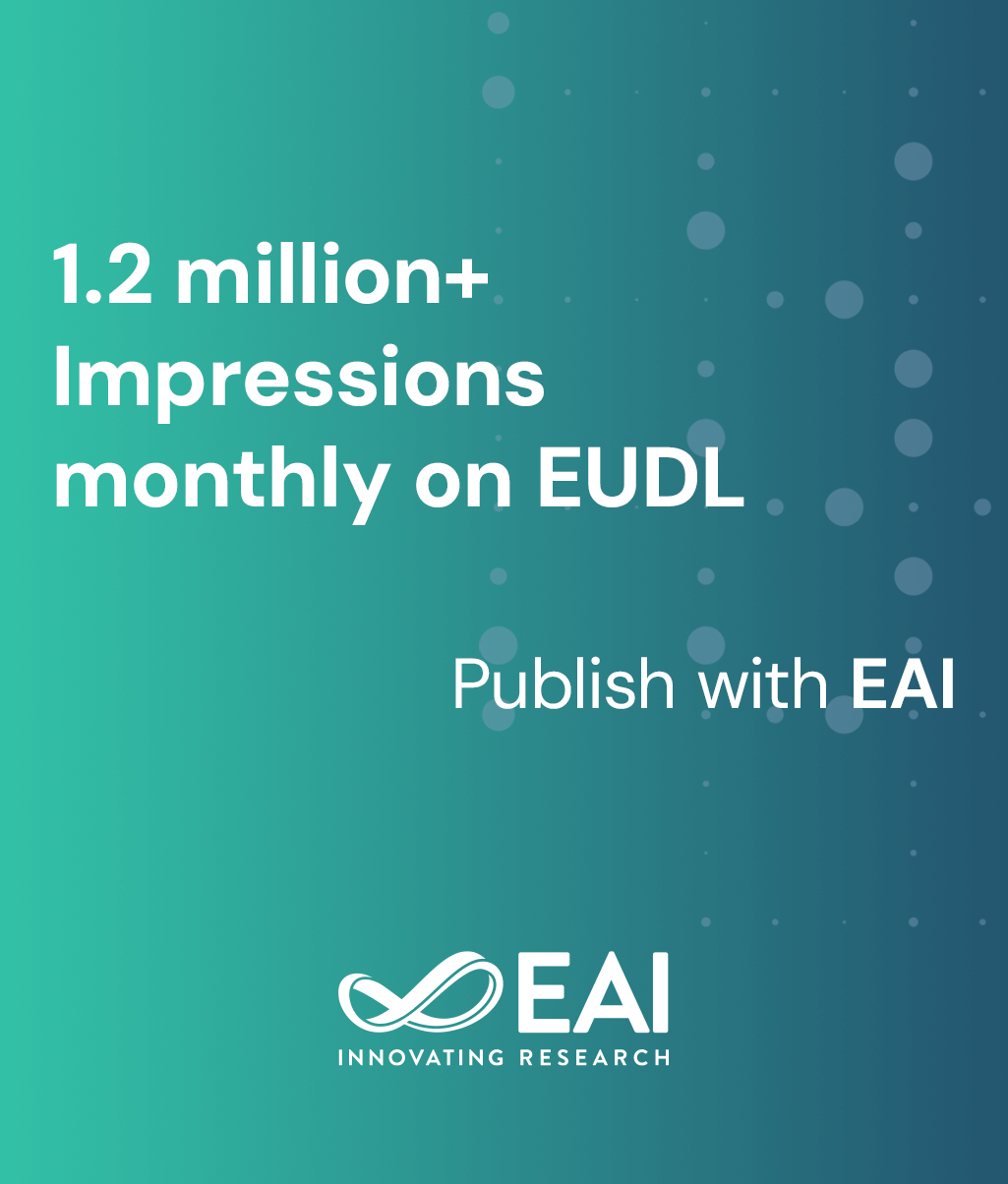
Research Article
Improved Channel Equalization using Deep Reinforcement Learning and Optimization
@ARTICLE{10.4108/eai.28-10-2021.171685, author={Swati Katwal and Vinay Bhatia}, title={Improved Channel Equalization using Deep Reinforcement Learning and Optimization}, journal={EAI Endorsed Transactions on Scalable Information Systems}, volume={9}, number={35}, publisher={EAI}, journal_a={SIS}, year={2021}, month={10}, keywords={Digital communication, Inter Symbol Interference (ISI), Channel equalization, Whale Optimization Algorithm (WOA), and Reinforcement Learning (RL)}, doi={10.4108/eai.28-10-2021.171685} }
- Swati Katwal
Vinay Bhatia
Year: 2021
Improved Channel Equalization using Deep Reinforcement Learning and Optimization
SIS
EAI
DOI: 10.4108/eai.28-10-2021.171685
Abstract
INTRODUCTION: Data transmission through channels observe large distortions arising due to the channel's dispersive nature challenged with inter-symbol interference.
OBJECTIVES: The paper serves twin tasks, firstly addresses the challenges of signal interference using RL based model and secondly evaluates its effectiveness using different communication channels.
METHODS: The author proposes an improvement in channel equalization with the implementation of Whale Optimization Algorithm (WOA) followed by the Q-Learning model for Reinforcement Learning (RL) to identify the most suitable bit streams that will offer least interference.
RESULTS: Simulation analysis is performed against four existing works in terms of Bit Error Rate (BER), reflecting 20 to 30% improvement. The performance evaluation is executed using AWGN, Rician, Rayleigh, and Nakagami channels to evaluate BER against SNR, Eb/No, and Es/No.
CONCLUSION: Overall, the proposed work offers high-speed data transfer through a reliable communication channel with least BER under different scenarios.
Copyright © 2021 Swati Katwal et al., licensed to EAI. This is an open access article distributed under the terms of the Creative Commons Attribution license, which permits unlimited use, distribution and reproduction in any medium so long as the original work is properly cited.