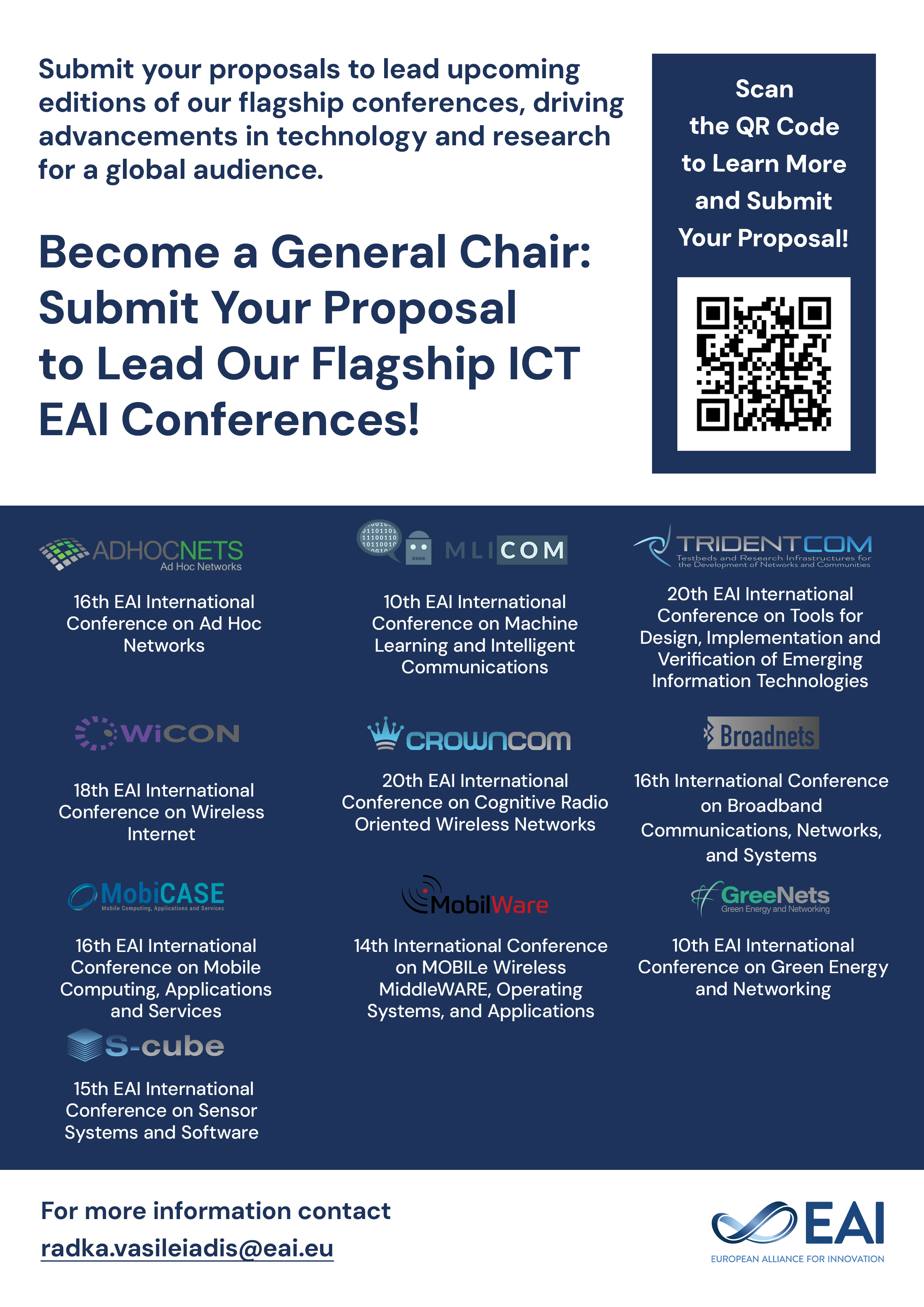
Research Article
Random Aggregation: Differential Privacy in k-Anonymity
@INPROCEEDINGS{10.4108/eai.28-10-2022.2328460, author={Liyuan Yang}, title={Random Aggregation: Differential Privacy in k-Anonymity}, proceedings={Proceedings of the International Conference on Financial Innovation, FinTech and Information Technology, FFIT 2022, October 28-30, 2022, Shenzhen, China}, publisher={EAI}, proceedings_a={FFIT}, year={2023}, month={4}, keywords={random k-anonymity privacy protection statistical disclosure control differential privacy random aggregation microaggregation}, doi={10.4108/eai.28-10-2022.2328460} }
- Liyuan Yang
Year: 2023
Random Aggregation: Differential Privacy in k-Anonymity
FFIT
EAI
DOI: 10.4108/eai.28-10-2022.2328460
Abstract
Microaggregation is used to solve the problem of privacy protection. A new method of microaggregation is proposed in this paper to satisfy differential privacy. It adds random noises to the centroids of equivalence classes, which reduces the risks of differential attacks and link attacks. Since the process of k-partition is very time-consuming, a top-down method is used to divide the original dataset into equivalence classes. Firstly, a matrix of distances is built and the original dataset is divided into several smaller datasets. And then the equivalence classes are formed by splitting datasets repeatedly. The experiments are conducted on two different datasets. Comparing with the existing methods, the results show that the proposed methods have the advantage of high efficiency and less information loss.