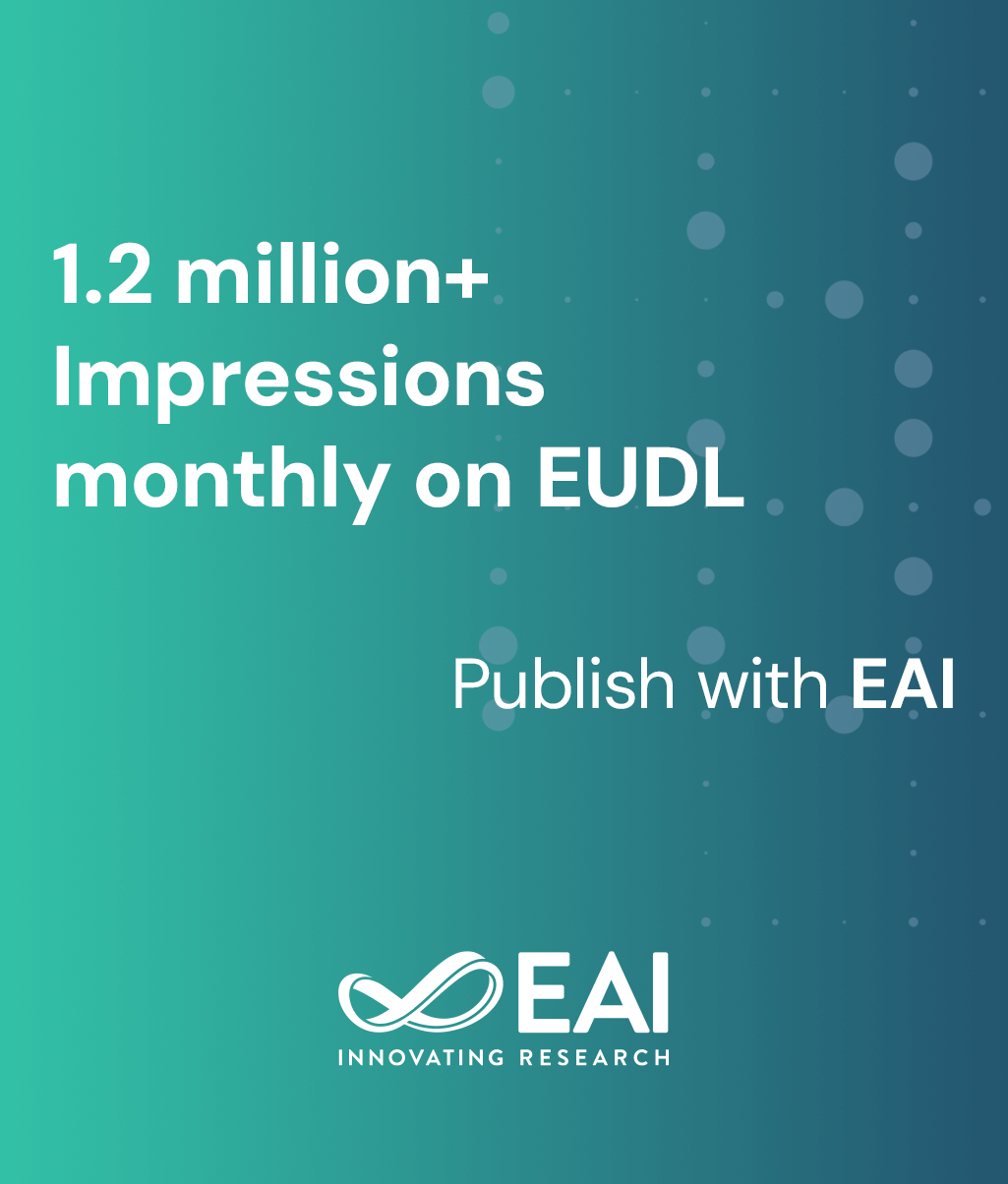
Research Article
ViMedNER: A Medical Named Entity Recognition Dataset for Vietnamese
@ARTICLE{10.4108/eetinis.v11i3.5221, author={Pham Van Duong and Tien-Dat Trinh and Minh-Tien Nguyen and Huy-The Vu and Minh Chuan Pham and Tran Manh Tuan and Le Hoang Son}, title={ViMedNER: A Medical Named Entity Recognition Dataset for Vietnamese}, journal={EAI Endorsed Transactions on Industrial Networks and Intelligent Systems}, volume={11}, number={4}, publisher={EAI}, journal_a={INIS}, year={2024}, month={7}, keywords={Named entity recognition, Vietnamese corpus, Medical text, Pre-trained language model}, doi={10.4108/eetinis.v11i3.5221} }
- Pham Van Duong
Tien-Dat Trinh
Minh-Tien Nguyen
Huy-The Vu
Minh Chuan Pham
Tran Manh Tuan
Le Hoang Son
Year: 2024
ViMedNER: A Medical Named Entity Recognition Dataset for Vietnamese
INIS
EAI
DOI: 10.4108/eetinis.v11i3.5221
Abstract
Named entity recognition (NER) is one of the most important tasks in natural language processing, which identifies entity boundaries and classifies them into pre-defined categories. In literature, NER systems have been developed for various languages but limited works have been conducted for Vietnamese. This mainly comes from the limitation of available and high-quality annotated data, especially for specific domains such as medicine and healthcare. In this paper, we introduce a new medical NER dataset, named ViMedNER, for recognizing Vietnamese medical entities. Unlike existing works designed for common or too-specific entities, we focus on entity types that can be used in common diagnostic and treatment scenarios, including disease names, the symptoms of the diseases, the cause of the diseases, the diagnostic, and the treatment. These entities facilitate the diagnosis and treatment of doctors for common diseases. Our dataset is collected from four well-known Vietnamese websites that are professional in terms of drag selling and disease diagnostics and annotated by domain experts with high agreement scores. To create benchmark results, strong NER baselines based on pre-trained language models including PhoBERT, XLM-R, ViDeBERTa, ViPubMedDeBERTa, and ViHealthBERT are implemented and evaluated on the dataset. Experiment results show that the performance of XLM-R is consistently better than that of the other pre-trained language models. Furthermore, additional experiments are conducted to explore the behavior of the baselines and the characteristics of our dataset.
Copyright © 2024 P. V. Duong et al., licensed to EAI. This is an open access article distributed under the terms of the CC BY-NC-SA 4.0, which permits copying, redistributing, remixing, transformation, and building upon the material in any medium so long as the original work is properly cited.