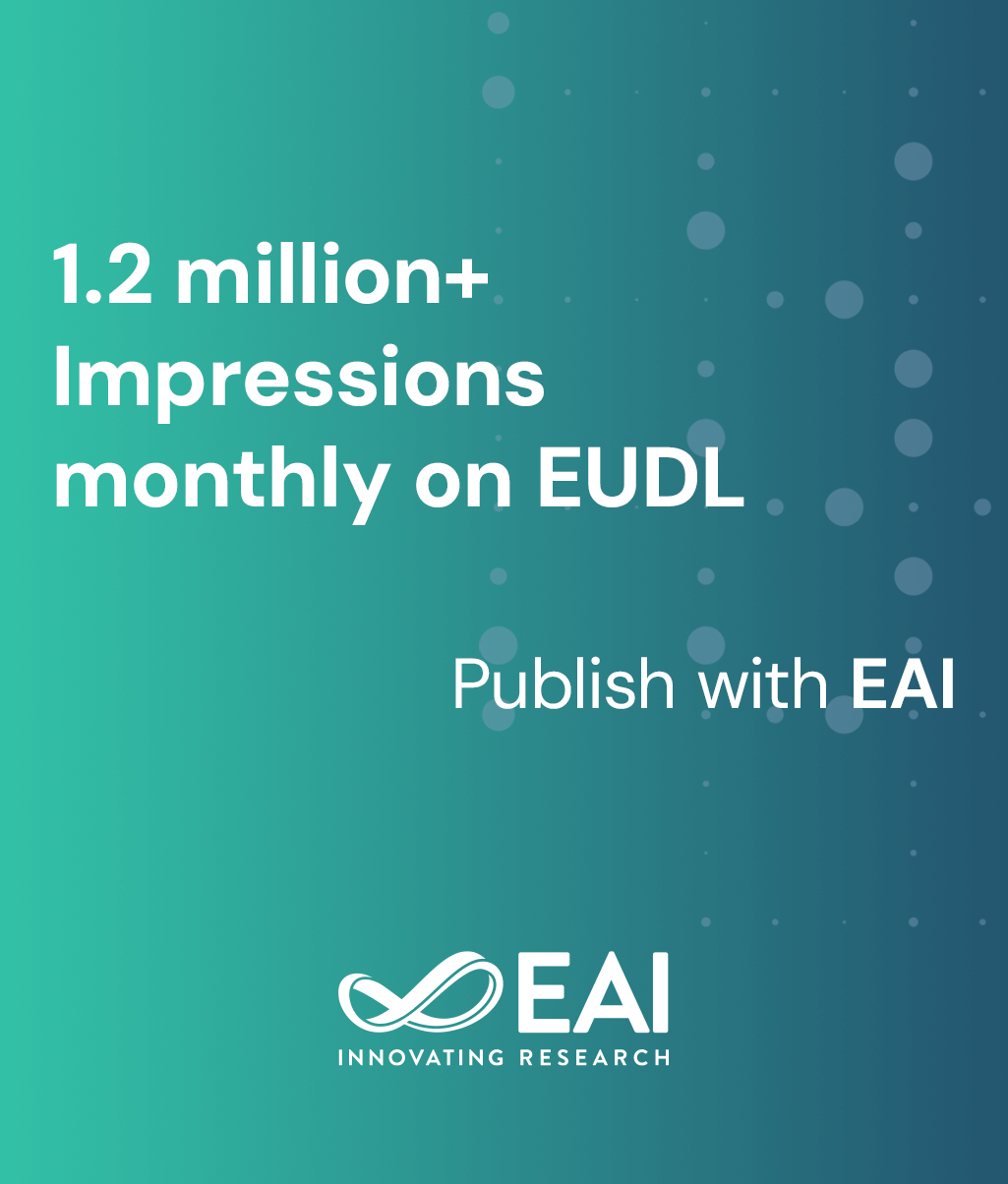
Research Article
Prediction of College Admission Scores Based on an XGBoost-LSTM Hybrid Model
@INPROCEEDINGS{10.4108/eai.29-3-2024.2347687, author={Liangyu Xu}, title={Prediction of College Admission Scores Based on an XGBoost-LSTM Hybrid Model}, proceedings={Proceedings of the 3rd International Conference on Educational Innovation and Multimedia Technology, EIMT 2024, March 29--31, 2024, Wuhan, China}, publisher={EAI}, proceedings_a={EIMT}, year={2024}, month={6}, keywords={xgboost lstm chinese college admission scores}, doi={10.4108/eai.29-3-2024.2347687} }
- Liangyu Xu
Year: 2024
Prediction of College Admission Scores Based on an XGBoost-LSTM Hybrid Model
EIMT
EAI
DOI: 10.4108/eai.29-3-2024.2347687
Abstract
This study introduces a predictive model that combines XGBoost and Long Short-Term Memory (LSTM) networks for forecasting the minimum college admission scores in the Chinese college entrance examination system. By leveraging the strengths of LSTM in handling multivariate time series data and the efficiency of XGBoost in processing categorical data, the fusion model enhances prediction accuracy. The experimental results demonstrate that this hybrid model outperforms individual models in predicting college entrance scores, offering valuable support for educational planning and student decision-making.
Copyright © 2024–2025 EAI