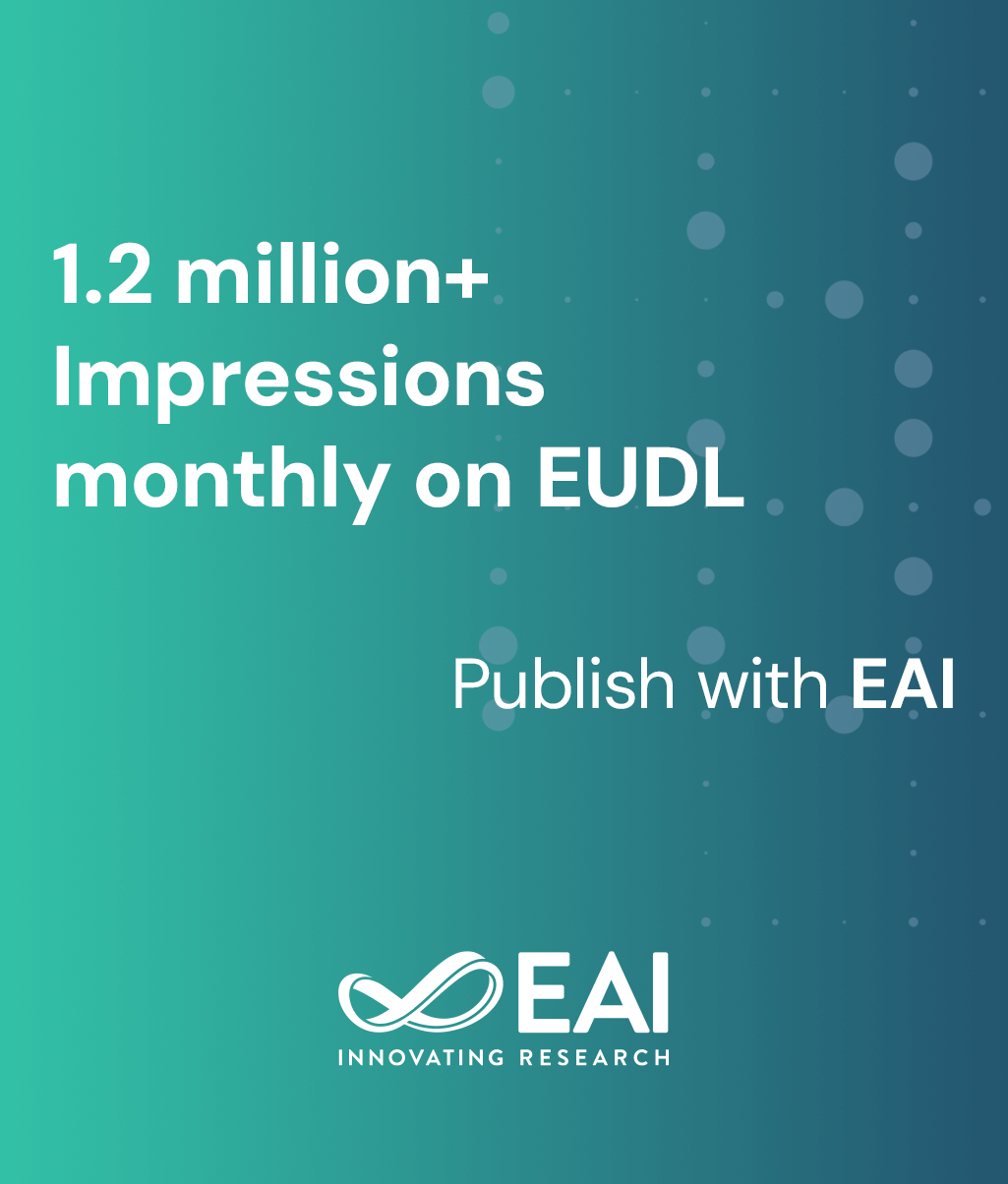
Research Article
ERKT-Net: Implementing Efficient and Robust Knowledge Distillation for Remote Sensing Image Classification
@ARTICLE{10.4108/eetinis.v11i3.4748, author={Huaxiang Song and Yafang Li and Xiaowen Li and Yuxuan Zhang and Yangyan Zhu and Yong Zhou}, title={ERKT-Net: Implementing Efficient and Robust Knowledge Distillation for Remote Sensing Image Classification}, journal={EAI Endorsed Transactions on Industrial Networks and Intelligent Systems}, volume={11}, number={3}, publisher={EAI}, journal_a={INIS}, year={2024}, month={12}, keywords={ERKT-Net, Variance-Suppression Strategy, Knowledge Distillation, Remote Sensing Image Classification, Deep Learning}, doi={10.4108/eetinis.v11i3.4748} }
- Huaxiang Song
Yafang Li
Xiaowen Li
Yuxuan Zhang
Yangyan Zhu
Yong Zhou
Year: 2024
ERKT-Net: Implementing Efficient and Robust Knowledge Distillation for Remote Sensing Image Classification
INIS
EAI
DOI: 10.4108/eetinis.v11i3.4748
Abstract
The classification of Remote Sensing Images (RSIs) poses a significant challenge due to the presence of clustered ground objects and noisy backgrounds. While many approaches rely on scaling models to enhance accuracy, the deployment of RSI classifiers often requires substantial computational and storage resources, thus necessitating the use of lightweight algorithms. In this paper, we present an efficient and robust knowledge transfer network named ERKT-Net, which is designed to provide a lightweight yet accurate Convolutional Neural Network (CNN) classifier. This method utilizes innovative yet simple concepts to better accommodate the inherent nature of RSIs, thereby significantly improving the efficiency and robustness of traditional Knowledge Distillation (KD) techniques developed on ImageNet-1K. We evaluated ERKT-Net on three benchmark RSI datasets and found that it demonstrated superior accuracy and a very compact volume compared to 40 other advanced methods published between 2020 and 2023. On the most challenging NWPU45 dataset, ERKT-Net outperformed other KD-based methods with a maximum Overall Accuracy (OA) value of 22.4%. Using the same criterion, it also surpassed the first-ranked multi-model method with a minimum OA value of 0.7 but presented at least an 82% reduction in parameters. Furthermore, ablation experiments indicated that our training approach has significantly improved the efficiency and robustness of classic DA techniques. Notably, it can reduce the time expenditure in the distillation phase by at least 80%, with a slight sacrifice in accuracy. This study confirmed that a logit-based KD technique can be more efficient and effective in developing lightweight yet accurate classifiers, especially when the method is tailored to the inherent characteristics of RSIs.
Copyright © 2024 Song et al., licensed to EAI. This is an open access article distributed under the terms of theCC BY-NC-SA 4.0, which permits copying, redistributing, remixing, transformation, and building upon the material in any medium so long as the original work is properly cited.