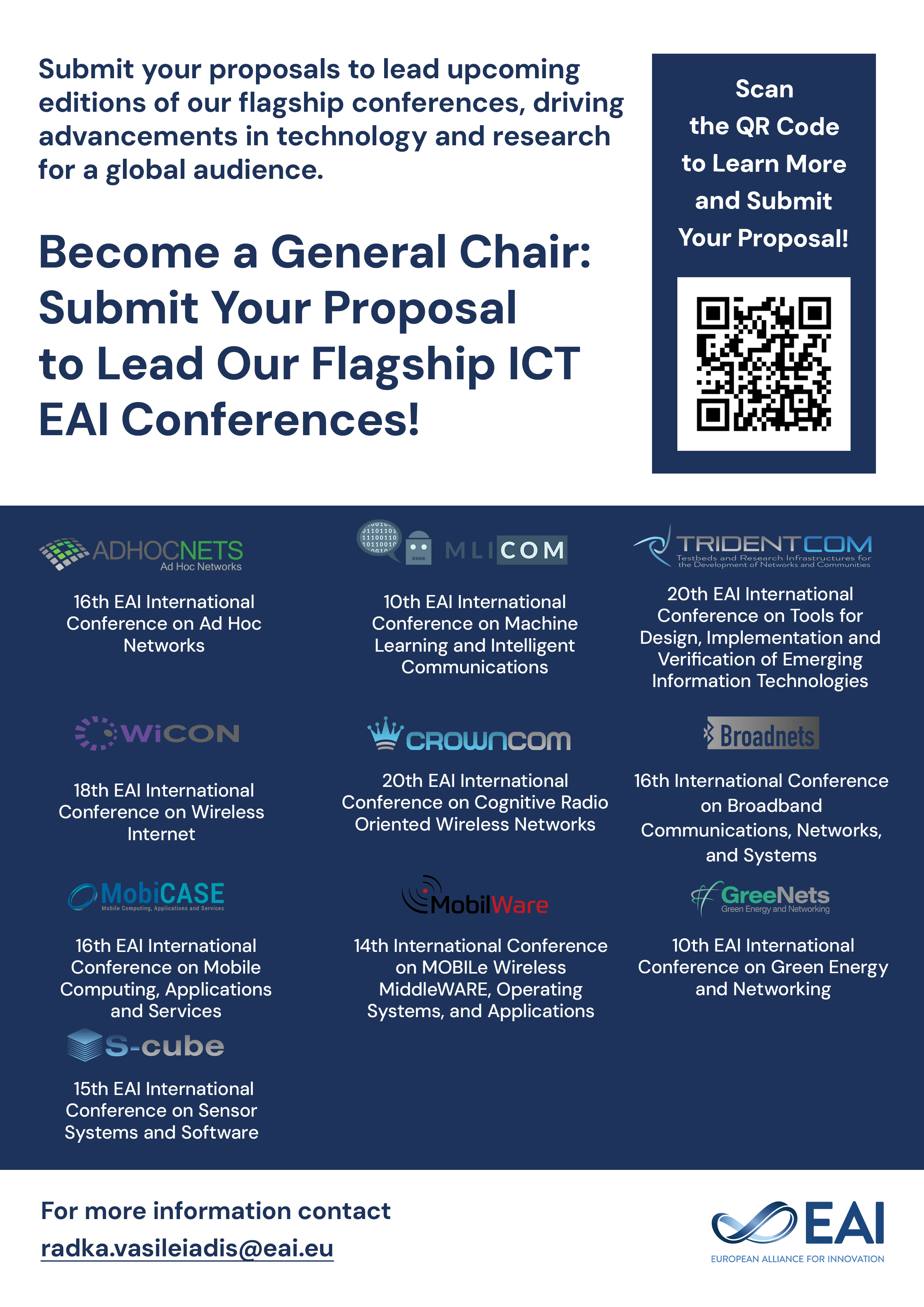
Research Article
Design of Voice Command In Smart Wheelchair Using Hmm Method
@INPROCEEDINGS{10.4108/eai.30-8-2021.2311520, author={Achmad Hidayatno and Sumardi Sumardi and David Kristian Adi Putra and M Hilal Bayu Aji and Arum Patmadani}, title={Design of Voice Command In Smart Wheelchair Using Hmm Method}, proceedings={Proceedings of the 2nd International Conference on Industrial and Technology and Information Design, ICITID 2021, 30 August 2021, Yogyakarta, Indonesia}, publisher={EAI}, proceedings_a={ICITID}, year={2021}, month={10}, keywords={wheelchair voice instructions hmm pocketsphinx raspberry pi}, doi={10.4108/eai.30-8-2021.2311520} }
- Achmad Hidayatno
Sumardi Sumardi
David Kristian Adi Putra
M Hilal Bayu Aji
Arum Patmadani
Year: 2021
Design of Voice Command In Smart Wheelchair Using Hmm Method
ICITID
EAI
DOI: 10.4108/eai.30-8-2021.2311520
Abstract
A wheelchair is a tool used for people with disabilities to be able to move from one place to another. Wheelchairs are used not only in a horizontal place but can be used in a higher place. Wheelchairs are also not only used for people with foot disabilities but are used by hospital patients, the elderly, and people who are at high risk of injury when walking alone. In general, a wheelchair that is often used is a standard wheelchair that is used in hospitals with operations using human labor or assisted by others. However, the use of a standard wheelchair is considered quite difficult because it requires enough energy to be able to move the wheelchair if no one else is helping. Along with the times, motorized wheelchairs have been built and operated using joysticks. The addition of a motor to the wheel helps the user so that the user does not need to use power anymore in moving the wheelchair. In this paper, we develops the ability of a wheelchair that can move using voice instructions. We use the Hidden Markov Model (HMM) method which is utilized in the Pocketsphinx library. This library supports the speech recognition feature to recognize spoken words that implemented at Raspberry Pi mini-computer with the Raspbian operating system. Based on the test results, the average success the word of “maju” 97%, the word of “mundur” 88.5%, the word of “kanan” 94%, the word of “kiri” 92%, the word of “stop” 98.5%, the word of “tamu” 97.5%, the word of “tidur” 91.5%, the wo