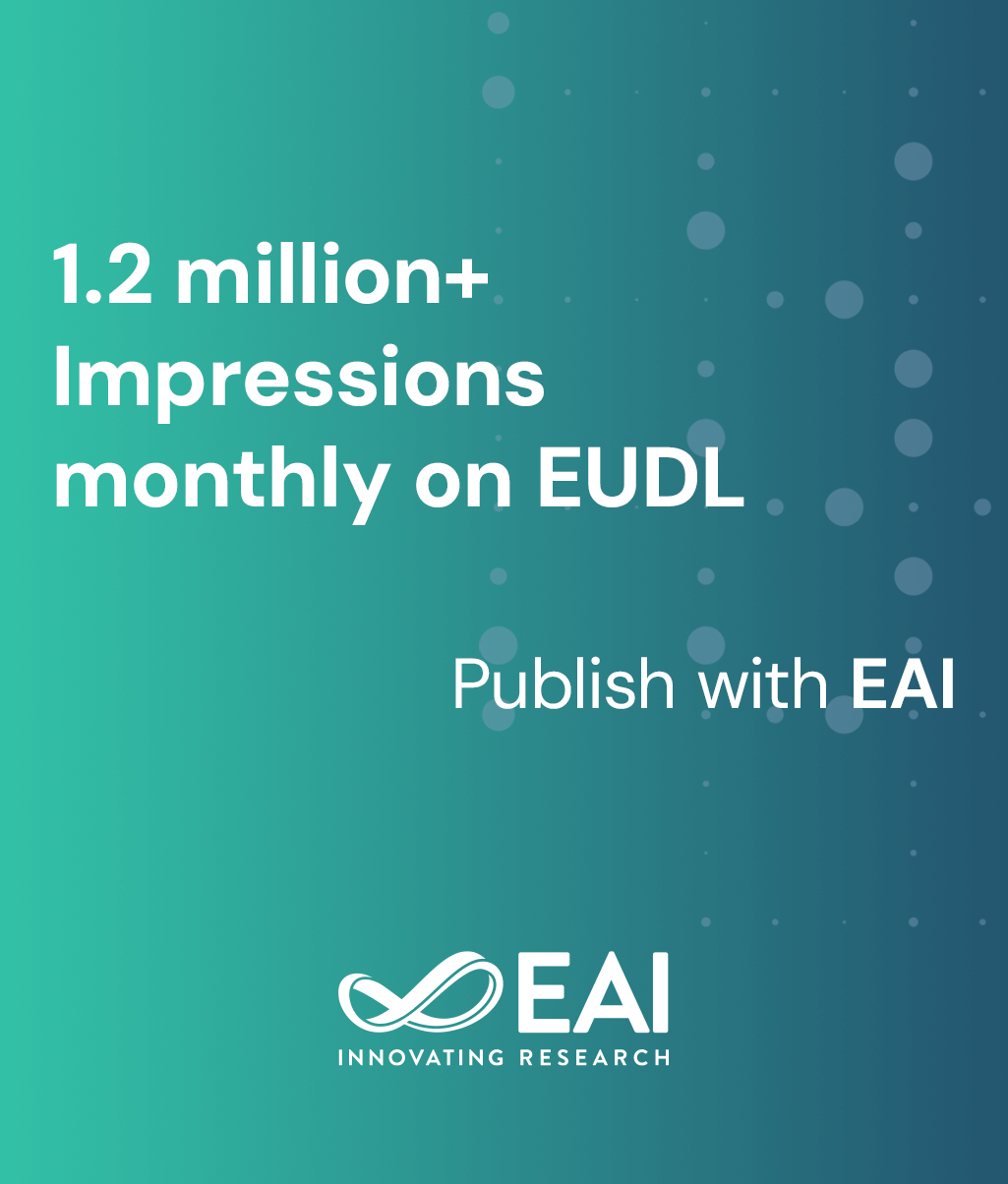
Research Article
A spatio-temporal attention fusion model for students behaviour recognition
@ARTICLE{10.4108/eai.3-9-2021.170905, author={Xiaoli Wang}, title={A spatio-temporal attention fusion model for students behaviour recognition}, journal={EAI Endorsed Transactions on Scalable Information Systems}, volume={9}, number={34}, publisher={EAI}, journal_a={SIS}, year={2021}, month={9}, keywords={student behavior, spatio-temporal attention, channel information, multi-spatial attention, CNN}, doi={10.4108/eai.3-9-2021.170905} }
- Xiaoli Wang
Year: 2021
A spatio-temporal attention fusion model for students behaviour recognition
SIS
EAI
DOI: 10.4108/eai.3-9-2021.170905
Abstract
Student behavior analysis can reflect students' learning situation in real time, which provides an important basis for optimizing classroom teaching strategies and improving teaching methods. It is an important task for smart classroom to explore how to use big data to detect and recognize students behavior. Traditional recognition methods have some defects, such as low efficiency, edge blur, time-consuming, etc. In this paper, we propose a new students behaviour recognition
method based on spatio-temporal attention fusion model. It makes full use of key spatio-temporal information of video, the problem of spatio-temporal information redundancy is solved. Firstly, the channel attention mechanism is introduced into the spatio-temporal network, and the channel information is calibrated by modeling the dependency relationship between feature channels. It can improve the expression ability of features. Secondly, a time attention model based on convolutional neural network (CNN) is proposed, which uses fewer parameters to learn the attention score of each frame, focusing on the frames with obvious behaviour amplitude. Meanwhile, a multi-spatial attention model is presented to calculate the attention score of each position in each frame from different angles, extract several saliency areas of behaviour, and fuse the spatio-temporal features to further enhance the feature representation of video. Finally, the fused features are input into the classification network, and the behaviour recognition results are obtained by combining the two output streams according to different weights. Experiment results on HMDB51, UCF101 datasets and eight typical classroom behaviors of students show that the proposed method can effectively recognize the behaviours in videos. The accuracy of HMDB51 is higher than 90%, that of UCF101 and real data are higher than 90%.
Copyright © 2021 Xiaoli Wang et al., licensed to EAI. This is an open access article distributed under the terms of the Creative Commons Attribution license, which permits unlimited use, distribution and reproduction in any medium so long as the original work is properly cited.