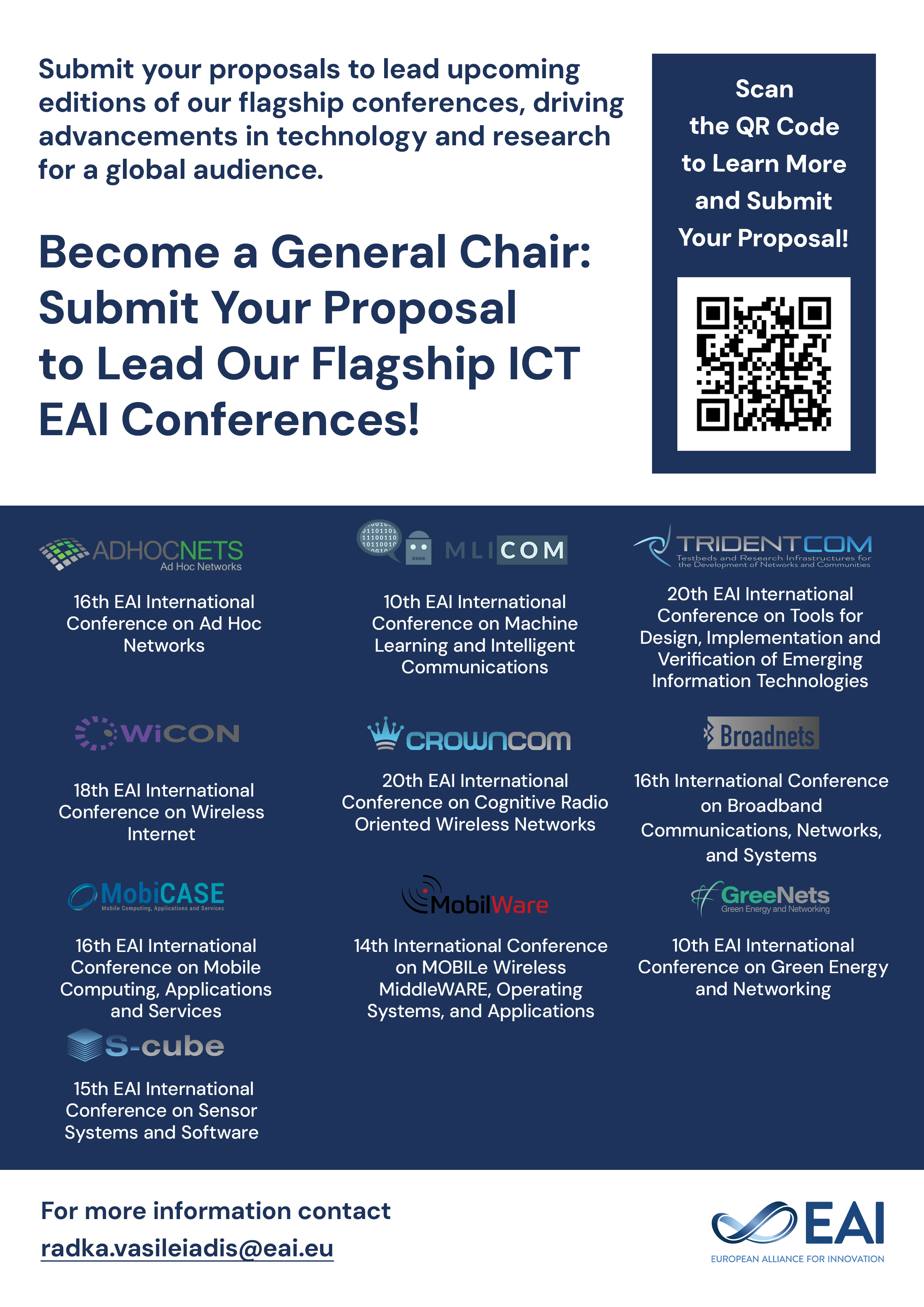
Research Article
Dynamic Computation Offloading Based on Deep Reinforcement Learning
@INPROCEEDINGS{10.4108/eai.29-6-2019.2282108, author={BaiChuan Cheng and ZhiLong Zhang and DanPu Liu}, title={Dynamic Computation Offloading Based on Deep Reinforcement Learning}, proceedings={12th EAI International Conference on Mobile Multimedia Communications, Mobimedia 2019, 29th - 30th Jun 2019, Weihai, China}, publisher={EAI}, proceedings_a={MOBIMEDIA}, year={2019}, month={6}, keywords={computation offloading mobile edge computing reinforcement learning policy gradients}, doi={10.4108/eai.29-6-2019.2282108} }
- BaiChuan Cheng
ZhiLong Zhang
DanPu Liu
Year: 2019
Dynamic Computation Offloading Based on Deep Reinforcement Learning
MOBIMEDIA
EAI
DOI: 10.4108/eai.29-6-2019.2282108
Abstract
Mobile edge computing (MEC) provides computation capability at the edge of wireless network. To reduce the execution delay, computation-intensive multimedia tasks can be offloaded from user equipments (UEs) to the MEC server. How to allocate the computational and wireless resources is one of the key issues to guarantee the quality of services, and is very challenging when tasks are generated dynamically. In this paper, we address the above problem. To minimize the sum execution delay of multiple users, we jointly optimize the offloading decision and the allocation of both computational and wireless resources. We propose a deep policy gradient (DPG) algorithm based on the deep reinforcement learning. Simulation results show that our proposed DPG method can achieve lower latency than the baselines under different numbers of users, computation capacities and wireless bandwidths.