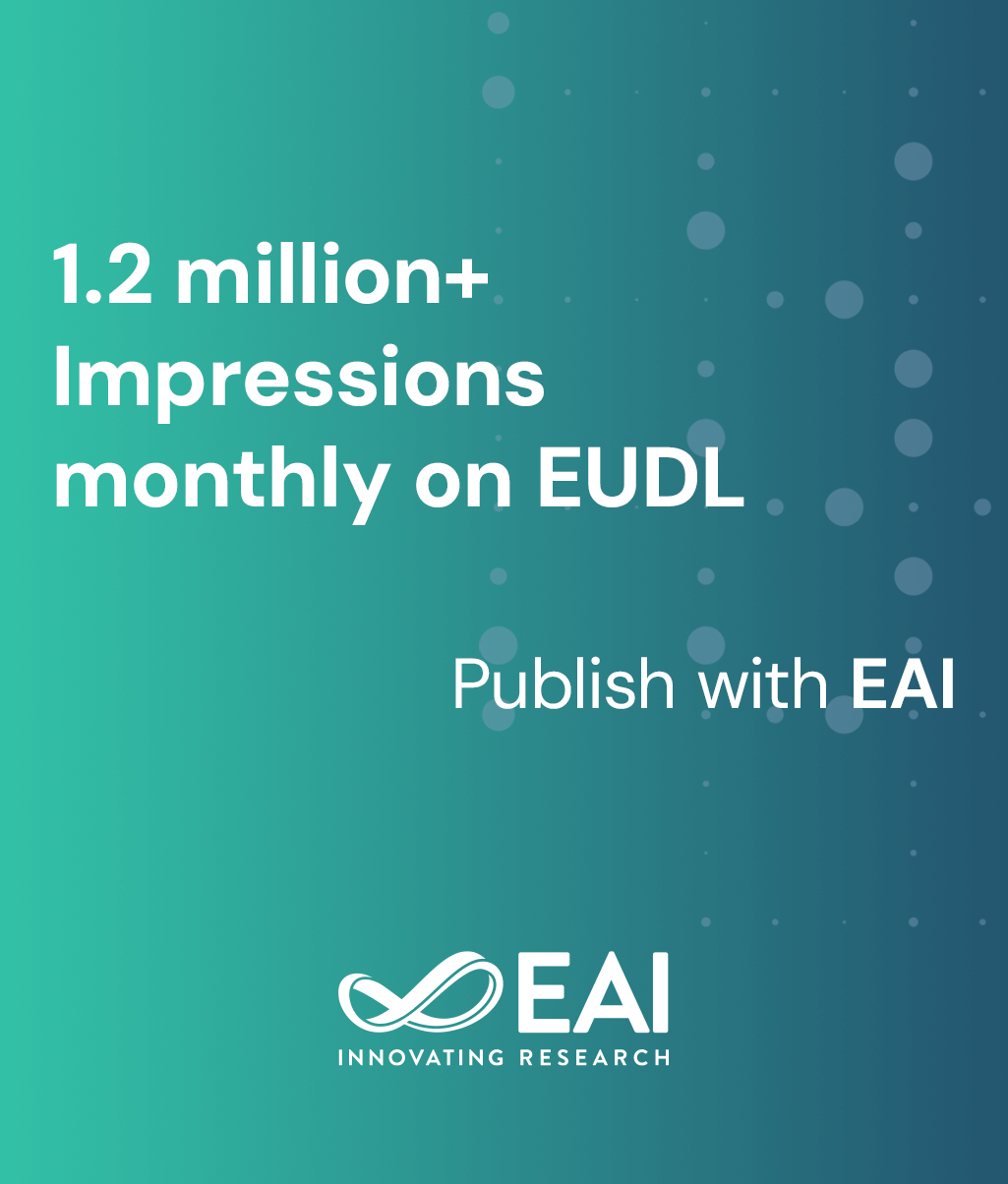
Research Article
Human Emotion Recognition with an Advanced Vision Transformer Model
@ARTICLE{10.4108/eetcasa.8101, author={Kha Tu Huynh and Vo Nhat Anh Nguyen and Tan Duy Le and Thuong Le-Tien}, title={Human Emotion Recognition with an Advanced Vision Transformer Model}, journal={EAI Endorsed Transactions on Contex-aware Systems and Applications}, volume={10}, number={1}, publisher={EAI}, journal_a={CASA}, year={2025}, month={4}, keywords={facial expression, facial emotion detection, face recognition, Vision Transformer, ViT, EffectiveViT-M5}, doi={10.4108/eetcasa.8101} }
- Kha Tu Huynh
Vo Nhat Anh Nguyen
Tan Duy Le
Thuong Le-Tien
Year: 2025
Human Emotion Recognition with an Advanced Vision Transformer Model
CASA
EAI
DOI: 10.4108/eetcasa.8101
Abstract
This paper proposes a novel deep-learning technique that leverages the Efficient Vision Transformer –M5 (Efficient ViT-M5) model to improve the existing design by offering a more computationally economical version that maintains good performance, making it highly suitable for practical applica-tions. The utilization of transfer learning involved leveraging pre-trained weights from the ImageNet dataset, substantially enhancing the model's accu-racy and efficiency. The proposed method involves training the advanced Effi-cientViTM5 model utilizing three widely recognized facial emotion recognition datasets: FER2013+, AffectNet, and RAF-DB. A comprehensive data augmentation pipeline is employed to enhance the diversity of the training data and bolster the model's robustness. The trained proposed model proved exceptional accuracy rates of 94.28% (FER2013+), 94.69% (AffectNet), and 97.76% (RAF-DB). The results emphasize the strength and effectiveness of the proposed model in identifying face emotions in various datasets, showcasing its potential for practical use in emotion-aware computing, security, and health diagnostics. The research significantly improves facial emotion recognition by introducing a reliable and practical way of recognizing emotions using cutting-edge deep learning techniques. The results show the possibility of enhancing and flexible interactions between humans and computers, highlighting the efficacy of sophisticated deep learning models in addressing complex computer vision problems.
Copyright © 2025 K. T. Huynh et al., licensed to EAI. This is an open access article distributed under the terms of the CC BY-NCSA 4.0, which permits copying, redistributing, remixing, transformation, and building upon the material in any medium so long as the original work is properly cited.