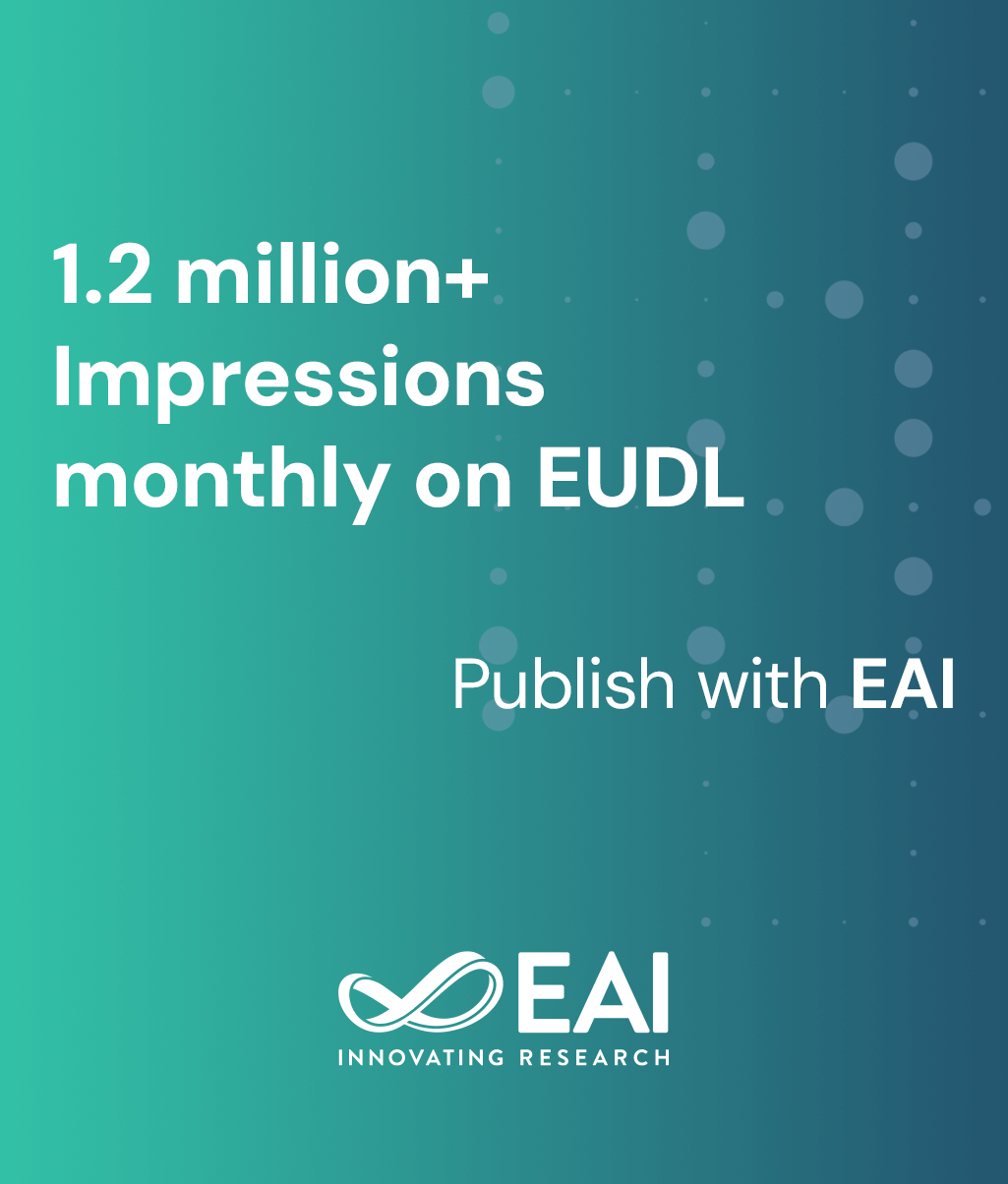
Research Article
A Review of Real-Time Semantic Segmentation Methods for 2D Data in the Context of Deep Learning
@ARTICLE{10.4108/eetel.8433, author={Meng Gao and Haifeng Sima}, title={A Review of Real-Time Semantic Segmentation Methods for 2D Data in the Context of Deep Learning}, journal={EAI Endorsed Transactions on e-Learning}, volume={11}, number={1}, publisher={EAI}, journal_a={EL}, year={2025}, month={4}, keywords={Image Semantic Segmentation, Deep Learning, Fully Supervised Learning, 2D Data}, doi={10.4108/eetel.8433} }
- Meng Gao
Haifeng Sima
Year: 2025
A Review of Real-Time Semantic Segmentation Methods for 2D Data in the Context of Deep Learning
EL
EAI
DOI: 10.4108/eetel.8433
Abstract
Semantic segmentation is a key research topic in the field of computer vision, aiming to assign each pixel to the corresponding category based on the semantic information in the image. This technology has significant application value in fields such as virtual reality and autonomous driving.With the rapid development of deep learning, particularly with the advent of FCN, image semantic segmentation has made substantial progress. Fully supervised learning, which trains deep learning models using labeled data, has demonstrated excellent performance in semantic segmentation tasks. This paper provides a comprehensive discussion and analysis of fully supervised semantic segmentation algorithms for 2D data in deep learning. First, it introduces the concept of semantic segmentation, its development, and its application scenarios. Next, it systematically reviews and categorizes current real-time semantic segmentation algorithms, analyzing the characteristics and limitations of each. Additionally, this paper presents a complete evaluation framework for real-time semantic segmentation, including relevant datasets and evaluation metrics. Based on this foundation, it identifies several challenges currently facing the field and suggests potential directions for future research. Through this summary and analysis, the paper aims to provide valuable insights for researchers conducting studies on image semantic segmentation.
Copyright © 2025 M. Gao, H. Sima, licensed to EAI. This is an open access article distributed under the terms of the CC BY-NC-SA 4.0, which permits copying, redistributing, remixing, transformation, and building upon the material in any medium so long as the original work is properly cited.