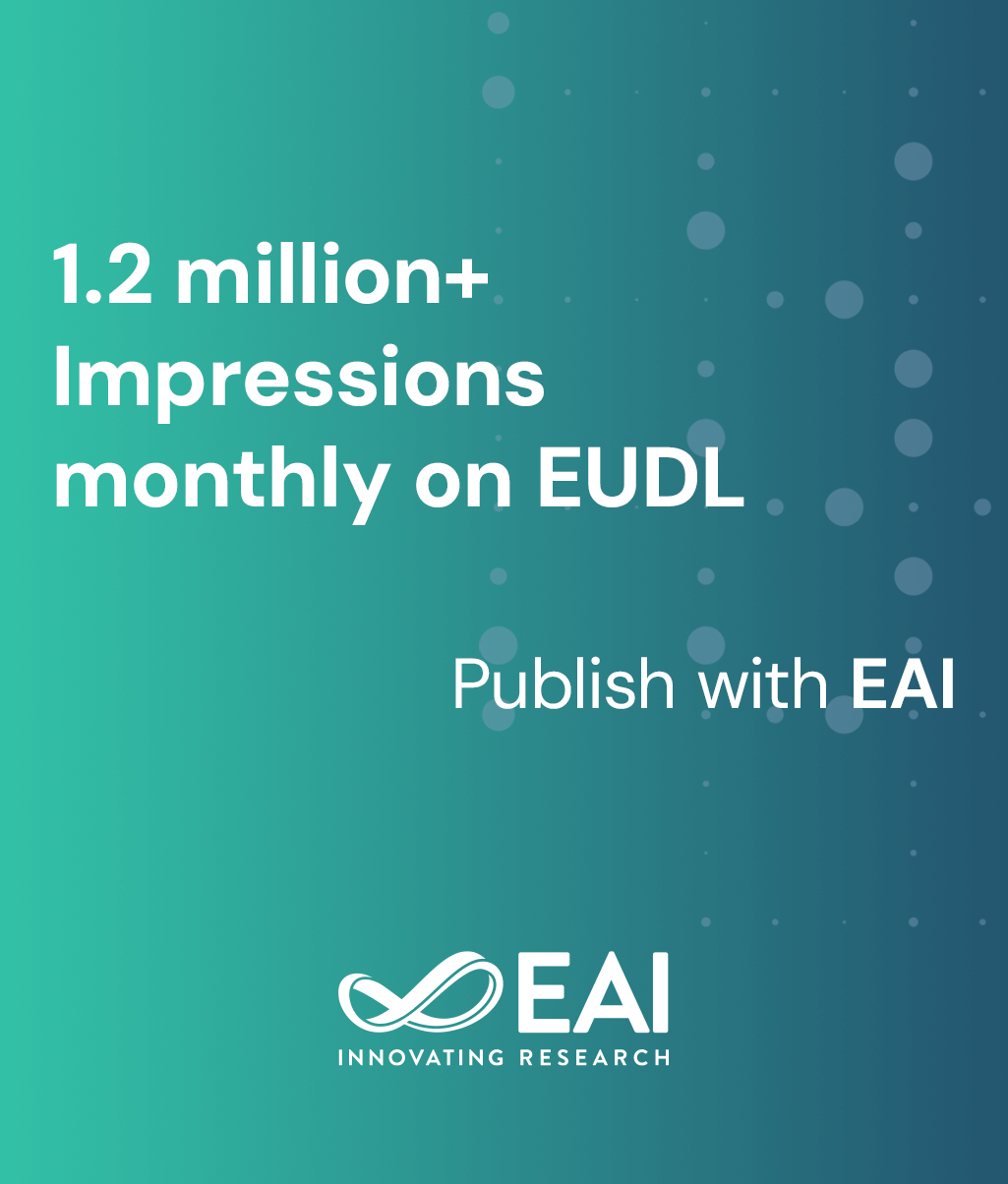
Research Article
A review of research and development of semi-supervised learning strategies for medical image processing
@ARTICLE{10.4108/eetel.4822, author={Shengke Yang}, title={A review of research and development of semi-supervised learning strategies for medical image processing}, journal={EAI Endorsed Transactions on e-Learning}, volume={9}, number={1}, publisher={EAI}, journal_a={EL}, year={2024}, month={1}, keywords={Deep Learning, semi-supervised learning, Medical Image Processing, neural network}, doi={10.4108/eetel.4822} }
- Shengke Yang
Year: 2024
A review of research and development of semi-supervised learning strategies for medical image processing
EL
EAI
DOI: 10.4108/eetel.4822
Abstract
Accurate and robust segmentation of organs or lesions from medical images plays a vital role in many clinical applications such as diagnosis and treatment planning. With the massive increase in labeled data, deep learning has achieved great success in image segmentation. However, for medical images, the acquisition of labeled data is usually expensive because generating accurate annotations requires expertise and time, especially in 3D images. To reduce the cost of labeling, many approaches have been proposed in recent years to develop a high-performance medical image segmentation model to reduce the labeling data. For example, combining user interaction with deep neural networks to interactively perform image segmentation can reduce the labeling effort. Self-supervised learning methods utilize unlabeled data to train the model in a supervised manner, learn the basics and then perform knowledge transfer. Semi-supervised learning frameworks learn directly from a limited amount of labeled data and a large amount of unlabeled data to get high quality segmentation results. Weakly supervised learning approaches learn image segmentation from borders, graffiti, or image-level labels instead of using pixel-level labeling, which reduces the burden of labeling. However, the performance of weakly supervised learning and self-supervised learning is still limited on medical image segmentation tasks, especially on 3D medical images. In addition to this, a small amount of labeled data and a large amount of unlabeled data are more in line with actual clinical scenarios. Therefore, semi-supervised learning strategies become very important in the field of medical image processing.
Copyright © 2024 S. Yang et al., licensed to EAI. This is an open access article distributed under the terms of the CC BY-NC-SA 4.0, which permits copying, redistributing, remixing, transformation, and building upon the material in any medium so long as the original work is properly cited.