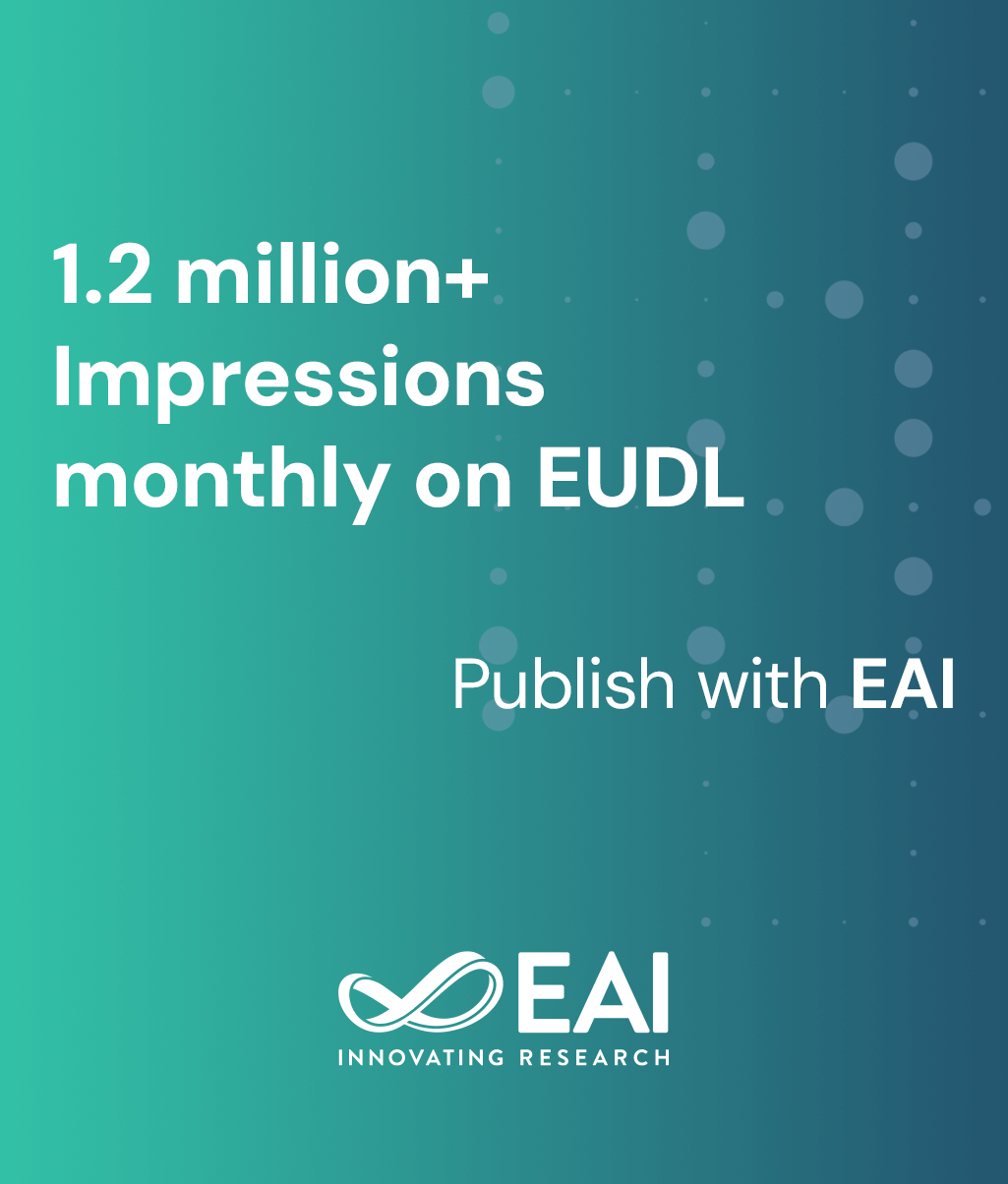
Research Article
AIoT Enabled Traffic Congestion Control System Using Deep Neural Network
@ARTICLE{10.4108/eai.28-9-2021.171170, author={Shahan Yamin Siddiqui and Inzmam Ahmad and Muhammad Adnan Khan and Bilal Shoaib Khan and Muhammad Nadeem Ali and Iftikhar Naseer and Kausar Parveen and Hafiz Muhammad Usama}, title={AIoT Enabled Traffic Congestion Control System Using Deep Neural Network}, journal={EAI Endorsed Transactions on Scalable Information Systems}, volume={8}, number={33}, publisher={EAI}, journal_a={SIS}, year={2021}, month={9}, keywords={Deep neural network (DNN), Traffic congestion control system, AIoT, Smart city, Machine Learning}, doi={10.4108/eai.28-9-2021.171170} }
- Shahan Yamin Siddiqui
Inzmam Ahmad
Muhammad Adnan Khan
Bilal Shoaib Khan
Muhammad Nadeem Ali
Iftikhar Naseer
Kausar Parveen
Hafiz Muhammad Usama
Year: 2021
AIoT Enabled Traffic Congestion Control System Using Deep Neural Network
SIS
EAI
DOI: 10.4108/eai.28-9-2021.171170
Abstract
With rapid population growth in cities, to allow full use of modern technology, transportation networks need to be developed efficiently and sustainability. A significant problem in the traffic motion barrier is dynamic traffic flow. To manage traffic congestion problems, this paper provides a method for forecasting traffic congestion with the aid of a Deep neural network that minimizes blockage and plays a vital role in traffic smoothing. In the proposed model, data is collected and received by using smart Internet of things enabled devices. With the help of this model, data of the previous junction of signals will send to another junction and update after that next layer named as intelligence prediction for the congestion layer will receive data from sensors and the cloud which is used to find out the congestion point. The proposed TC2S- DNN model achieved the accuracy of 98.03 percent and miss rate of 1.97 percent which is better then previous published approaches.
Copyright © 2021 Shahan Yamin Siddiqui et al., licensed to EAI. This is an open access article distributed under the terms of the Creative Commons Attribution licence (http://creativecommons.org/licenses/by/3.0/), which permits unlimited use, distribution and reproduction in any medium so long as the original work is properly cited.