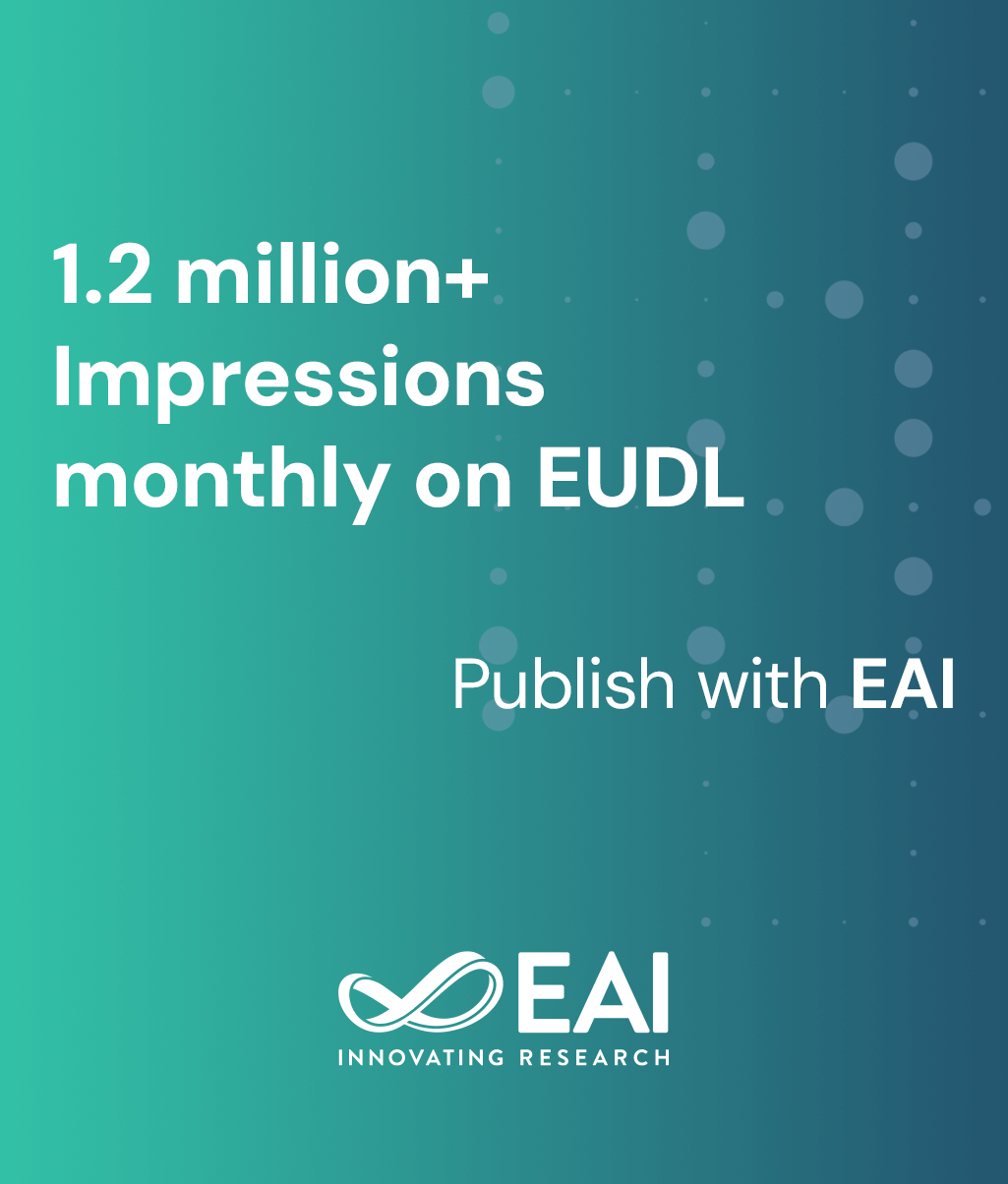
Research Article
Covid-19 Recognition by Chest CT and Deep Learning
@ARTICLE{10.4108/eai.7-1-2022.172812, author={Lin Yang and Dimas Lima}, title={Covid-19 Recognition by Chest CT and Deep Learning}, journal={EAI Endorsed Transactions on e-Learning}, volume={7}, number={23}, publisher={EAI}, journal_a={EL}, year={2022}, month={1}, keywords={Covid-19, deep learning, deep transfer learning, ResNet152V2}, doi={10.4108/eai.7-1-2022.172812} }
- Lin Yang
Dimas Lima
Year: 2022
Covid-19 Recognition by Chest CT and Deep Learning
EL
EAI
DOI: 10.4108/eai.7-1-2022.172812
Abstract
INTRODUCTION: The current RT-qPCR approach to identify Covid-19 diseases is slow and non-optimal for a large number of candidates.
OBJECTIVES: Several studies have demonstrated that deep learning can help healthcare professionals diagnose Covid-19 patients. The deep learning model proposed in this paper significantly enhanced the accuracy of identifying Covid-19 patients compared to prior approaches.
METHODS: This paper applies transfer learning and deep residual network ResNet152V2 to detect Covid-19 patients with the help of CT scan images. Monte Carlo Cross-Validation has been applied to obtain an accurate and valid result.
RESULTS: The proposed model can identify Covid-19 disease with an overall accuracy of 95.06%, along with an average precision and recall of 97.19% and 92.81%, respectively. It also obtained a specificity of 93.14% and a F1-score of 94.96%.
CONCLUSION: The performance of this proposed ResNet152V2 model is superior to most of the current Covid-19 detection models.
Copyright © 2022 Lin Yang et al., licensed to EAI. This is an open access article distributed under the terms of the Creative Commons Attribution license, which permits unlimited use, distribution and reproduction in any medium so long as the original work is properly cited.