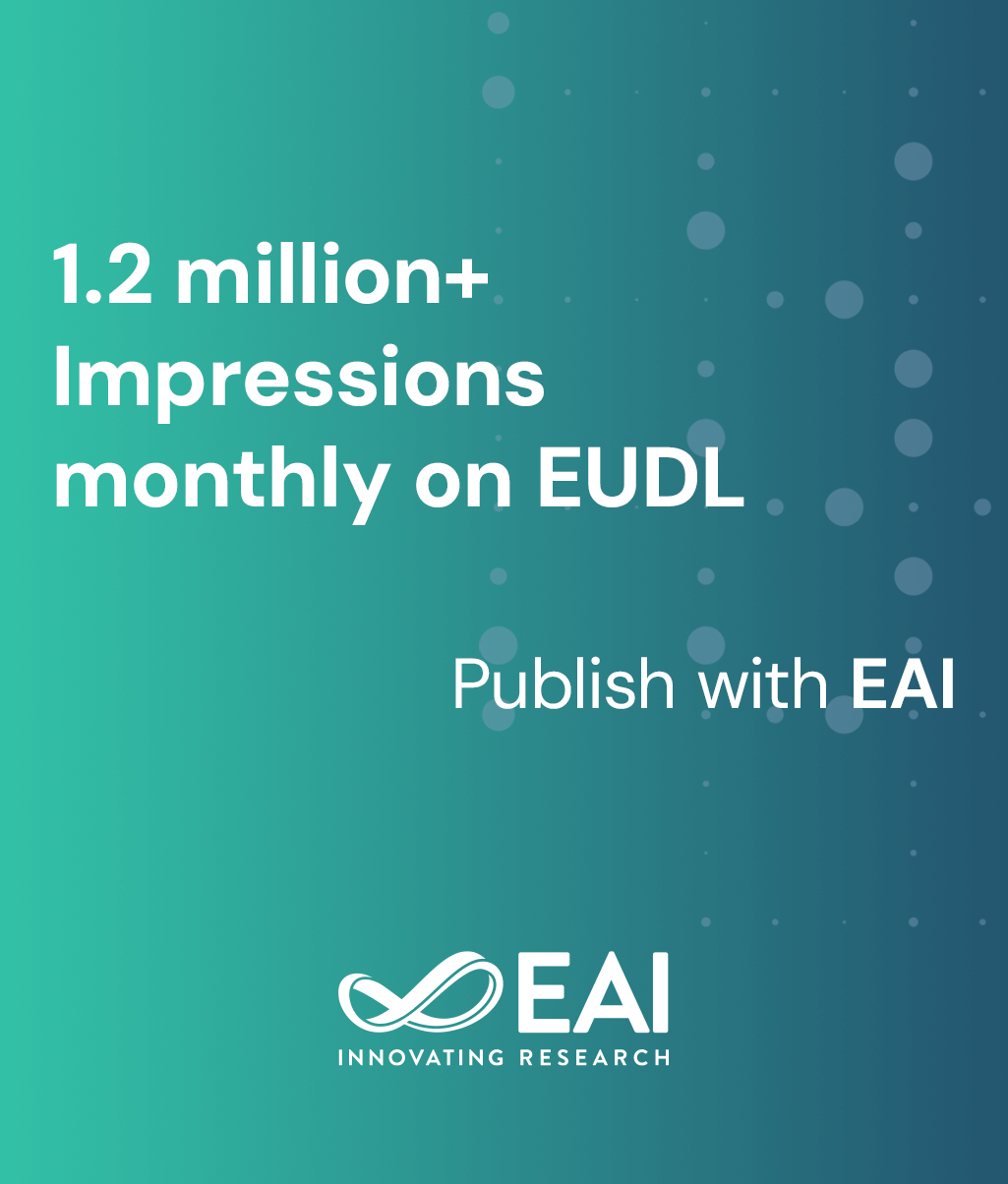
Research Article
Research on the Important Accounts Identification in Blockchain Trading Networks
@INPROCEEDINGS{10.4108/eai.29-3-2024.2347430, author={Min Zhang and Yongsheng Zhao and Zesan Liu and Jian Zhang and Yunxi Fu and Zhenya Wang}, title={Research on the Important Accounts Identification in Blockchain Trading Networks}, proceedings={Proceedings of the 3rd International Conference on Bigdata Blockchain and Economy Management, ICBBEM 2024, March 29--31, 2024, Wuhan, China}, publisher={EAI}, proceedings_a={ICBBEM}, year={2024}, month={6}, keywords={blockchain; complex network; critical node; network security}, doi={10.4108/eai.29-3-2024.2347430} }
- Min Zhang
Yongsheng Zhao
Zesan Liu
Jian Zhang
Yunxi Fu
Zhenya Wang
Year: 2024
Research on the Important Accounts Identification in Blockchain Trading Networks
ICBBEM
EAI
DOI: 10.4108/eai.29-3-2024.2347430
Abstract
Important accounts play an important role in guaranteeing the security and stability of blockchain trading networks, given that attacking them can increase the risk of account theft and disrupt the trade order. Consequently, the identification of critical accounts within blockchain trading networks holds paramount significance. However, previous research usually focuses on individual account features while neglecting the impacts of neighbors, leading to biased assessments and inaccurate ranking lists. To overcome these limitations, this paper proposes the NDL algorithm to identify critical accounts in the blockchain trading networks based on complex network methods. Specifically, NDL utilizes degree centrality to compute the attributes of an account itself, and employs the shortest paths to calculate the attributes of its neighbors. By comprehensively considering the influence of accounts and neighbors, NDL effectively distinguishes their importance. Besides, the Susceptible-Infectious-Recovered (SIR) model is employed to estimate the transmission potential of accounts. In addition, Kendall’s tau correlation coefficient and monotonicity index are employed to assess the effectiveness and distinguishability of NDL. After conducting thorough experiments on four datasets, the findings demonstrate that NDL outperforms six baseline methods.