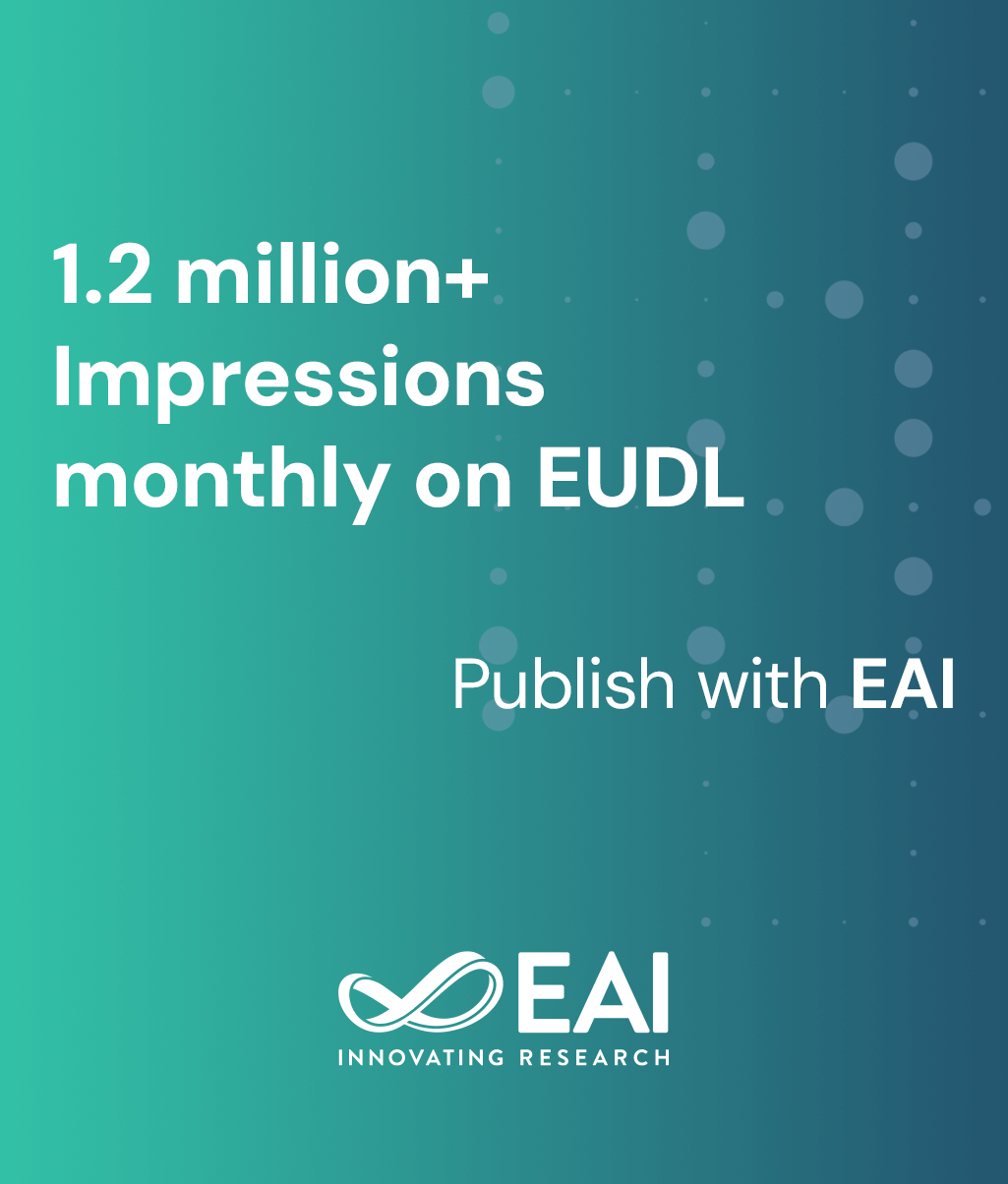
Research Article
Predicting Future Aircraft Emissions in Indonesia: A Comparative Analysis using LSTM and GRU Methods
@INPROCEEDINGS{10.4108/eai.8-11-2023.2345860, author={Fhilipus Mahendra and Reici Ratasya Putri S and Sheyla Hannim and Mufti Arifin}, title={Predicting Future Aircraft Emissions in Indonesia: A Comparative Analysis using LSTM and GRU Methods}, proceedings={Proceedings of the 2nd International Conference on Aviation Industry, Education, and Regulation, AVINER 2023, 8-9 November 2023, Jakarta, Indonesia}, publisher={EAI}, proceedings_a={AVINER}, year={2024}, month={5}, keywords={aircraft emissions long-short term memory (lstm) gate recurrent unit (gru)}, doi={10.4108/eai.8-11-2023.2345860} }
- Fhilipus Mahendra
Reici Ratasya Putri S
Sheyla Hannim
Mufti Arifin
Year: 2024
Predicting Future Aircraft Emissions in Indonesia: A Comparative Analysis using LSTM and GRU Methods
AVINER
EAI
DOI: 10.4108/eai.8-11-2023.2345860
Abstract
The aviation industry in Indonesia continues to expand resulting in concerns over the environmental impact of aircraft emissions have become paramount. This arti-cle presents a comprehensive analysis of future emission predictions using two advanced time series forecasting methods, Long Short-Term Memory (LSTM) and Gated Recurrent Unit (GRU), applied to historical aircraft emission data (Q3 2022 to Q3 2023). Leveraging the sequential nature of the data, LSTM and GRU networks are harnessed to model the intricate temporal dependencies and inherent seasonality present in the emission time series. The evaluation encompasses vari-ous performance metrics, including Mean Absolute Error (MAE), Root Mean Squared Error (RMSE), and R-squared (R²), to gauge the models' predictive ca-pabilities across different forecasting horizons. Results indicate that both LSTM and GRU methods demonstrate promising forecasting capabilities, outperforming traditional time series models. However, subtle distinctions emerge in their predic-tive efficiency. LSTM exhibits superior performance in capturing long-term de-pendencies and handling complex emission patterns, whereas GRU showcases efficiency in shorter forecasting horizons. Remarkably, the research uncovers the profound impact of ML, with the Long Short-Term Memory (LSTM) and Gated Recurrent Unit (GRU) methods emerging as the most potent tools with an accuracy reached up to 87%.