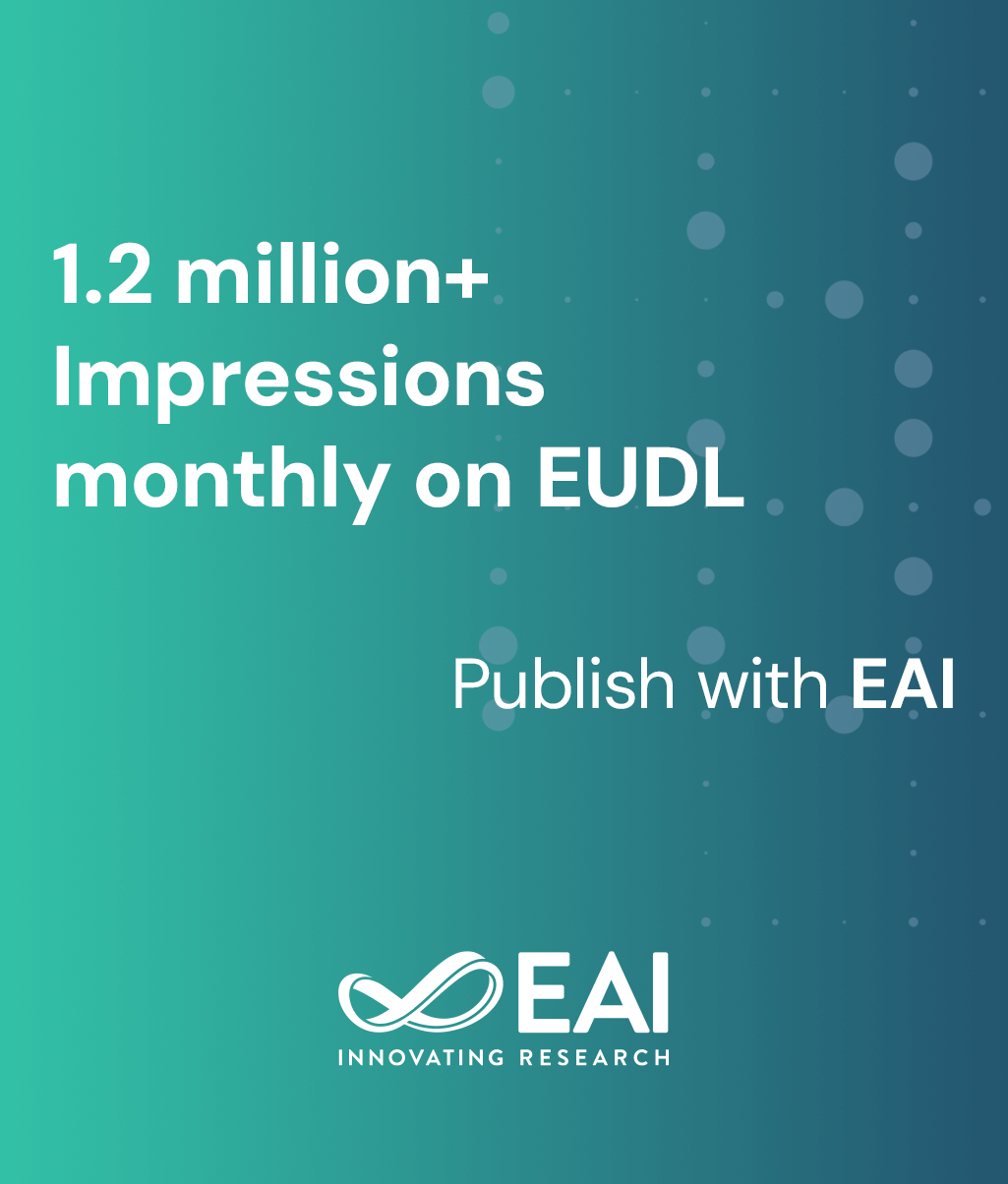
Research Article
Review of Graph Neural Networks for Medical Image
@ARTICLE{10.4108/eetel.4358, author={Jing Wang}, title={Review of Graph Neural Networks for Medical Image}, journal={EAI Endorsed Transactions on e-Learning}, volume={9}, number={1}, publisher={EAI}, journal_a={EL}, year={2023}, month={12}, keywords={graph neural networks, deep learning, graph data, medical images, denoising}, doi={10.4108/eetel.4358} }
- Jing Wang
Year: 2023
Review of Graph Neural Networks for Medical Image
EL
EAI
DOI: 10.4108/eetel.4358
Abstract
As deep learning continues to evolve, more and more applications are generating data from non-Euclidean domains and representing them as graphs with complex relationships and interdependencies between objects. This poses a significant challenge to deep learning algorithms. Because, due to the uniqueness of graphs, applying deep learning to ubiquitous graph data is not an easy task. To solve the problem in non-Euclidean domains, graph Neural Networks (GNNs) have emerged. A graph neural network (GNN) is a neural model that captures dependencies between graphs by passing messages between graph nodes. With the continuous development of medical image technology, medical image diagnosis plays a crucial role in clinical practice. However, in practice, medical images are often affected by noise, artifacts, and other interfering factors, which may lead to inaccurate and unstable diagnostic results. Therefore, image-denoising techniques become especially critical in medical image processing. Therefore, researchers have proposed innovative methods based on graph neural networks for effective noise removal, preserving the key features of the image and improving the quality and usability of medical images. This paper reviews the research progress of graph neural networks in the field of medical image denoising. It also summarises the problems and challenges of current research and looks at the future direction of medical image-denoising research.
Copyright © 2023 J. Wang et al., licensed to EAI. This is an open access article distributed under the terms of the CC BY-NC-SA 4.0, which permits copying, redistributing, remixing, transformation, and building upon the material in any medium so long as the original work is properly cited.