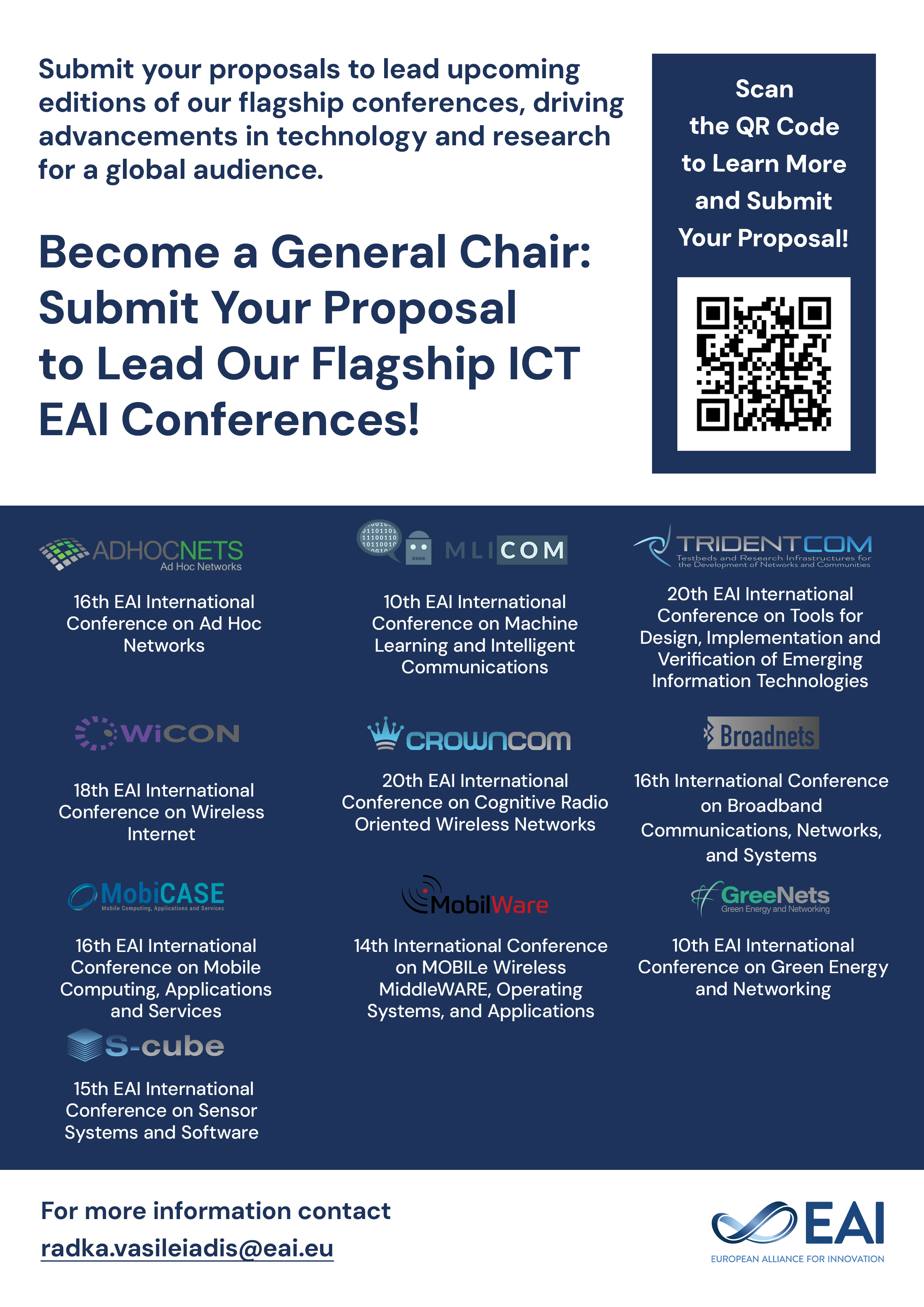
Research Article
Energy Saving by Reinforcement Learning for Multi-Chillers of HVAC Systems
@INPROCEEDINGS{10.4108/eai.7-9-2021.2315301, author={Lubna A. Hussein and Adnan A. Ateeq and Raad Z. Homod}, title={Energy Saving by Reinforcement Learning for Multi-Chillers of HVAC Systems}, proceedings={Proceedings of 2nd International Multi-Disciplinary Conference Theme: Integrated Sciences and Technologies, IMDC-IST 2021, 7-9 September 2021, Sakarya, Turkey}, publisher={EAI}, proceedings_a={IMDC-IST}, year={2022}, month={1}, keywords={multi-chillers system reinforcement learning model-based control chilled water system}, doi={10.4108/eai.7-9-2021.2315301} }
- Lubna A. Hussein
Adnan A. Ateeq
Raad Z. Homod
Year: 2022
Energy Saving by Reinforcement Learning for Multi-Chillers of HVAC Systems
IMDC-IST
EAI
DOI: 10.4108/eai.7-9-2021.2315301
Abstract
Buildings are responsible for a significant amount of energy use with the global construction sector currently consuming about 40% of the total energy production; the (HVAC) system is a broad field and is responsible for 50% of the total energy consumption. Chillers account for (30-40%) of the energy consumed in HVAC systems. In the domain of optimal control of HVAC systems, a single chiller was used for the building, and this leads to the consumption of a large amount of electrical energy. To tackle this problem, it was proposed to use multi-chillers operating alternately instead of one chiller, we use RL so that we can control the operation of refrigerants, to improve the operation of the HVAC system, and to save electrical energy in buildings. A model-based control method was used that does not take many iterations. This paper presents a method for controlling and operating a multi-chillers system: (1) Model-based control approach was used by MATLAB/SIMULINK to model a building containing two non-identical chillers depending on thermal loads. (2) ON/OFF all chillers alternately using the model reinforcement learning controller (RL-control) to select the appropriate chiller for the building conditioning process. The results were in terms of energy efficiency and performance of the enhanced learning control for the chiller, and a control unit signal (PID) was applied to make a comparison with the signals of energy, power, and temperatures.