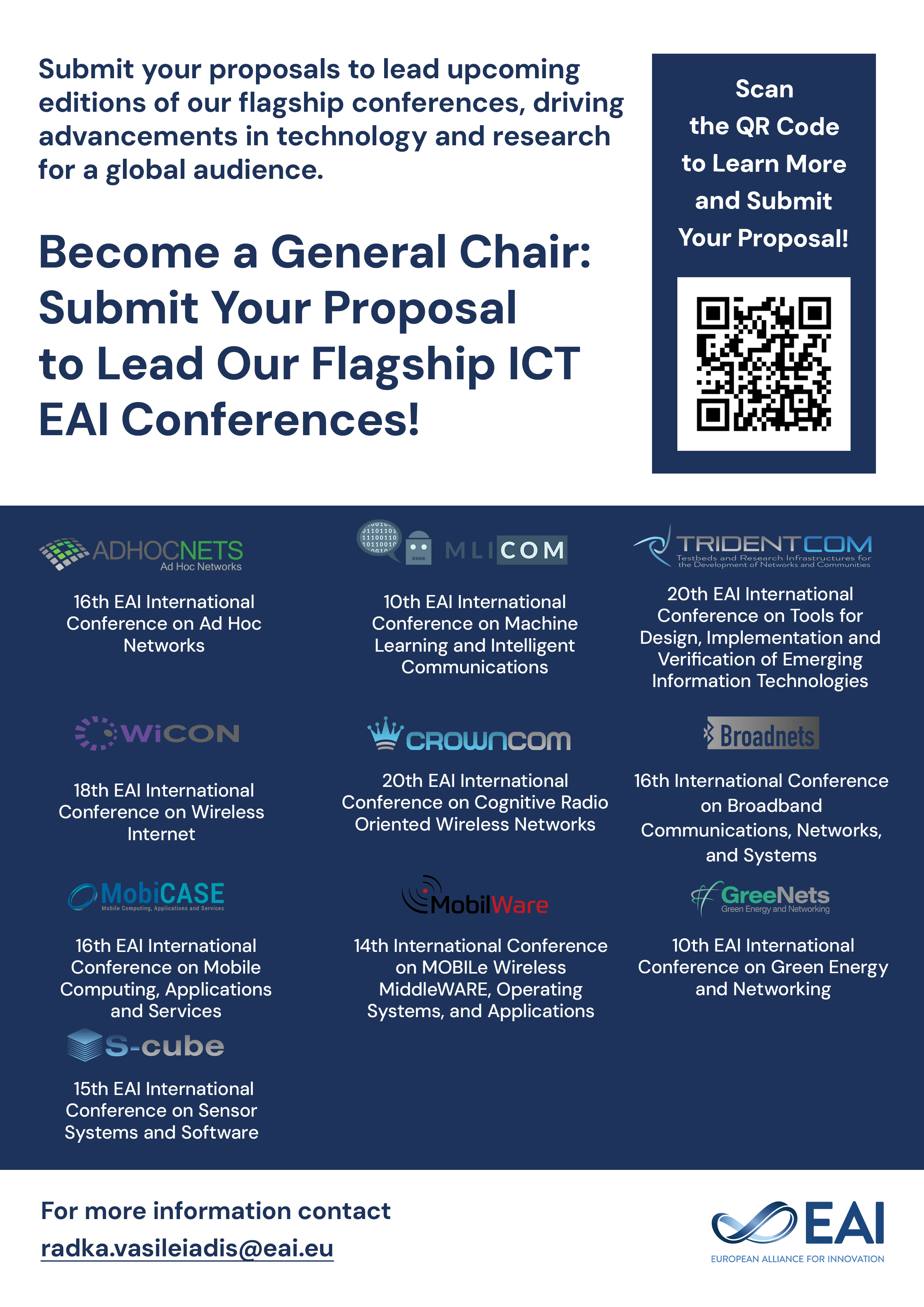
Research Article
A Novel Loss Function Considering the Distance Between Forecasting and Historical Values in Financial Time Series Forecasting Models
@INPROCEEDINGS{10.4108/eai.8-12-2023.2344823, author={Weijie Zhang and Jiaxing Li and Yujie Li}, title={A Novel Loss Function Considering the Distance Between Forecasting and Historical Values in Financial Time Series Forecasting Models}, proceedings={Proceedings of the 5th Management Science Informatization and Economic Innovation Development Conference, MSIEID 2023, December 8--10, 2023, Guangzhou, China}, publisher={EAI}, proceedings_a={MSIEID}, year={2024}, month={4}, keywords={stock index forecasting; long short-term memory; loss function optimization;}, doi={10.4108/eai.8-12-2023.2344823} }
- Weijie Zhang
Jiaxing Li
Yujie Li
Year: 2024
A Novel Loss Function Considering the Distance Between Forecasting and Historical Values in Financial Time Series Forecasting Models
MSIEID
EAI
DOI: 10.4108/eai.8-12-2023.2344823
Abstract
As big data advances by leaps and bounds, a considerable amount of extremely valuable time series data has been accumulated in finance and industry, from which the regularity can be excavated and the future trend can be forecasted. Time series forecasting has obtained widespread application in numerous fields, particularly in financial contexts, and some deep learning methods, including RNN and LSTM, has showed better performance than traditional methods, such as ARIMA, in financial time series forecasting, yet the problem of translation lag in deep learning forecasting still exists. The models may do pseudo learning. In other words, they use the values of the most current historical data directly as the forecasting values. To resolve the issue, this paper designs a new loss function which considers the distance between forecasting and historical values and conducts an experiment on three stock index time series datasets with carrier of the LSTM model. The experimental results reveal that, compared with the traditional MSE loss function, the proposed loss function has higher forecasting accuracy. This research alleviates the translation lag problem triggered by pseudo learning and thus provides a new method for reducing the error of stock index time series forecasting.