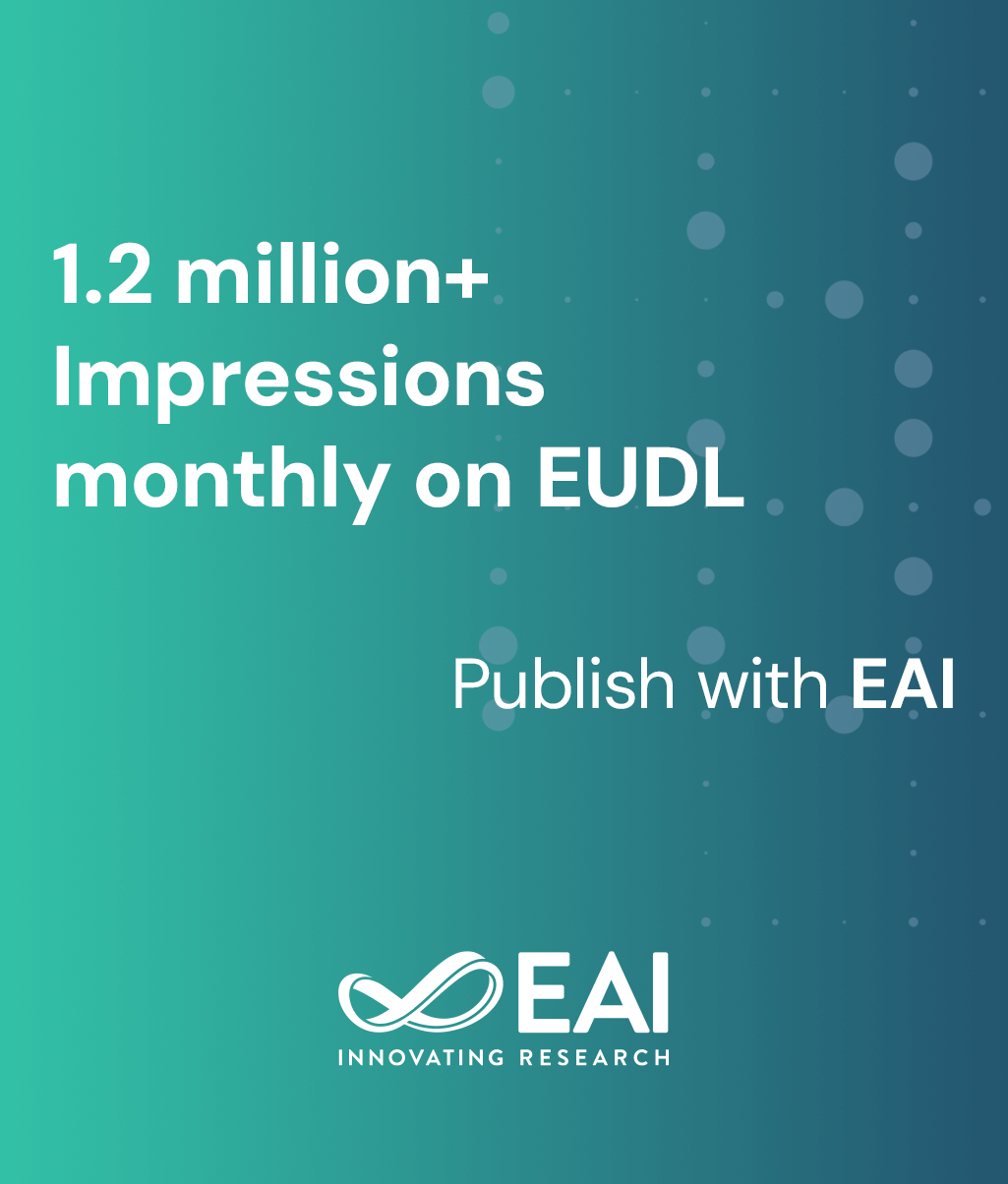
Research Article
A Big-Data based and process-oriented decision support system for traffic management
@ARTICLE{10.4108/eai.29-5-2018.154810, author={Alejandro Vera-Baquero and Ricardo Colomo-Palacios}, title={A Big-Data based and process-oriented decision support system for traffic management}, journal={EAI Endorsed Transactions on Scalable Information Systems}, volume={5}, number={17}, publisher={EAI}, journal_a={SIS}, year={2018}, month={5}, keywords={Traffic Management, Big Data, Cloud Computing, Big Data Analytics, Business Process, Process Monitoring, Event-Driven, Business Intelligence, Decision Support Systems.}, doi={10.4108/eai.29-5-2018.154810} }
- Alejandro Vera-Baquero
Ricardo Colomo-Palacios
Year: 2018
A Big-Data based and process-oriented decision support system for traffic management
SIS
EAI
DOI: 10.4108/eai.29-5-2018.154810
Abstract
Data analysis and monitoring of road networks in terms of reliability and performance are valuable but hard to achieve, especially when the analytical information has to be available to decision makers on time. The gathering and analysis of the observable facts can be used to infer knowledge about traffic congestion over time and gain insights into the roads safety. However, the continuous monitoring of live traffic information produces a vast amount of data that makes it difficult for business intelligence (BI) tools to generate metrics and key performance indicators (KPI) in nearly real-time. In order to overcome these limitations, we propose the application of a big-data based and process-centric approach that integrates with operational traffic information systems to give insights into the road network's efficiency. This paper demonstrates how the adoption of an existent process-oriented DSS solution with big-data support can be leveraged to monitor and analyse live traffic data on an acceptable response time basis.
Copyright © 2018 Alejandro Vera-Baquero and Ricardo Colomo-Palacios, licensed to EAI. This is an open access article distributed under the terms of the Creative Commons Attribution licence (http://creativecommons.org/licenses/by/3.0/), which permits unlimited use, distribution and reproduction in any medium so long as the original work is properly cited.