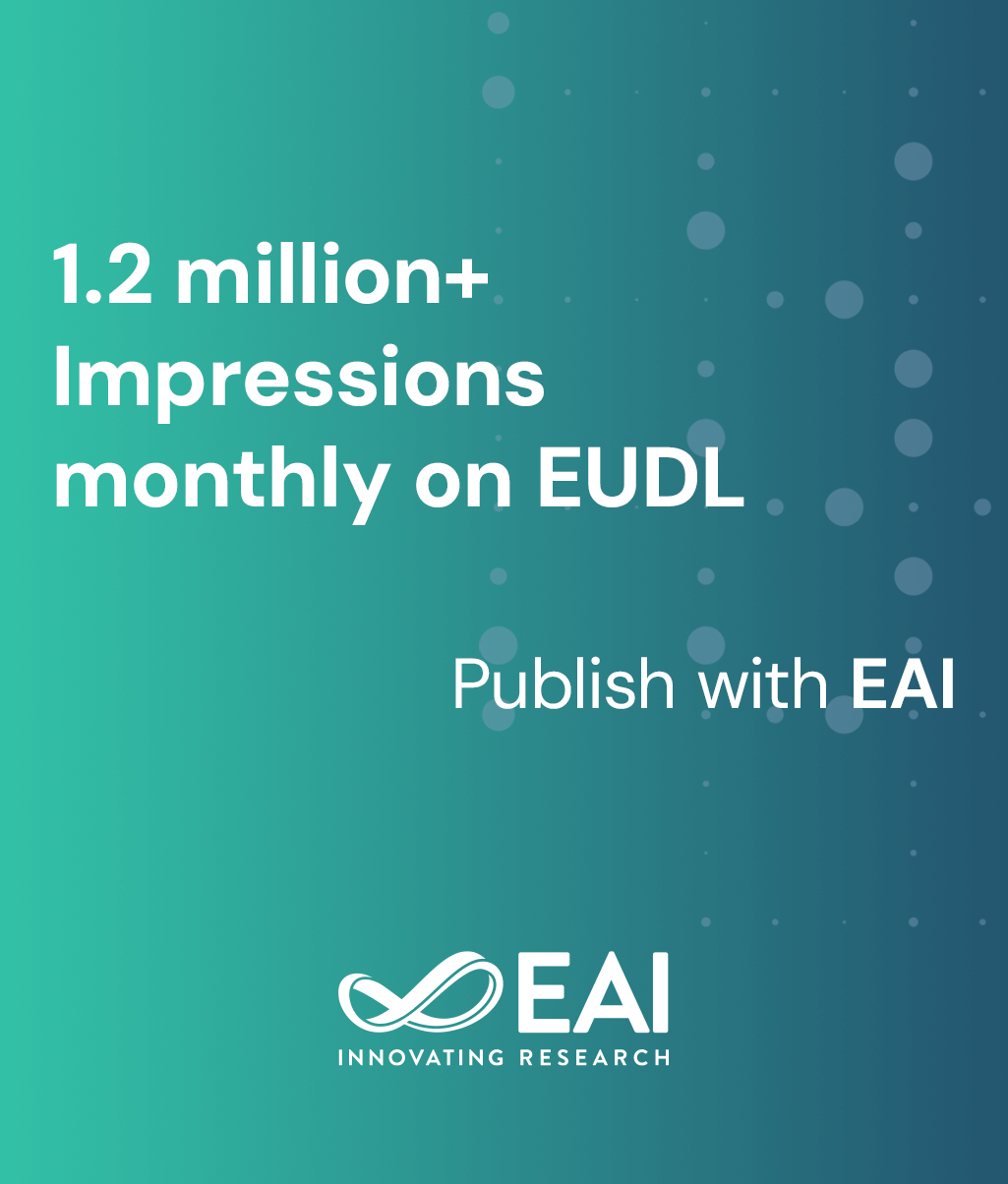
Research Article
Machine Learning in Cybersecurity: Advanced Detection and Classification Techniques for Network Traffic Environments
@ARTICLE{10.4108/eetinis.v11i3.5237, author={Samer El Hajj Hassan and Nghia Duong-Trung}, title={Machine Learning in Cybersecurity: Advanced Detection and Classification Techniques for Network Traffic Environments}, journal={EAI Endorsed Transactions on Industrial Networks and Intelligent Systems}, volume={11}, number={3}, publisher={EAI}, journal_a={INIS}, year={2024}, month={7}, keywords={Machine Learning, Cybersecurity, Network Analysis, Anomaly Detection, Data Security, Traffic Classification, Network Optimization, Traffic Volume}, doi={10.4108/eetinis.v11i3.5237} }
- Samer El Hajj Hassan
Nghia Duong-Trung
Year: 2024
Machine Learning in Cybersecurity: Advanced Detection and Classification Techniques for Network Traffic Environments
INIS
EAI
DOI: 10.4108/eetinis.v11i3.5237
Abstract
In the digital age, the integrity of business operations and the smoothness of their execution heavily depend on cybersecurity and network efficiency. The need for robust solutions to prevent cyber threats and enhance network functionality has never been more critical. This research aims to utilize machine learning (ML) techniques for the meticulous analysis of network traffic, with the dual goals of detecting anomalies and categorizing network activities to bolster security and performance. Employing a detailed methodology, this study begins with data preparation and progresses through to the deployment of advanced ML models, including logistic regression, decision trees, and ensemble learning techniques. This approach ensures the accuracy of the analysis and facilitates a nuanced understanding of network dynamics. Our findings indicate a notable enhancement in identifying network inefficiencies and in the more accurate classification of network traffic. The application of ML models significantly reduces network delays and bottlenecks by providing a strong defence strategy against cyber threats and network shortcomings, thereby improving user satisfaction, and boosting the organizational reputation as a secure and effective service layer. Conclusively, the research highlights the pivotal role of machine learning in network traffic analysis, offering innovative insights and fresh perspectives on anomaly detection and the identification of malicious activities. It lays a foundation for future explorations and acts as an evaluation benchmark in the fields of cybersecurity and network management.
Copyright © 2024 S. El Hajj Hassan et al., licensed to EAI. This is an open access article distributed under the terms of the CC BY-NC-SA 4.0, which permits copying, redistributing, remixing, transformation, and building upon the material in any medium so long as the original work is properly cited.