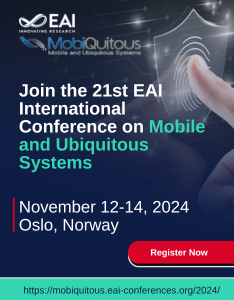
Research Article
Effective sensor positioning to localize target transmitters in a Cognitive Radio Network
@ARTICLE{10.4108/eai.5-4-2016.151145, author={Audri Biswas and Sam Reisenfeld and Mark Hedley and Zhuo Chen}, title={Effective sensor positioning to localize target transmitters in a Cognitive Radio Network}, journal={EAI Endorsed Transactions on Cognitive Communications}, volume={2}, number={6}, publisher={EAI}, journal_a={COGCOM}, year={2016}, month={4}, keywords={Cognitive Radio, Compressive Sensing, Radio Environment Map, Localization, Power Measurements.}, doi={10.4108/eai.5-4-2016.151145} }
- Audri Biswas
Sam Reisenfeld
Mark Hedley
Zhuo Chen
Year: 2016
Effective sensor positioning to localize target transmitters in a Cognitive Radio Network
COGCOM
EAI
DOI: 10.4108/eai.5-4-2016.151145
Abstract
A precise positioning of transmitting nodes enhances the performance of Cognitive Radio (CR), by enabling more efficient dynamic allocation of channels and transmit powers for unlicensed users. Most localization techniques rely on random positioning of sensor nodes where, few sensor nodes may have a small separation between adjacent nodes. Closely spaced nodes introduces correlated observations, effecting the performance of Compressive Sensing (CS) algorithm. This paper introduces a novel minimum distance separation aided compressive sensing algorithm (MDACS). The algorithm selectively eliminates Secondary User (SU) power observations from the set of SU receiving terminals such that pairs of the remaining SUs are separated by a minimum geographic distance.We have evaluated the detection of multiple sparse targets locations and error in l2-norm of the recovery vector. The proposed method offers an improvement in detection ratio by 20% while reducing the error in l2-norm by 57%.
Copyright © 2016 A. Biswas and S. Reisenfeld, licensed to EAI. This is an open access article distributed under the terms of the Creative Commons Attribution license (http://creativecommons.org/licenses/by/3.0/), which permits unlimited use, distribution and reproduction in any medium so long as the original work is properly cited.