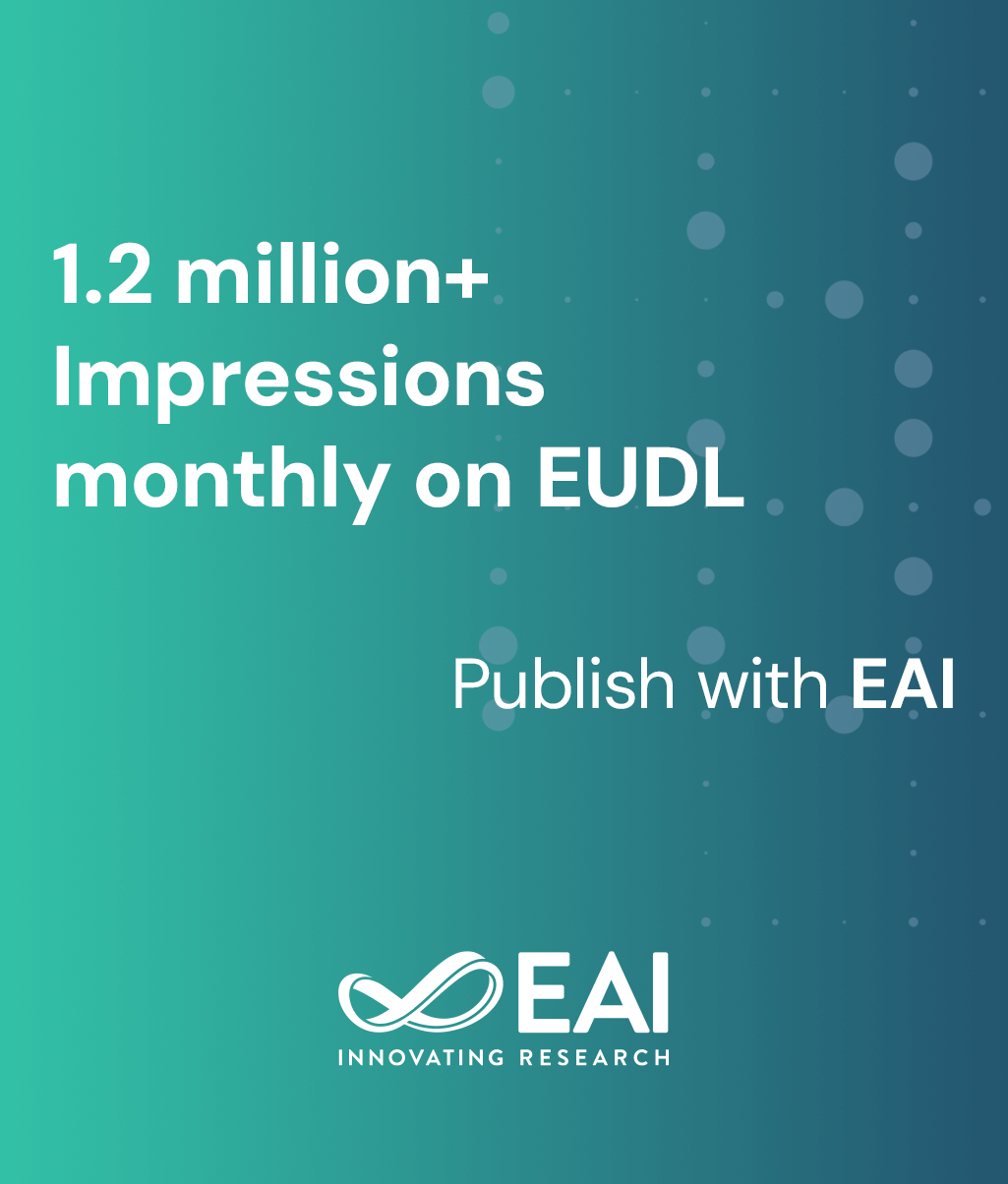
Research Article
SHELF: Combination of Shape Fitting and Heatmap Regression for Landmark Detection in Human Face
@ARTICLE{10.4108/eetinis.v10i3.3863, author={Ngo Thi Ngoc Quyen and Tran Duy Linh and Vu Hong Phuc and Nguyen Van Nam}, title={SHELF: Combination of Shape Fitting and Heatmap Regression for Landmark Detection in Human Face}, journal={EAI Endorsed Transactions on Industrial Networks and Intelligent Systems}, volume={10}, number={3}, publisher={EAI}, journal_a={INIS}, year={2023}, month={9}, keywords={facial landmarks, heatmap regression, shape fitting, coordination regression}, doi={10.4108/eetinis.v10i3.3863} }
- Ngo Thi Ngoc Quyen
Tran Duy Linh
Vu Hong Phuc
Nguyen Van Nam
Year: 2023
SHELF: Combination of Shape Fitting and Heatmap Regression for Landmark Detection in Human Face
INIS
EAI
DOI: 10.4108/eetinis.v10i3.3863
Abstract
Today, facial emotion recognition is widely adopted in many intelligent applications including the driver monitoring system, the smart customer care as well as the e-learning system. In fact, the human emotions can be well represented by facial landmarks which are hard to be detected from images, due to the high number of discrete landmarks, the variation of shapes and poses of the human face in real world. Over decades, many methods have been proposed for facial landmark detection including the shape fitting, the coordinate regression such as ASMNet and AnchorFace. However, their performance is still limited for real-time applications in terms of both accuracy and efficiency. In this paper, we propose a novel method called SHELF which is the first to combine the shape fitting and heatmap regression approaches for landmark detection in human face. The heatmap model aims to generate the landmarks that fit to the common shapes. The method has been evaluated on three datasets 300W-Challenging, WFLW, 300VW-E with 31557 images and achieved a normalized mean error (NME) of 6.67% , 7.34%, 12.55% correspondingly, which overcomes most existing methods. For the first two datasets, the method is also comparable to the state of the art AnchorFace with a NME of 6.19%, 4.62%, respectively.
Copyright © 2023 N. T. N. Quyen et al., licensed to EAI. This is an open access article distributed under the terms of the CC BY-NC-SA 4.0, which permits copying, redistributing, remixing, transformation, and building upon the material in any medium so long as the original work is properly cited.