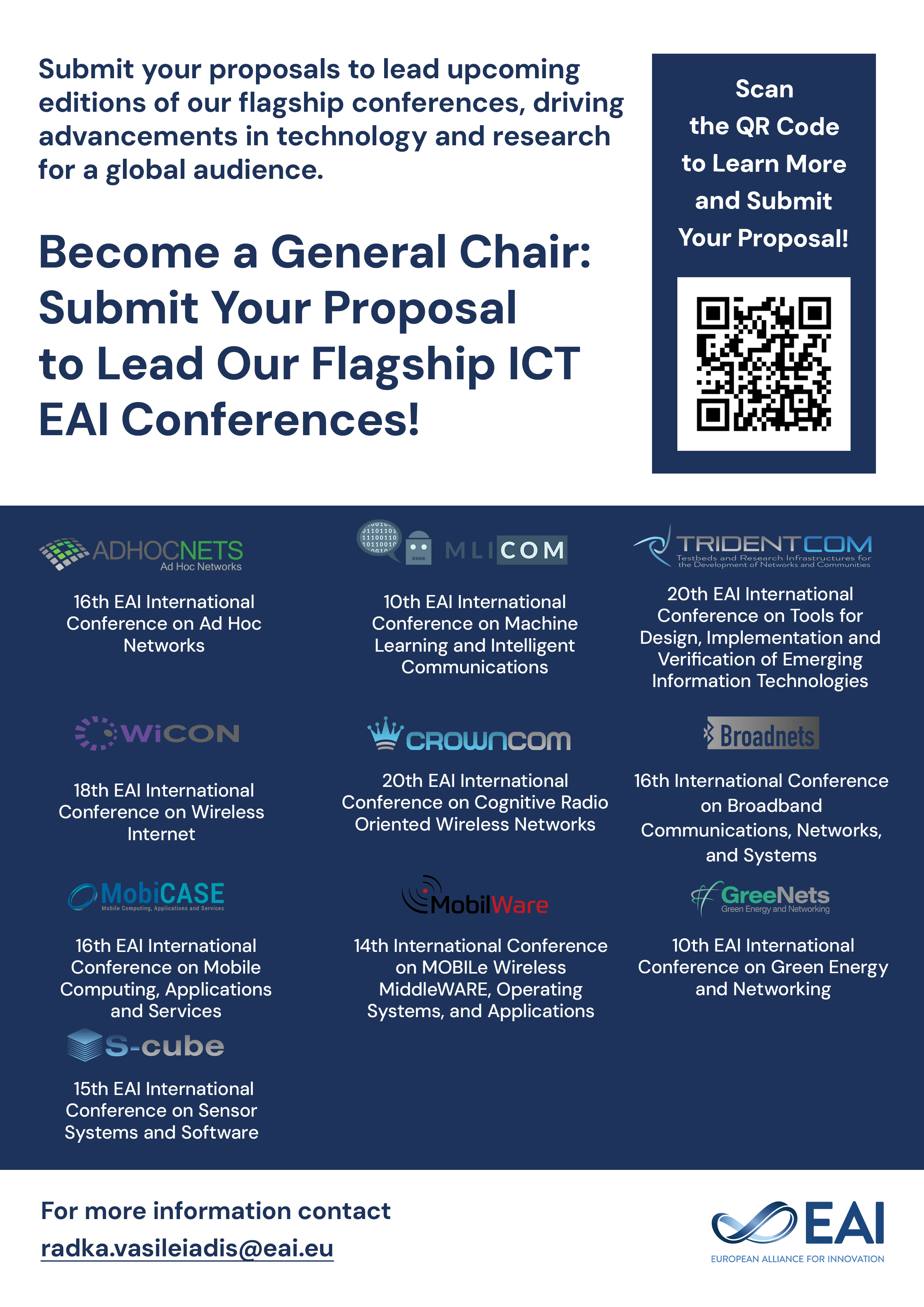
Research Article
Temporal Fusion Transformers Model for Traffic Flow Prediction
@INPROCEEDINGS{10.4108/eai.6-1-2023.2330350, author={Yuxuan Zhou}, title={Temporal Fusion Transformers Model for Traffic Flow Prediction}, proceedings={Proceedings of the 2nd International Conference on Big Data Economy and Digital Management, BDEDM 2023, January 6-8, 2023, Changsha, China}, publisher={EAI}, proceedings_a={BDEDM}, year={2023}, month={6}, keywords={transformer attention temporal fusion transformers model}, doi={10.4108/eai.6-1-2023.2330350} }
- Yuxuan Zhou
Year: 2023
Temporal Fusion Transformers Model for Traffic Flow Prediction
BDEDM
EAI
DOI: 10.4108/eai.6-1-2023.2330350
Abstract
Temporal Fusion Transformers (TFT) is a Transformer model for multi-step forecasting tasks. Because TFT models can integrate decoders to import various types of inputs, including static covariates, known future inputs, and other exogenous time series observed only in the past, which are well performed in the multi-step prediction of time series. To learn temporal relationships at different scales, TFT uses a cyclic layer for local processing and an interpretable self-attention layer for long-term dependence. TFT leverages specialized components to select relevant functions and inhibits unnecessary components through a series of gating layers to achieve high performance in a wide range of scenarios. When the model was proposed, it was considered to have good interpretability. As the research continues to increase, people put forward a lot of different opinions about this. This paper focuses on the explain ability of the TFT model and its attention mechanism.