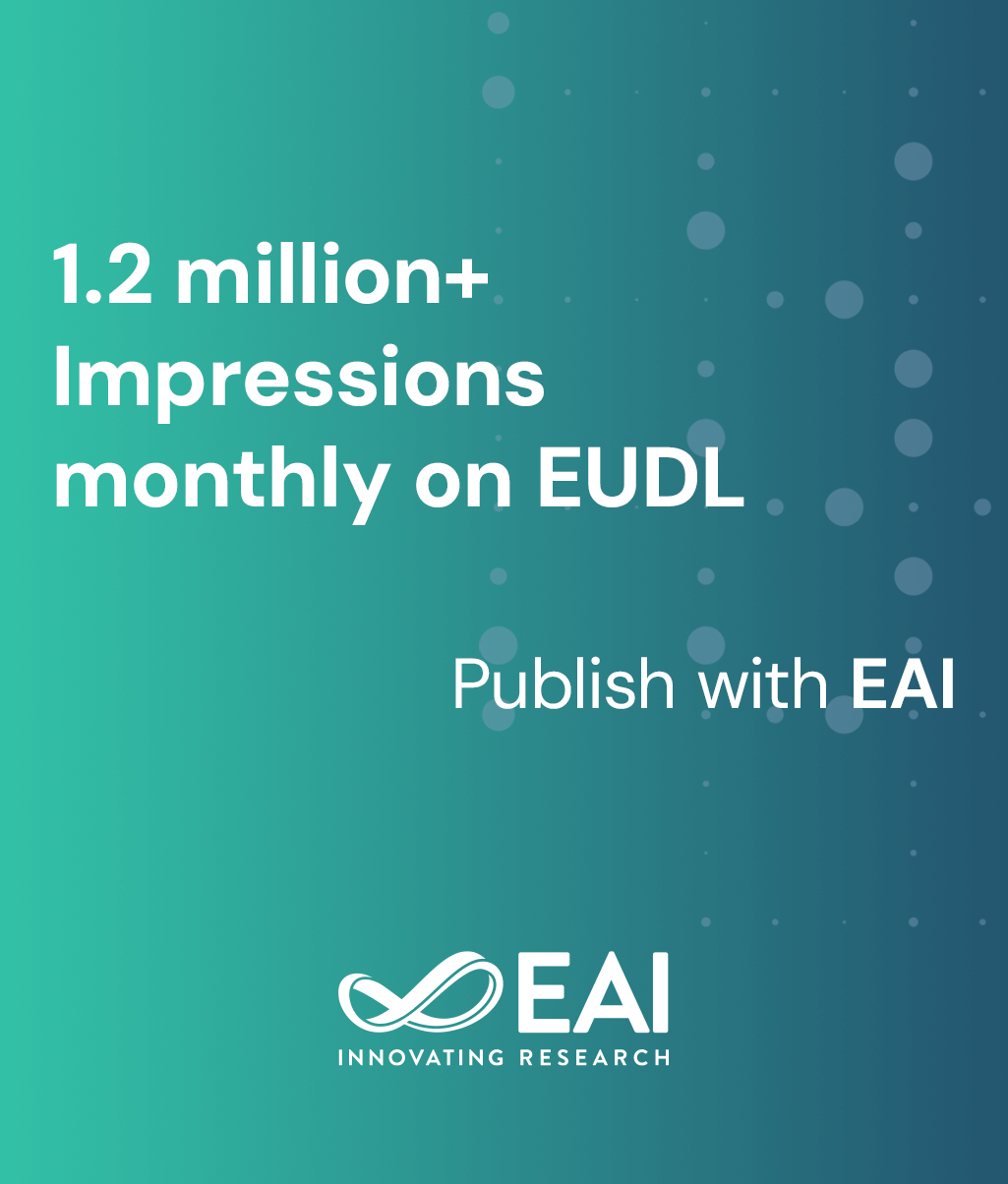
Research Article
Covid-19 Diagnosis by Gray-level Cooccurrence Matrix and Genetic Algorithm
@ARTICLE{10.4108/eetel.v8i1.2344, author={Xiaoyan Jiang and Mackenzie Brown and Zuojin Hu and Hei-Ran Cheong}, title={Covid-19 Diagnosis by Gray-level Cooccurrence Matrix and Genetic Algorithm}, journal={EAI Endorsed Transactions on e-Learning}, volume={8}, number={1}, publisher={EAI}, journal_a={EL}, year={2022}, month={8}, keywords={Gray-level Cooccurrence Matrix, Genetic Algorithm, optimization, Feedforward Neural Network, K-fold cross-validation, COVID-19, Diagnosis}, doi={10.4108/eetel.v8i1.2344} }
- Xiaoyan Jiang
Mackenzie Brown
Zuojin Hu
Hei-Ran Cheong
Year: 2022
Covid-19 Diagnosis by Gray-level Cooccurrence Matrix and Genetic Algorithm
EL
EAI
DOI: 10.4108/eetel.v8i1.2344
Abstract
Currently, improving the identification of COVID-19 with the help of computer vision and artificial intelligence has received great attention from researchers. This paper proposes a novel method for automatic detection of COVID-19 based on chest CT to help radiologists improve the speed and reliability of tests for diagnosing COVID-19. Our algorithm is a hybrid approach based on the Gray-level Cooccurrence Matrix and Genetic Algorithm. The Gray-level Cooccurrence Matrix (GLCM) was used to extract CT scan image features, GA algorithm was used as an optimizer, and a feedforward neural network was used as a classifier. Finally, we use 296 chest CT scan images to evaluate the detection performance of our proposed method. To more accurately evaluate the accuracy of the algorithm, 10-run 10-fold cross-validation was introduced. Experimental results show that our proposed method outperforms state-of-the-art methods in terms of Sensitivity, Accuracy, F1, MCC, and FMI.
Copyright © 2022 Xiaoyan Jiang et al., licensed to EAI. This is an open access article distributed under the terms of the Creative Commons Attribution license, which permits unlimited use, distribution and reproduction in any medium so long as the original work is properly cited.