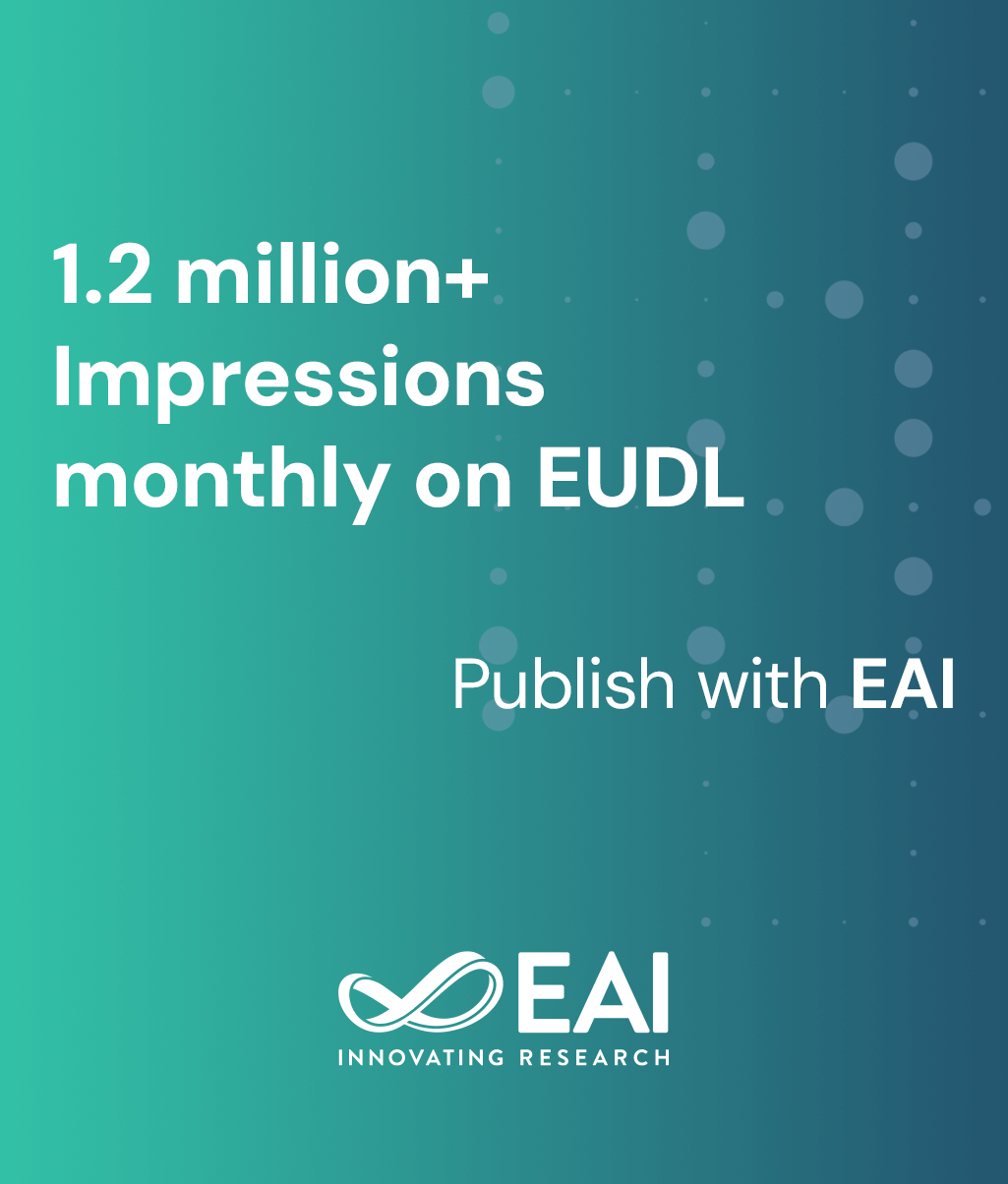
Research Article
Cyberbullying Text Identification based on Deep Learning and Transformer-based Language Models
@ARTICLE{10.4108/eetinis.v11i1.4703, author={Khalid Saifullah and Muhammad Ibrahim Khan and Suhaima Jamal and Iqbal H. Sarker}, title={Cyberbullying Text Identification based on Deep Learning and Transformer-based Language Models}, journal={EAI Endorsed Transactions on Industrial Networks and Intelligent Systems}, volume={11}, number={1}, publisher={EAI}, journal_a={INIS}, year={2024}, month={2}, keywords={Cyberbullying, large language modeling, deep learning, transformers models, natural language processing, NLP, fine tuning, OOV, harmful messages}, doi={10.4108/eetinis.v11i1.4703} }
- Khalid Saifullah
Muhammad Ibrahim Khan
Suhaima Jamal
Iqbal H. Sarker
Year: 2024
Cyberbullying Text Identification based on Deep Learning and Transformer-based Language Models
INIS
EAI
DOI: 10.4108/eetinis.v11i1.4703
Abstract
In the contemporary digital age, social media platforms like Facebook, Twitter, and YouTube serve as vital channels for individuals to express ideas and connect with others. Despite fostering increased connectivity, these platforms have inadvertently given rise to negative behaviors, particularly cyberbullying. While extensive research has been conducted on high-resource languages such as English, there is a notable scarcity of resources for low-resource languages like Bengali, Arabic, Tamil, etc., particularly in terms of language modeling. This study addresses this gap by developing a cyberbullying text identification system called BullyFilterNeT tailored for social media texts, considering Bengali as a test case. The intelligent BullyFilterNeT system devised overcomes Out-of-Vocabulary (OOV) challenges associated with non-contextual embeddings and addresses the limitations of context-aware feature representations. To facilitate a comprehensive understanding, three non-contextual embedding models GloVe, FastText, and Word2Vec are developed for feature extraction in Bengali. These embedding models are utilized in the classification models, employing three statistical models (SVM, SGD, Libsvm), and four deep learning models (CNN, VDCNN, LSTM, GRU). Additionally, the study employs six transformer-based language models: mBERT, bELECTRA, IndicBERT, XML-RoBERTa, DistilBERT, and BanglaBERT, respectively to overcome the limitations of earlier models. Remarkably, BanglaBERT-based BullyFilterNeT achieves the highest accuracy of 88.04% in our test set, underscoring its effectiveness in cyberbullying text identification in the Bengali language.
Copyright © 2024 K. Saifullah et al., licensed to EAI. This is an open access article distributed under the terms of the CC BY-NC-SA 4.0, which permits copying, redistributing, remixing, transformation, and building upon the material in any medium so long as the original work is properly cited.