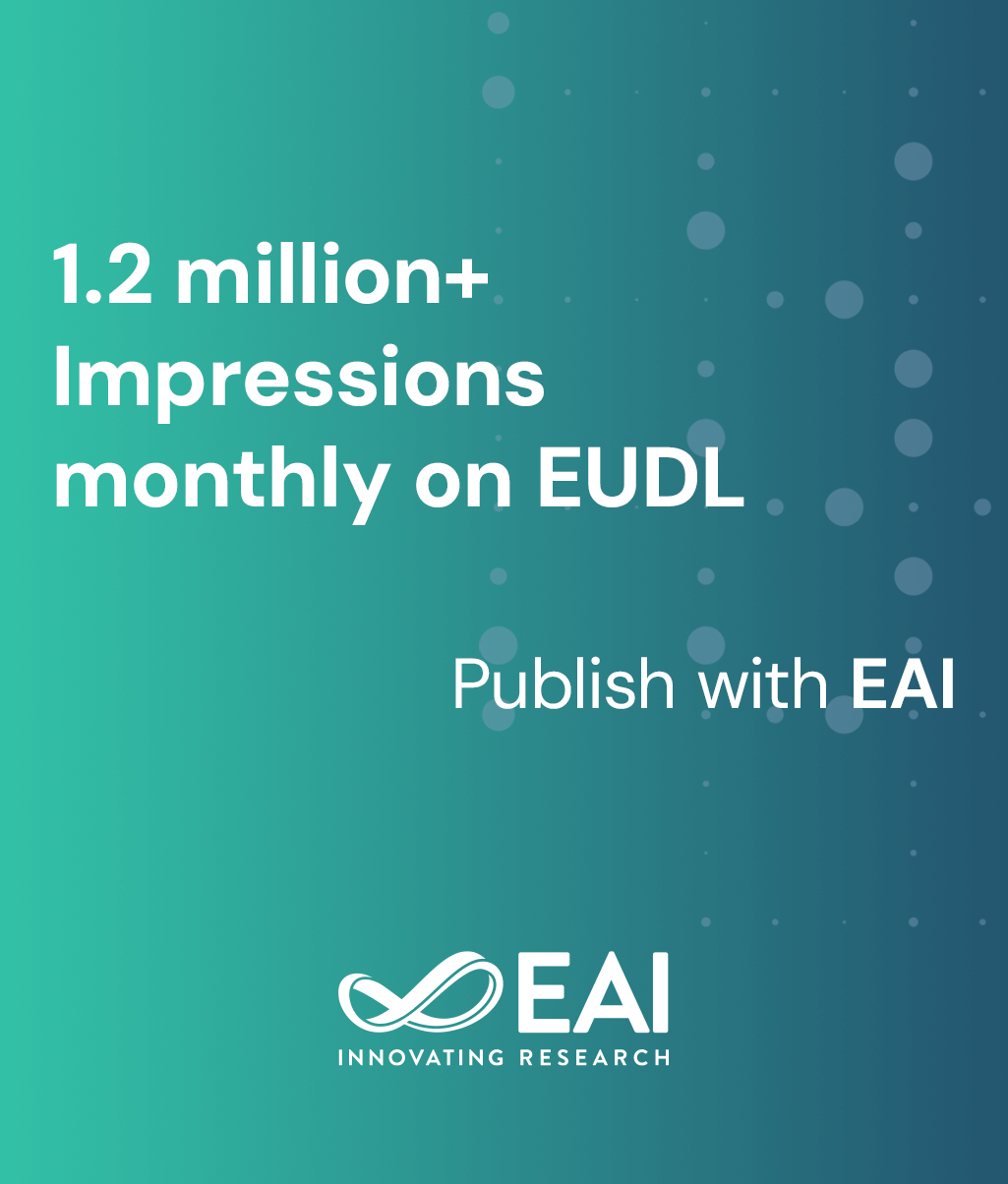
Research Article
Deep learning-based lung nodule detection: a review
@ARTICLE{10.4108/eetel.4775, author={Feifei Zhou}, title={Deep learning-based lung nodule detection: a review}, journal={EAI Endorsed Transactions on e-Learning}, volume={9}, number={1}, publisher={EAI}, journal_a={EL}, year={2024}, month={2}, keywords={}, doi={10.4108/eetel.4775} }
- Feifei Zhou
Year: 2024
Deep learning-based lung nodule detection: a review
EL
EAI
DOI: 10.4108/eetel.4775
Abstract
CT scan acquisition is fast and cost-effective and has become the main lung imaging tool. However, the increase in large numbers of CT scans has placed a heavy burden on radiologists; therefore, automated lung nodule detection techniques are needed to reduce the workload of radiologists and computer-aided detection systems are proposed for further accurate diagnosis of the condition. This review provides a comprehensive overview of recent automated lung nodule detection techniques and challenges, etc., as well as a detailed overview and discussion of current research gaps, future developments, and research trends. Relevant articles published in databases such as IEEE Xplore, Science Direct, PubMed, and Web of Science cover research algorithms published from 2014 to 2023, mainly discussing deep learning-based techniques. The schemes presented in these articles, the databases used, the experimental results, and the performance of the algorithms are compared and discussed. This work aims to introduce researchers and readers to the latest techniques and their advances in the detection of lung nodules in the last decade, which will help researchers and radiologists to further understand the latest techniques in this field.
Copyright © 2024 F. Zhou et al., licensed to EAI. This is an open access article distributed under the terms of the CC BY-NC-SA 4.0, which permits copying, redistributing, remixing, transformation, and building upon the material in any medium so long as the original work is properly cited.