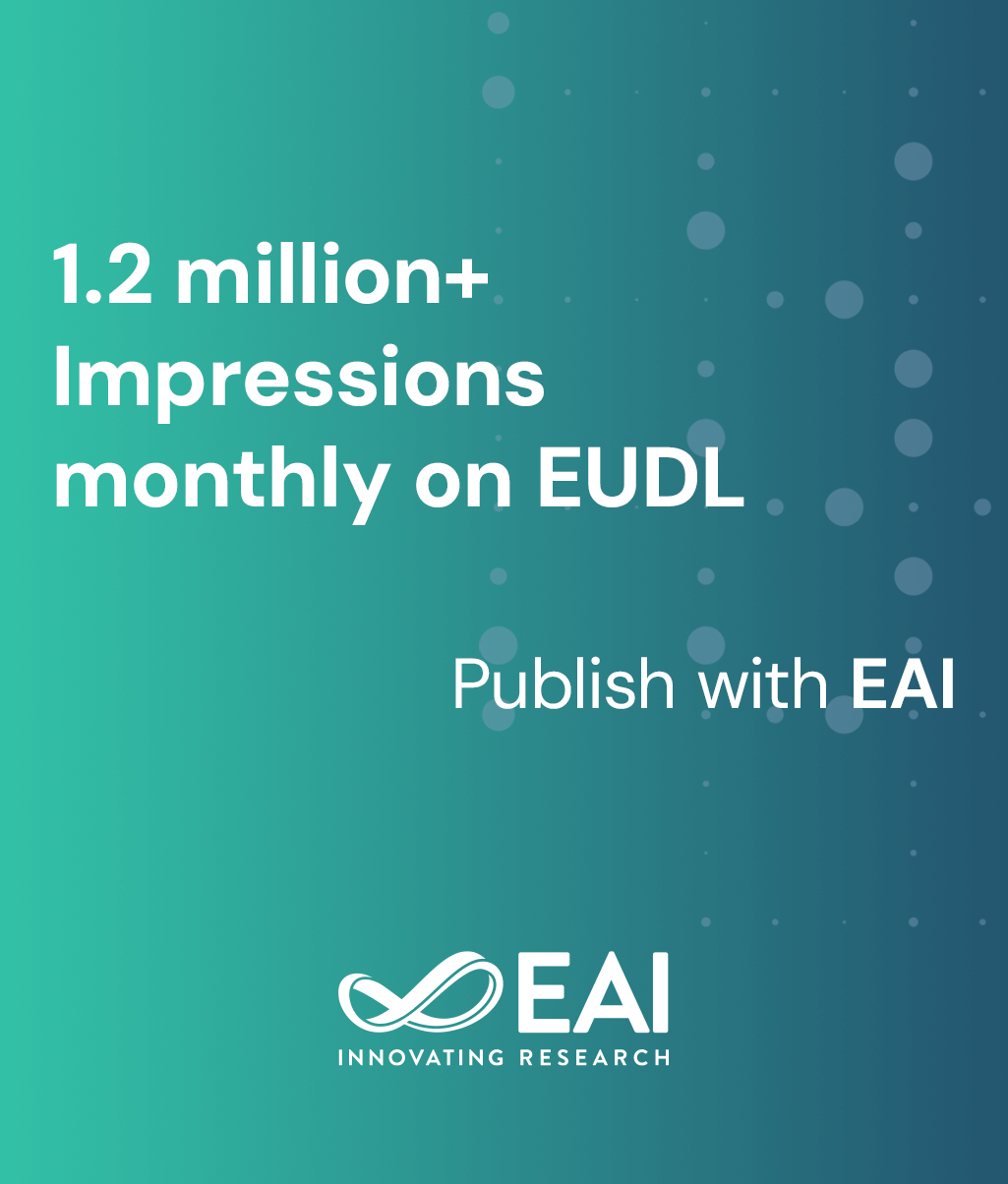
Research Article
Structural Importance-based Link Prediction Techniques in Social Network
@ARTICLE{10.4108/eai.7-1-2021.167840, author={Abdul Samad and Muhammad Azam and Mamoona Qadir}, title={Structural Importance-based Link Prediction Techniques in Social Network}, journal={EAI Endorsed Transactions on Industrial Networks and Intelligent Systems}, volume={7}, number={25}, publisher={EAI}, journal_a={INIS}, year={2021}, month={1}, keywords={Link Prediction, Social Network Analysis, Similarity Measure, Structural Importance, Centralization}, doi={10.4108/eai.7-1-2021.167840} }
- Abdul Samad
Muhammad Azam
Mamoona Qadir
Year: 2021
Structural Importance-based Link Prediction Techniques in Social Network
INIS
EAI
DOI: 10.4108/eai.7-1-2021.167840
Abstract
Link prediction in social network gaining high attention of researchers nowadays due to the rush of users towards social network. Link prediction is known as the prediction of missing or unobserved link, i.e., new interaction is going to be occurring in a near future. State-of-the-art link prediction techniques (e.g., Jaccard Index, Resource Allocation, SAM Similarity, Sorensen Index, Salton Cosine, Hub Depressed Index and Parameter-Dependent) considers only similarity of the pair of node in order to find the link. However, we argued that nodes having same status of centralization along with high similarity can connect to each other in a future. In this paper, we have proposed structural importance-based state-of-the-art link prediction techniques and compared. We have compared structural importance-based link prediction techniques with state-of-the-art techniques. The experiments are performed on four different datasets (i.e., Astro, CondMat, HepPh and HepTh). Our results show that structural importance-based link prediction techniques outperformed than state-of-the-art link prediction techniques by getting 95% at threshold 0.1 and 68% at threshold 0.7.
Copyright © 2021 A. Samad et al., licensed to EAI. This is an open access article distributed under the terms of the Creative Commons Attribution license (http://creativecommons.org/licenses/by/3.0/), which permits unlimited use, distribution and reproduction in any medium so long as the original work is properly cited.