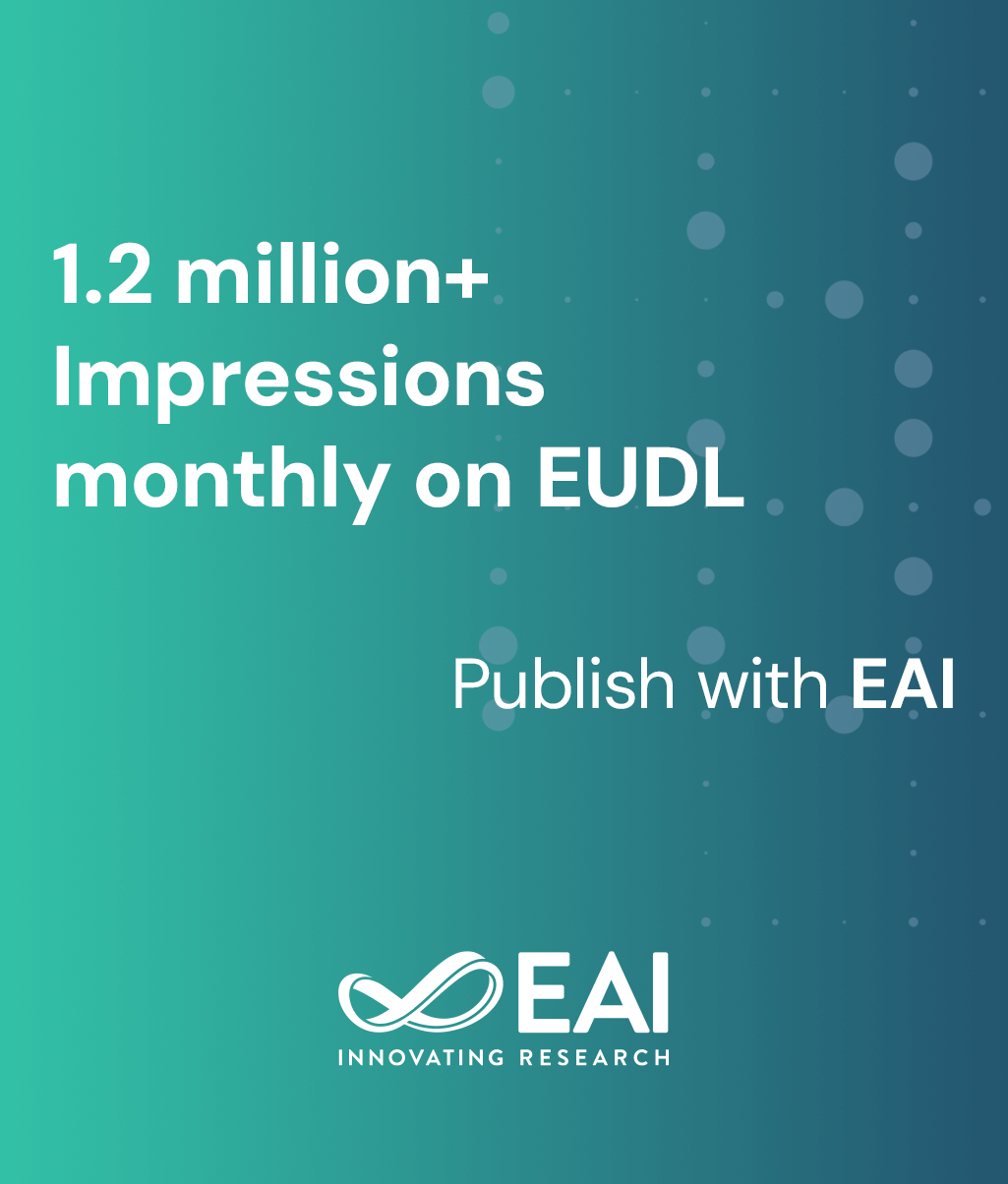
Research Article
Model Protection Scheme Against Distillation Attack in Internet of Vehicles
@ARTICLE{10.4108/eetel.v8i3.3318, author={Weiping Peng and Jiabao Liu and Yuan Ping and Di Ma}, title={Model Protection Scheme Against Distillation Attack in Internet of Vehicles}, journal={EAI Endorsed Transactions on e-Learning}, volume={8}, number={3}, publisher={EAI}, journal_a={EL}, year={2023}, month={6}, keywords={Internet of vehicles, Privacy protection, Distillation immunity, Model reinforcement, Differential privacy}, doi={10.4108/eetel.v8i3.3318} }
- Weiping Peng
Jiabao Liu
Yuan Ping
Di Ma
Year: 2023
Model Protection Scheme Against Distillation Attack in Internet of Vehicles
EL
EAI
DOI: 10.4108/eetel.v8i3.3318
Abstract
Aiming at the problems of model security and user data disclosure caused by the deep learning model in the Internet of Vehicles scenario, which can be stolen by malicious roadside units or base stations and other attackers through knowledge distillation and other techniques, this paper proposes a scheme to strengthen prevent against distillation. The scheme exploits the idea of model reinforcement such as model self-learning and attention mechanism to maximize the difference between the pre-trained model and the normal model without sacrificing performance. It also combines local differential privacy technology to reduce the effectiveness of model inversion attacks. Our experimental results on several datasets show that this method is effective for both standard and data-free knowledge distillation, and provides better model protection than passive defense.
Copyright © 2022 Weiping Peng et al., licensed to EAI. This is an open access article distributed under the terms of the CC BY-NCSA 4.0, which permits copying, redistributing, remixing, transformation, and building upon the material in any medium so long as the original work is properly cited.