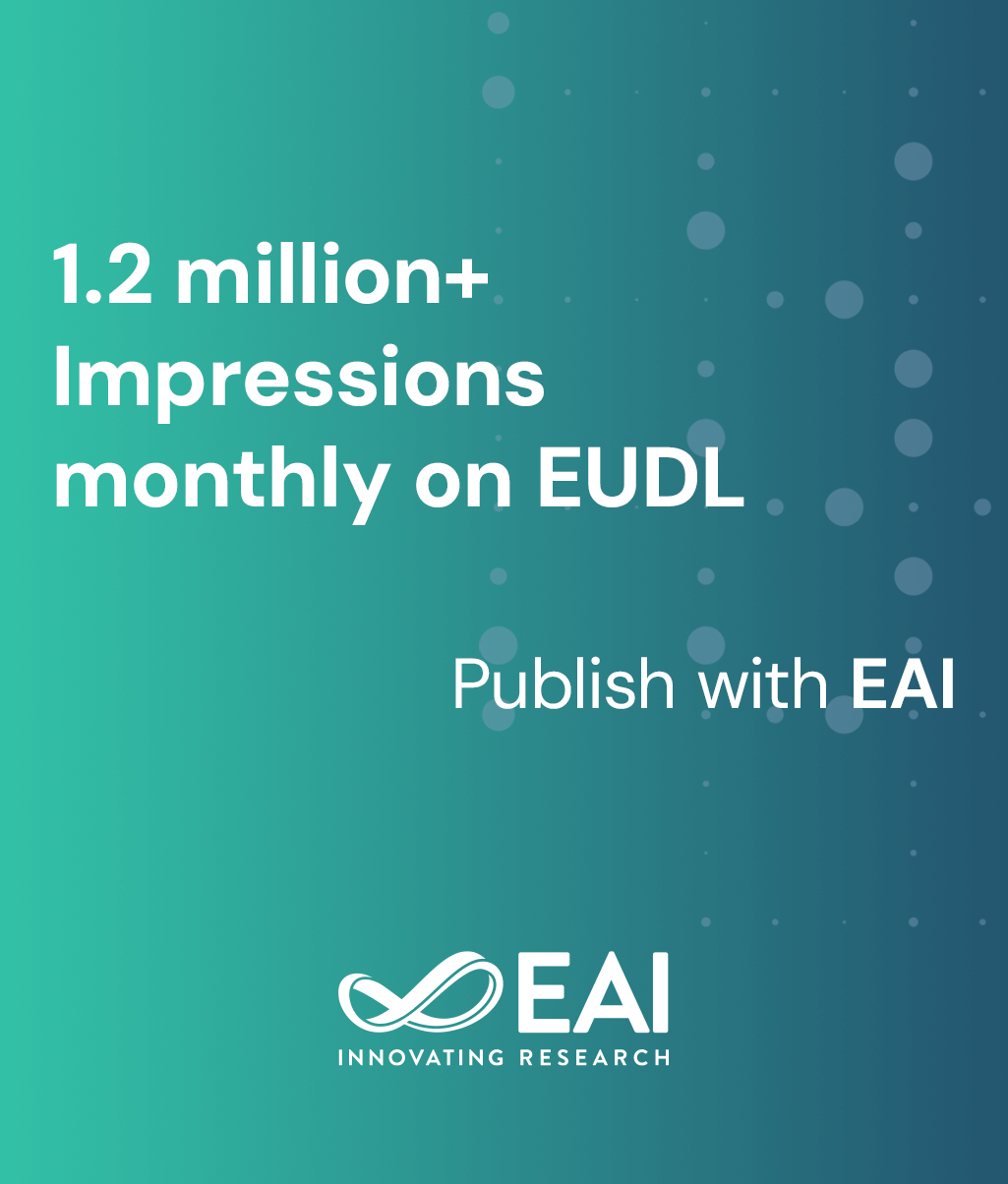
Research Article
A Hybrid Model Integrating LSTM with Multiple GARCH-Type Models for Volatility and Var Forecast
@INPROCEEDINGS{10.4108/eai.6-1-2023.2330313, author={Jiayi Liu}, title={A Hybrid Model Integrating LSTM with Multiple GARCH-Type Models for Volatility and Var Forecast}, proceedings={Proceedings of the 2nd International Conference on Big Data Economy and Digital Management, BDEDM 2023, January 6-8, 2023, Changsha, China}, publisher={EAI}, proceedings_a={BDEDM}, year={2023}, month={6}, keywords={volatility garch-type models lstm model}, doi={10.4108/eai.6-1-2023.2330313} }
- Jiayi Liu
Year: 2023
A Hybrid Model Integrating LSTM with Multiple GARCH-Type Models for Volatility and Var Forecast
BDEDM
EAI
DOI: 10.4108/eai.6-1-2023.2330313
Abstract
The prediction of volatility and Value-at-Risk is a key problem in finance which can help to measure the risk, or the error sizes obtained in modelling several financial variables. In this study, I use high-frequency data to calculate the realized volatility which will be implemented as a financial asset volatility measurement. Furthermore, a hybrid model that integrates three GARCH-type models and LSTM (Long Short-Term Memory) neural networks is proposed to forecast the volatility of CSI 300 index and further translate to accurate VaR (Value at Risk). Then the performance of the hybrid model prediction is compared against the performance of standalone models such as LSTM with RV as the only input. In the end, I generate the trading strategy signal and built the strategy for investment to explore the applicability of this hybrid model in risk management. The empirical analysis of the above model is carried out on CSI 300 index data. The empirical results in this study showed that the hybrid model could significantly improve the volatility and VaR prediction performance of the CSI 300 Index. Therefore, the research methods and the conclusions of this study could provide the possibility for the further application of this hybrid model in financial research.