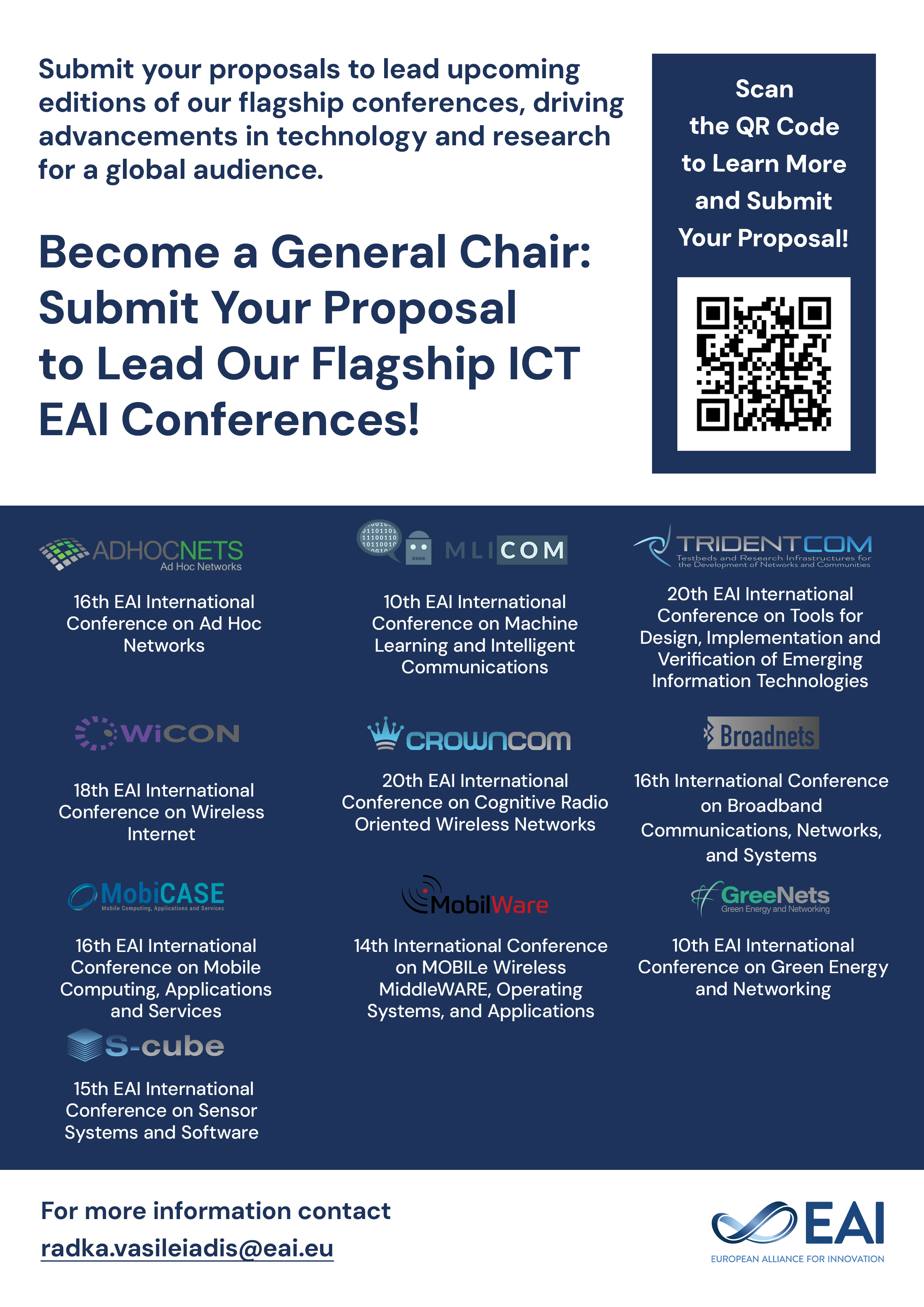
Research Article
Ambient Air Quality Estimation using Supervised Learning Techniques
@ARTICLE{10.4108/eai.29-7-2019.159628, author={Jasleen Kaur Sethi and Mamta Mittal}, title={Ambient Air Quality Estimation using Supervised Learning Techniques}, journal={EAI Endorsed Transactions on Scalable Information Systems}, volume={6}, number={22}, publisher={EAI}, journal_a={SIS}, year={2019}, month={7}, keywords={Air Quality Index, Supervised Learning, Classification, Regression, Voting, Stacking}, doi={10.4108/eai.29-7-2019.159628} }
- Jasleen Kaur Sethi
Mamta Mittal
Year: 2019
Ambient Air Quality Estimation using Supervised Learning Techniques
SIS
EAI
DOI: 10.4108/eai.29-7-2019.159628
Abstract
The exponential increase of population in the urban areas has led to deforestation and industrialization that greatly affects the air quality. The polluted air affects the human health. Due to this concern, the prediction of air quality has become a potential research area. For the assessment of air quality an important indicator is Air Quality Index (AQI). The objective of this paper is to build prediction models using supervised learning. Supervised Learning is broadly classified into: classification, regression and ensemble techniques. This study has been carried out using various techniques of classification, regression and ensemble learning. It has been observed from experimental work that Decision Trees from classification, Support Vector Regression from regression and Stacking Ensemble from ensemble techniques work more effectively and efficiently than the rest of the other techniques that fall under these categories.
Copyright © 2019 Jasleen Kaur Sethi et al., licensed to EAI. This is an open access article distributed under the terms of the Creative Commons Attribution licence (http://creativecommons.org/licenses/by/3.0/), which permits unlimited use, distribution and reproduction in any medium so long as the original work is properly cited.