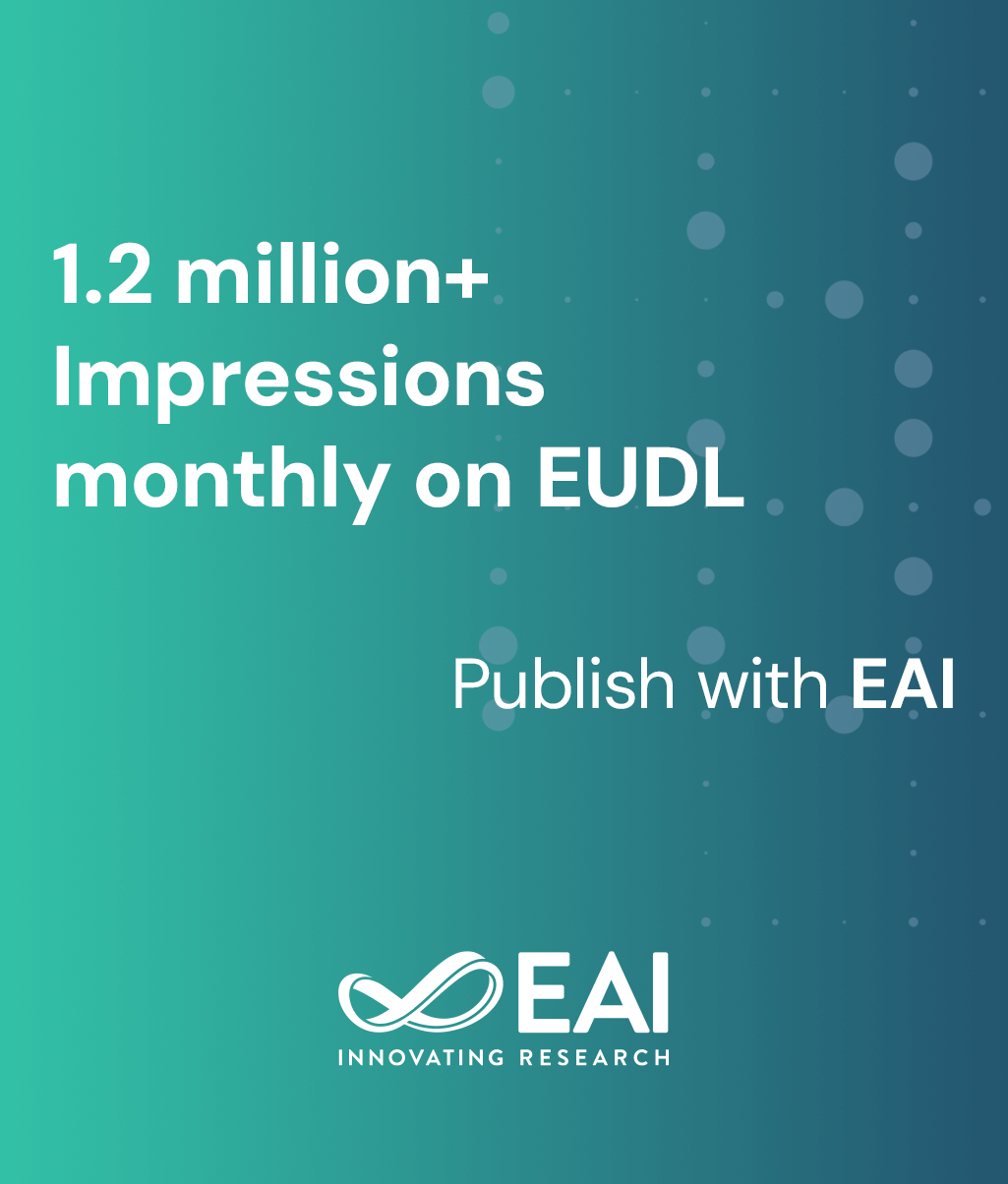
Research Article
Mobility and Fault Aware Adaptive Task Offloading in Heterogeneous Mobile Cloud Environments
@ARTICLE{10.4108/eai.3-9-2019.159947, author={Abdullah Lakhan and Xiaoping Li}, title={Mobility and Fault Aware Adaptive Task Offloading in Heterogeneous Mobile Cloud Environments}, journal={EAI Endorsed Transactions on Mobile Communications and Applications}, volume={5}, number={16}, publisher={EAI}, journal_a={MCA}, year={2019}, month={1}, keywords={Task Offloading, Software Defined Network (SDN), MATOA, Mobility, DHEFT, Edge server (cloudlet), DC (data center), Workflow Task Scheduling}, doi={10.4108/eai.3-9-2019.159947} }
- Abdullah Lakhan
Xiaoping Li
Year: 2019
Mobility and Fault Aware Adaptive Task Offloading in Heterogeneous Mobile Cloud Environments
MCA
EAI
DOI: 10.4108/eai.3-9-2019.159947
Abstract
Nowadays, Mobile Cloud Computing (MCC) has become a predominant prototype for fetching the benefits of cloud computing to mobile devices’ propinquity. Service availability in addition to performance enhancement and mobility features is a preliminary goal in MCC. This paper proposes a mobility aware adaptive offloading framework, known as Mob-Cloud, which includes a mobile device as a thick client, ad-hoc networking, cloudlet DC, and remote cloud services, to augment the performance and availability of the MCC services. However, the impact of dynamic changes in a mobile content (e.g., network status, bandwidth, latency, and location) for the task offloading model observes through proposing a mobility aware adaptive task offloading algorithm (MATOA), which makes a task offloading decision at runtime on selecting optimal wireless network channels and suitable resources for offloading. In this paper, we are formulating the decision problem, and it is well-known as an NP-hard problem. Nonetheless, MATOA has the following phases for the entire Mob-Cloud model: (i) adaptive offloading decision based on real-time information, (ii) workflow task scheduling phase, (iii) mobility model phase to motivate end-user invoke cloud services seamlessly while roaming, and (iv) faulttolerant phase to deal with failure (either network or node). We carry out actual real-life experiments at the implemented instruments to evaluate the overall performance of the MATOA algorithm. Evaluation results prove that MATOA adopts dynamic changes on offloading decision during run-time, and meet an enormous reduction in the total response time with the improved service availability whilst in comparison with the baseline task offloading strategies.
Copyright © 2019 Abdullah Lakhan et al., licensed to EAI. This is an open access article distributed under the terms of the Creative Commons Attribution license (http://creativecommons.org/licenses/by/3.0/), which permits unlimited use, distribution and reproduction in any medium so long as the original work is properly cited.