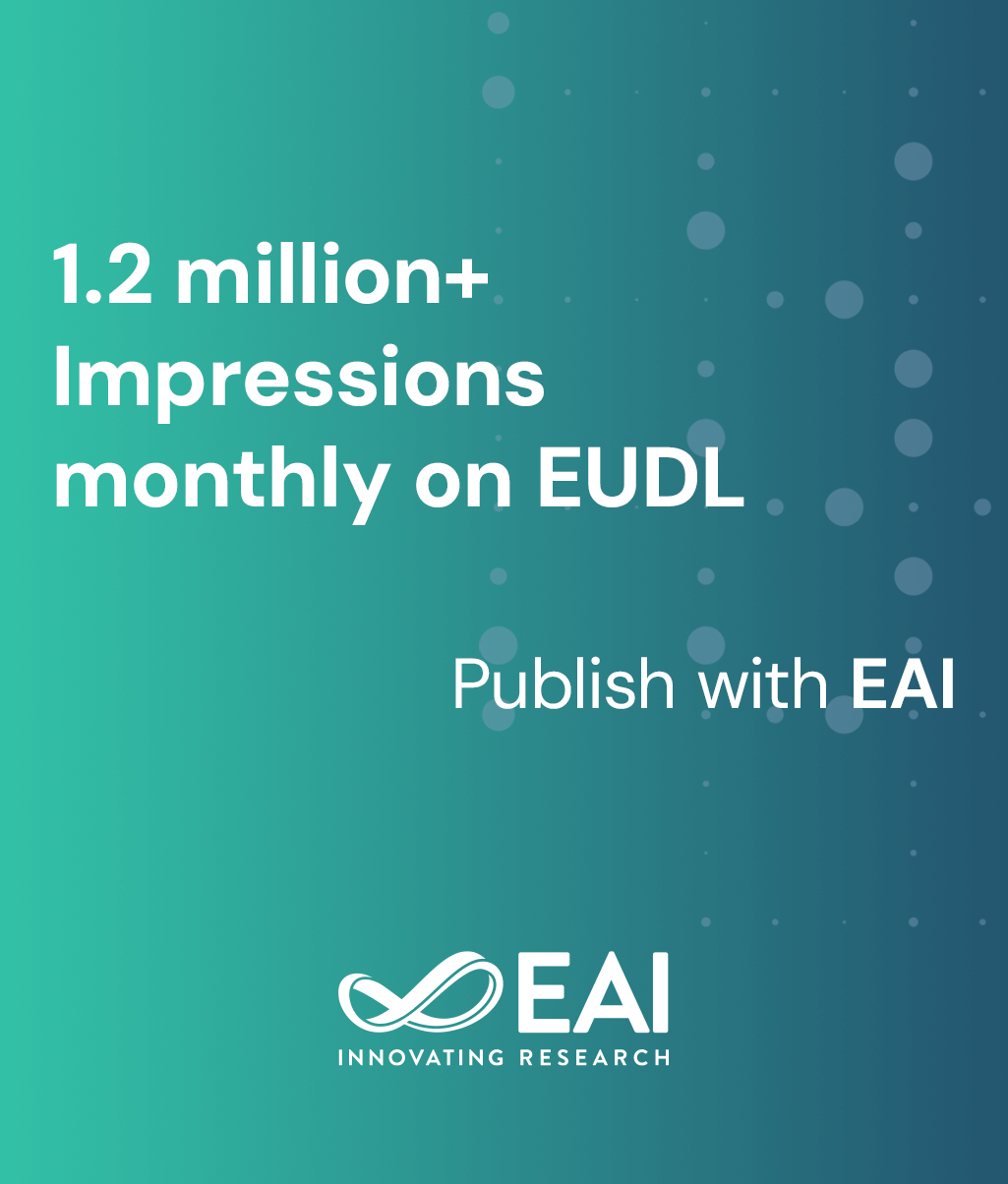
Research Article
Clustering based Contact Tracing Analysis and Prediction of SARS-CoV-2 Infections
@ARTICLE{10.4108/eai.3-11-2021.171756, author={Meenu Gupta and Rakesh Kumar and Sunil Kumar Chawla and Sunny Mishra and Sourabh Dhiman}, title={Clustering based Contact Tracing Analysis and Prediction of SARS-CoV-2 Infections}, journal={EAI Endorsed Transactions on Scalable Information Systems}, volume={9}, number={35}, publisher={EAI}, journal_a={SIS}, year={2021}, month={11}, keywords={Clustering algorithm, Contact tracing, DBSCAN, SARS-CoV-2, COVID-19}, doi={10.4108/eai.3-11-2021.171756} }
- Meenu Gupta
Rakesh Kumar
Sunil Kumar Chawla
Sunny Mishra
Sourabh Dhiman
Year: 2021
Clustering based Contact Tracing Analysis and Prediction of SARS-CoV-2 Infections
SIS
EAI
DOI: 10.4108/eai.3-11-2021.171756
Abstract
INTRODUCTION: Contact tracing is a method to track the victims, which have been infected from the host with any particular disease. Therefore, clustering based machine learning techniques can be employed for contact tracing. Contact tracing can be automated by using technology and thus helps us in producing much more accurate and efficient results.
OBJECTIVES: This work aims at finding usefulness of clustering techniques for contact tracing. Two different clustering techniques namely density-based clustering and partitioning-based clustering have been used to analyse corresponding results for COVID-19 infected cases. The dataset is generated from a mock data generator with certain assumptions.
RESULTS: The paper compares DBSCAN and K-means for contact tracing for COVID-19 Pandemic. The comparative analysis of two algorithms is presented.
CONCLUSION: The effectiveness of certain clustering algorithms in COVID-19 contact tracing is analysed. DBSCAN performs well for clustering tasks. This work only focuses on possible techniques useful for contact tracing and does not claim any medical accuracy.
Copyright © 2021 Meenu Gupta et al., licensed to EAI. This is an open access article distributed under the terms of the Creative Commons Attribution license, which permits unlimited use, distribution and reproduction in any medium so long as the original work is properly cited.