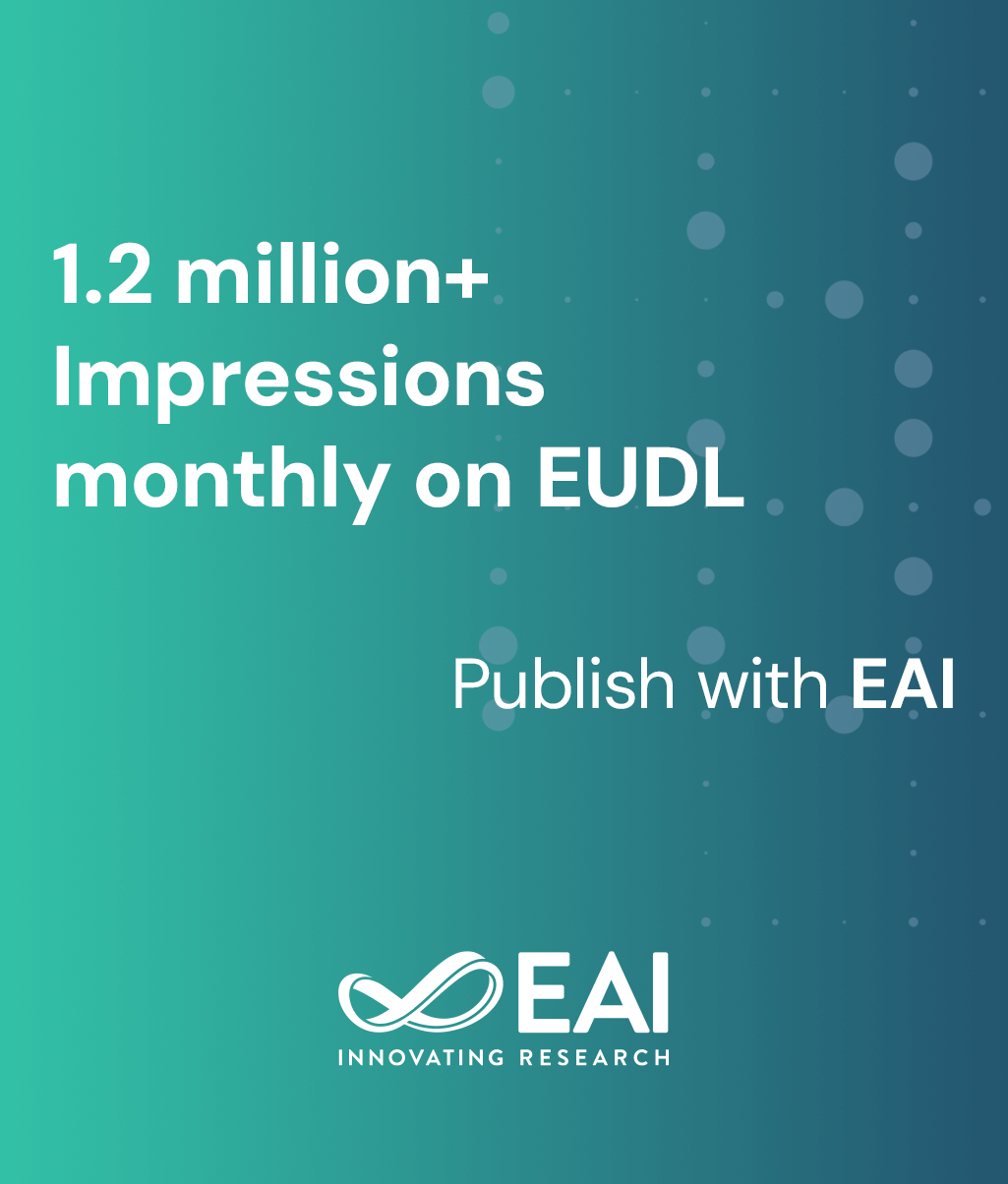
Research Article
A Hierarchical Bayesian Model for Matching Unlabeled Point Sets
@INPROCEEDINGS{10.4108/eai.29-6-2019.2282677, author={Xin Hu and Xiaodong Zhang and Xuequan Zhou and Hua Zhang and Chunshan Li and Deqiong Ding and Dianhui Chu}, title={A Hierarchical Bayesian Model for Matching Unlabeled Point Sets}, proceedings={12th EAI International Conference on Mobile Multimedia Communications, Mobimedia 2019, 29th - 30th Jun 2019, Weihai, China}, publisher={EAI}, proceedings_a={MOBIMEDIA}, year={2019}, month={6}, keywords={hierarchical model markov chain monte carlo matching registration}, doi={10.4108/eai.29-6-2019.2282677} }
- Xin Hu
Xiaodong Zhang
Xuequan Zhou
Hua Zhang
Chunshan Li
Deqiong Ding
Dianhui Chu
Year: 2019
A Hierarchical Bayesian Model for Matching Unlabeled Point Sets
MOBIMEDIA
EAI
DOI: 10.4108/eai.29-6-2019.2282677
Abstract
Point set registration is the key in many scientific disciplines. Target at several challenges in registration (e.g. initial registration, outliers, missing data, and local trap), we propose a robust registration method for two point sets using a hierarchical Bayesian model, which is combined with Markov chain Monte Carlo inference. Our approach is based on the introduction of a template of hidden locations underlying the observed configuration points. A Poisson process prior is assigned to these locations, resulting in a simplified formulation of the model. We make use of a structure containing the relevant information on the matches. We conduct several experiments to demonstrate that our algorithm is accurate and robust.
Copyright © 2019–2025 EAI