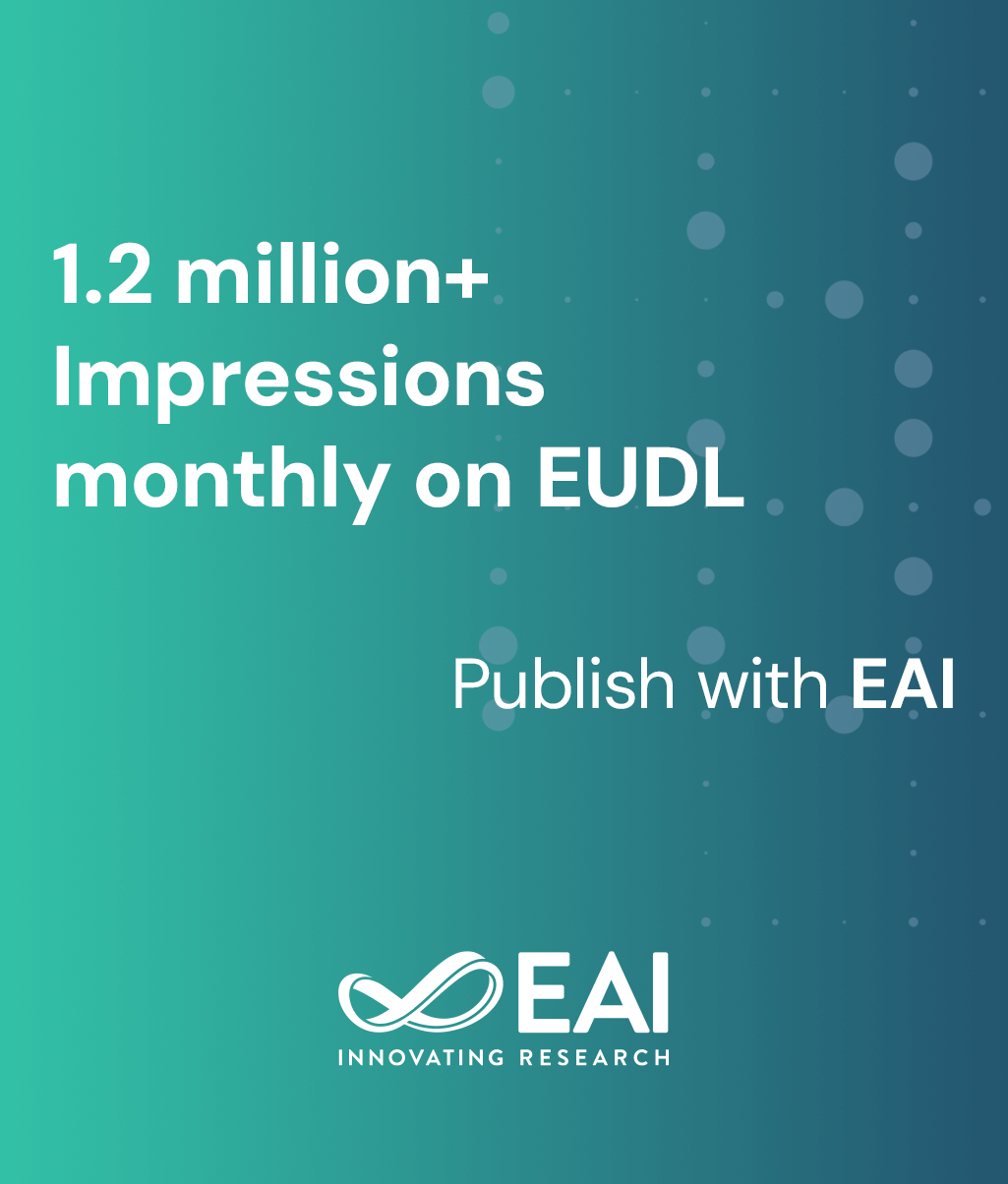
Research Article
Achievement Performance Prediction Model Based on Deep Neural Network of Student Similarity
@INPROCEEDINGS{10.4108/eai.8-9-2023.2340180, author={Yixuan Rong and Tingnian He and Zhuoran Li and Guoqi Liu}, title={Achievement Performance Prediction Model Based on Deep Neural Network of Student Similarity}, proceedings={Proceedings of the 4th International Conference on Modern Education and Information Management, ICMEIM 2023, September 8--10, 2023, Wuhan, China}, publisher={EAI}, proceedings_a={ICMEIM}, year={2023}, month={11}, keywords={achievement prediction; deep neural networks; dropout}, doi={10.4108/eai.8-9-2023.2340180} }
- Yixuan Rong
Tingnian He
Zhuoran Li
Guoqi Liu
Year: 2023
Achievement Performance Prediction Model Based on Deep Neural Network of Student Similarity
ICMEIM
EAI
DOI: 10.4108/eai.8-9-2023.2340180
Abstract
Student performance prediction aims to predict performance through student information and guide students and teachers to optimise the learning process. Traditional methodological studies focus more on students' own influencing factors and ignore the similarity of different students' learning abilities. To address this problem, the performance prediction model based on Deep Neural Network of student similarity (Sim-DNN) takes into account student-to-student associations, and predicts students' academic performance by calculating the similarity of different students' attribute features, selecting students with higher similarity to the target students, and weighting and summing the historical scores of the similar students according to the degree of similarity as the input to the deep neural network . In order to minimise the effect of overfitting, the model incorporates Dropout in the DNN.The experimental results show that the model proposed in this paper has a better prediction performance on both public datasets, Mathematics and Portuguese.