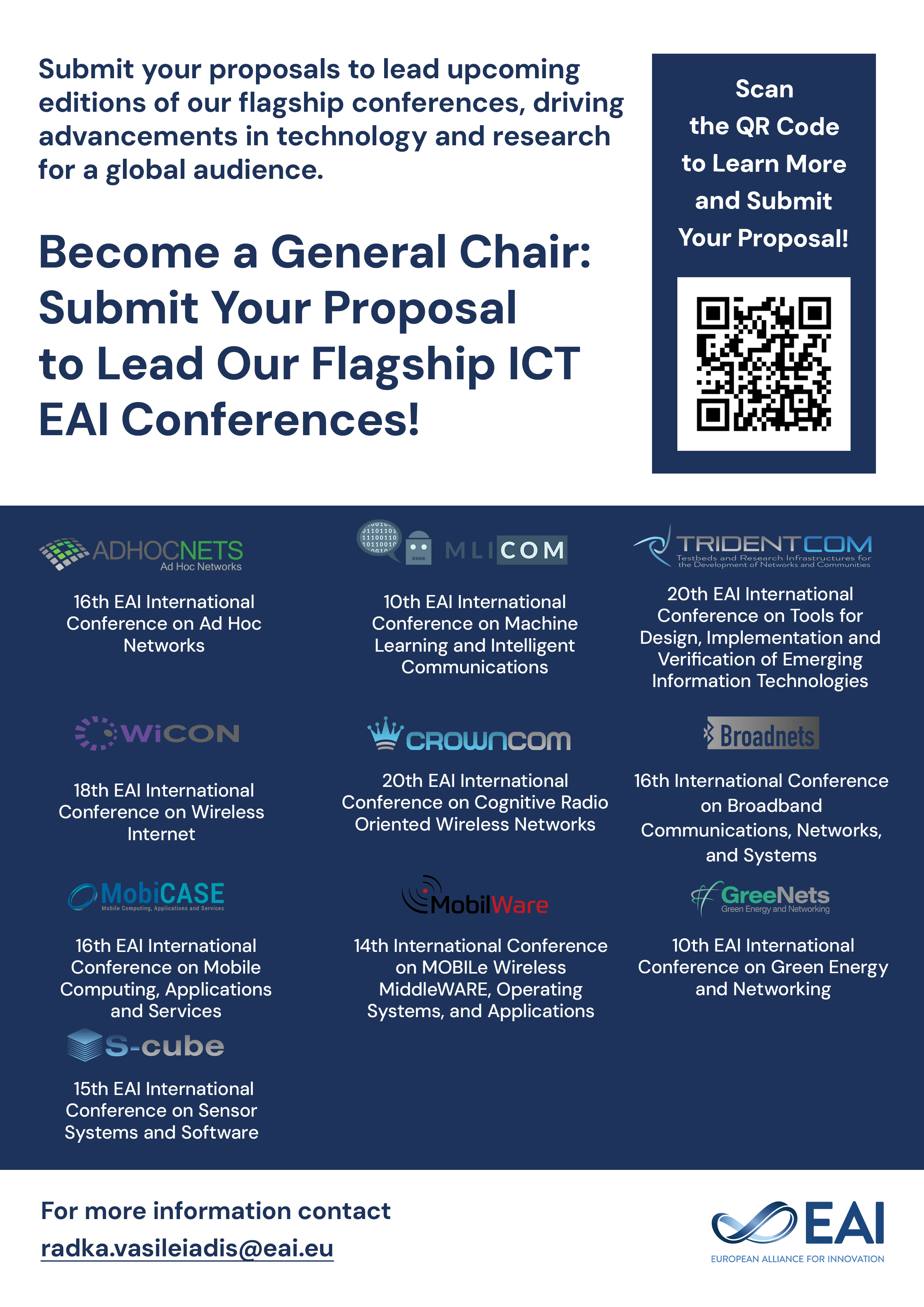
Research Article
Gingivitis detection by Fractional Fourier Entropy and Particle Swarm Optimization
@ARTICLE{10.4108/eai.28-4-2021.169424, author={Yan Yan}, title={Gingivitis detection by Fractional Fourier Entropy and Particle Swarm Optimization}, journal={EAI Endorsed Transactions on e-Learning}, volume={7}, number={21}, publisher={EAI}, journal_a={EL}, year={2021}, month={4}, keywords={Multi-layer perception, gingivitis detection, fractional Fourier entropy, particle swarm optimization, detection algorithm}, doi={10.4108/eai.28-4-2021.169424} }
- Yan Yan
Year: 2021
Gingivitis detection by Fractional Fourier Entropy and Particle Swarm Optimization
EL
EAI
DOI: 10.4108/eai.28-4-2021.169424
Abstract
INTRODUCTION: we propose a detection model of gingivitis based on feature extraction based on particle swarm optimization neural network with fractional Fourier entropy. OBJECTIVES: For the sake of reduce the diagnostic burden of doctors' frequent and high concentration. METHODS: Primarily, Fourier transform is applied to the collected image signal, and the entropy is extracted from the gingival image by Shannon entropy so as to get the input value. The particle swarm optimization algorithm was combined with the extraction eigenvector is used to detect whether patients have gingivitis in final. RESULTS: The experimental results show that this method can reduce the unnecessary space of image detection, reduce the complexity of image information, and achieve a sensitivity of 79.00±1.61%, specificity of 80.89±1.87% and accuracy of 79.94±0.96%. CONCLUSION: This optimized algorithm can effectively and accurately cluster the sample data, and the accuracy is also higher than the advanced gingival image diagnosis method, making the gingivitis diagnosis more accurate. Our neural network has good trainability and recognition ability, which makes a unique contribution to medically intelligent detection methods for gingival treatment.
Copyright © 2021 Yan Yan et al., licensed to EAI. This is an open access article distributed under the terms of the Creative Commons Attribution license, which permits unlimited use, distribution and reproduction in any medium so long as the original work is properly cited.