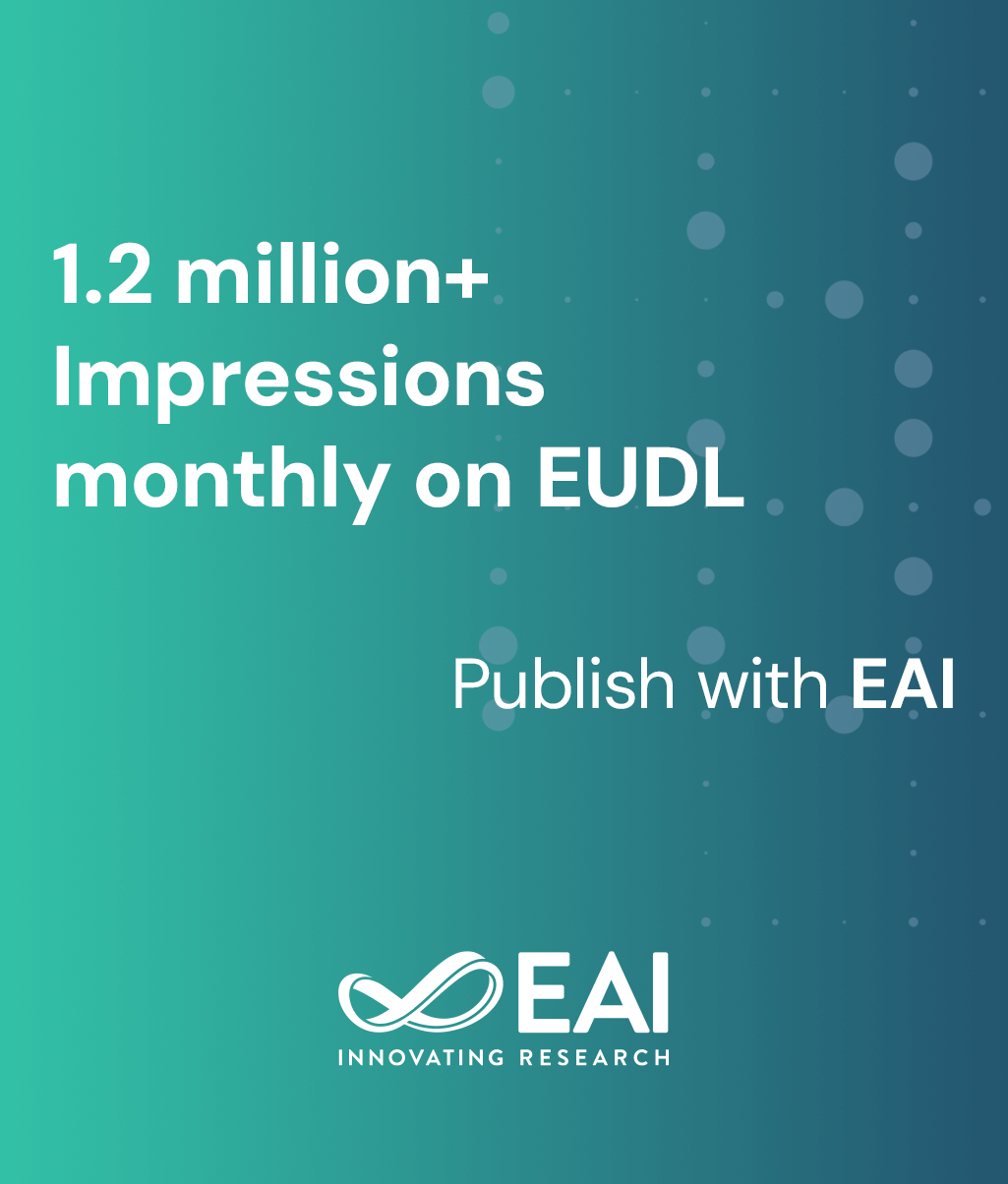
Research Article
Transforming Data with Ontology and Word Embedding for an Efficient Classification Framework
@ARTICLE{10.4108/eetinis.v10i2.2726, author={Thi Thanh Sang Nguyen and Pham Minh Thu Do and Thanh Tuan Nguyen and Thanh Tho Quan}, title={Transforming Data with Ontology and Word Embedding for an Efficient Classification Framework}, journal={EAI Endorsed Transactions on Industrial Networks and Intelligent Systems}, volume={10}, number={2}, publisher={EAI}, journal_a={INIS}, year={2023}, month={6}, keywords={ontology, Onto2Vec, Doc2Vec, Classification}, doi={10.4108/eetinis.v10i2.2726} }
- Thi Thanh Sang Nguyen
Pham Minh Thu Do
Thanh Tuan Nguyen
Thanh Tho Quan
Year: 2023
Transforming Data with Ontology and Word Embedding for an Efficient Classification Framework
INIS
EAI
DOI: 10.4108/eetinis.v10i2.2726
Abstract
Transforming data into appropriate formats is crucial because it can speed up the training process and enhance the performance of classification algorithms. It is, however, challenging due to the complicated process, resource-intensive and preserved meaning of the data. This study proposes new approaches to building knowledge representation models using word-embedding and ontology techniques, which can transform text data into digital data and still keep semantic/context information of themselves in order to enhance modeling data later. To evaluate the effectiveness of the built models, a classification framework is proposed and performed on a public real dataset. Experimental results show that the constructed knowledge representation models contribute significantly to the performance of classification methods.
Copyright © 2023 Thi Thanh Sang Nguyen et al., licensed to EAI. This is an open access article distributed under the terms of the CC BY-NC-SA 4.0, which permits copying, redistributing, remixing, transformation, and building upon the material in any medium so long as the original work is properly cited.