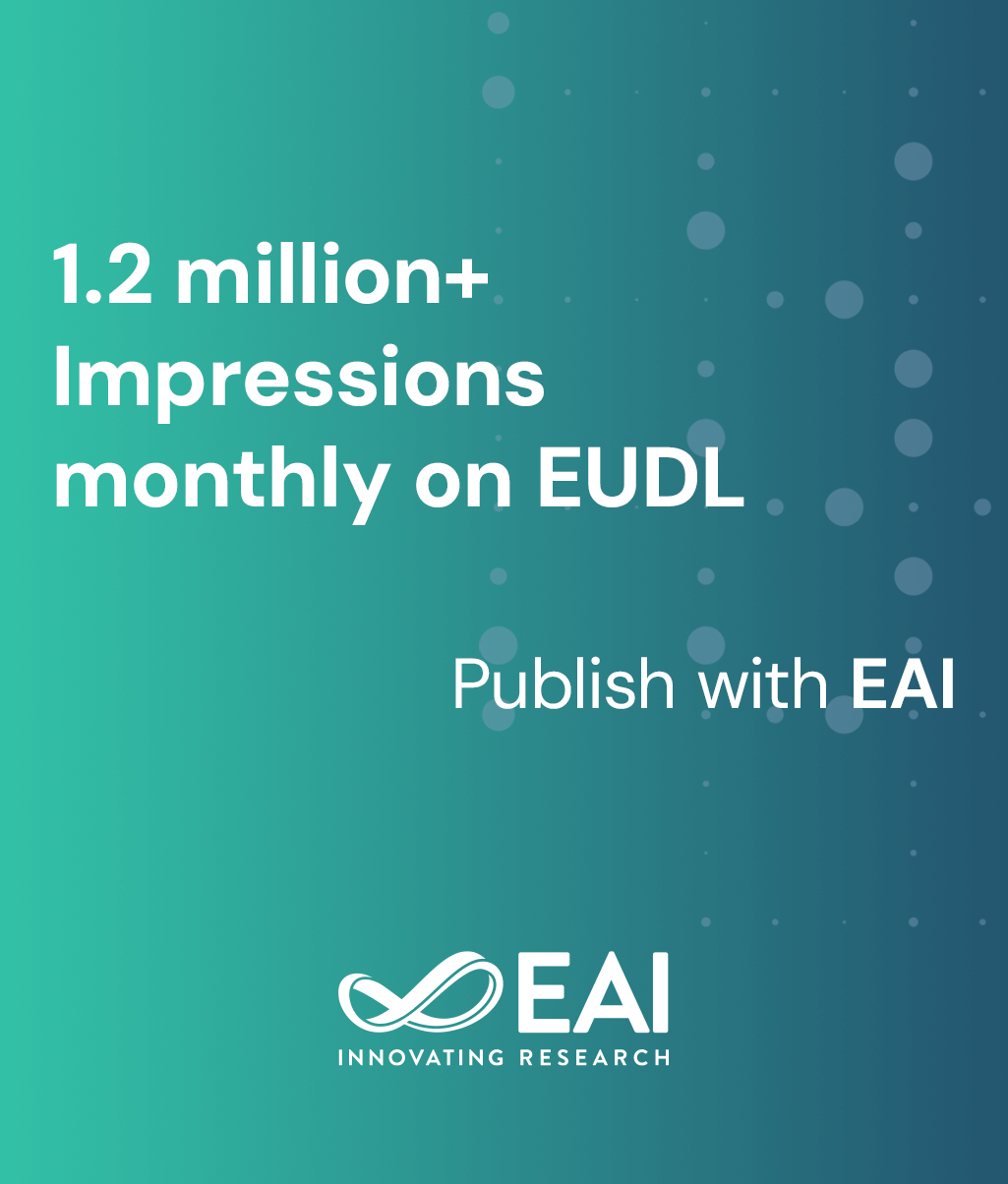
Research Article
Image Caption Generation Using CNN-LSTM Based Approach
@INPROCEEDINGS{10.4108/eai.7-12-2021.2314958, author={Bineeshia J}, title={Image Caption Generation Using CNN-LSTM Based Approach}, proceedings={Proceedings of the First International Conference on Combinatorial and Optimization, ICCAP 2021, December 7-8 2021, Chennai, India}, publisher={EAI}, proceedings_a={ICCAP}, year={2021}, month={12}, keywords={image caption long short term memory (lstm) recurrent neural network convolutional neural networks}, doi={10.4108/eai.7-12-2021.2314958} }
- Bineeshia J
Year: 2021
Image Caption Generation Using CNN-LSTM Based Approach
ICCAP
EAI
DOI: 10.4108/eai.7-12-2021.2314958
Abstract
The Picture Caption Generator automatically represents the content of an image, which is a key problem in artificial intelligence that links computer vision with natural language processing (NLP). There is a growing necessity for context-based natural language image descriptions. Recent advances in domains such as neural networks, natural language processing and computer vision, have paved the road for better description of images. It needs both computer vision approaches to interpret the content of the image and a language algorithm from the NLP sector to transform the image's interpretation into words in right order. To accomplish this, state-of-the-art algorithms like as Convolutional Neural Network (CNN) and sufficient image datasets with human-judged descriptions are used. This model combines Convolutional Neural Network (CNN) and Recurrent Neural Network (RNN). The former is employed for feature extraction of images and the latter is employed for generating sentences. The training of the model is done in such a way that it produces captions that almost define the image when the input source image is offered to the model. 6000 images have been used for training purpose and is trained over 20 epochs to finally obtain a loss value of 2.6380. The loss has been reduced exponentially through the span of 20 epochs. BLEU score metric is finally calculated to measure the model’s performance. Unigram, bigram, trigram and 4-gram precision is calculated.