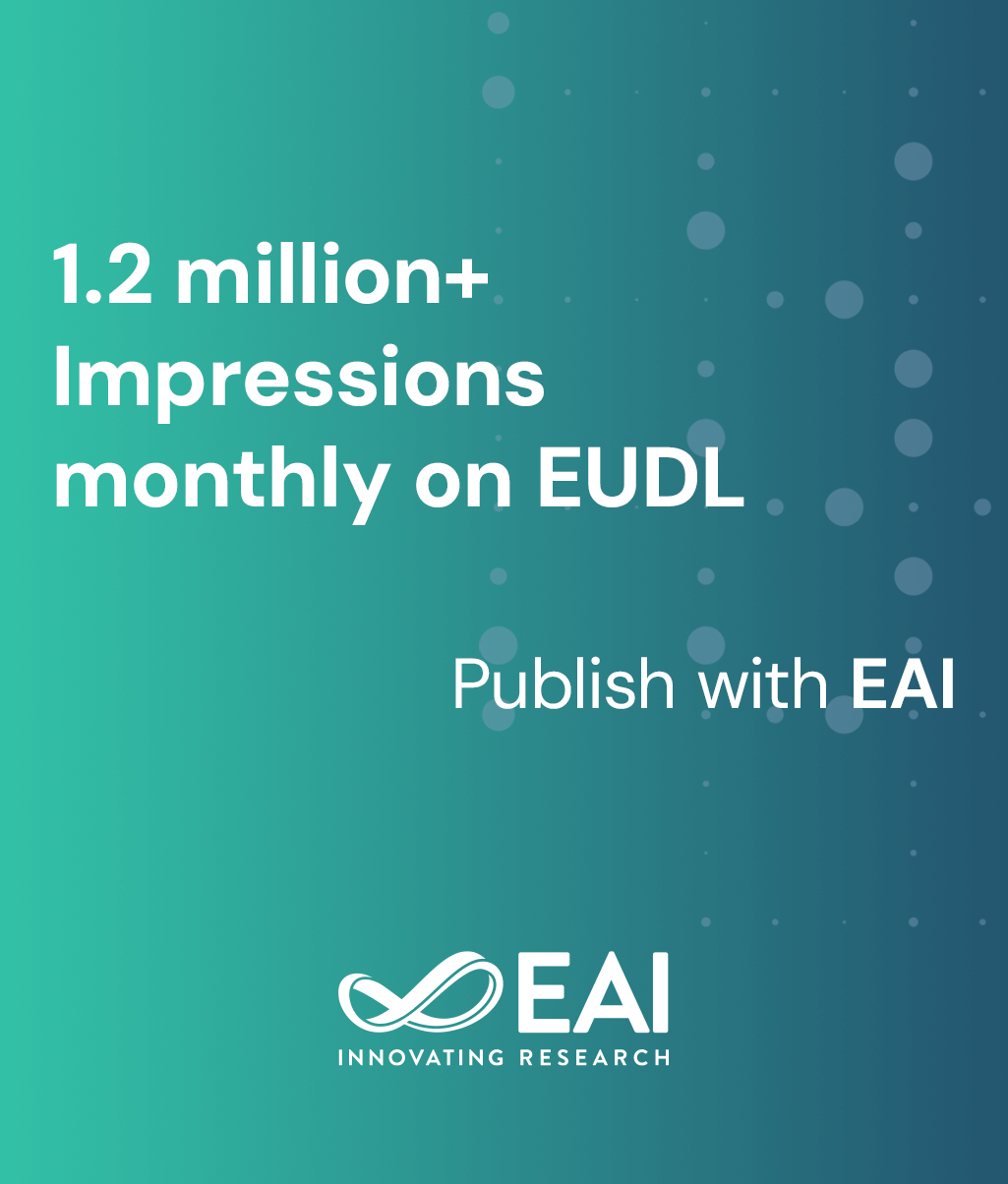
Research Article
Deep N-ary Error Correcting Output Codes
@INPROCEEDINGS{10.4108/eai.27-8-2020.2299197, author={Hao Zhang and Joey Tianyi Zhou and Tianying Wang and Ivor W. Tsang and Rick Siow Mong Goh}, title={Deep N-ary Error Correcting Output Codes}, proceedings={Proceedings of the 13th EAI International Conference on Mobile Multimedia Communications, Mobimedia 2020, 27-28 August 2020, Cyberspace}, publisher={EAI}, proceedings_a={MOBIMEDIA}, year={2020}, month={11}, keywords={deep n-ary ecoc ensemble learning multi-class classification}, doi={10.4108/eai.27-8-2020.2299197} }
- Hao Zhang
Joey Tianyi Zhou
Tianying Wang
Ivor W. Tsang
Rick Siow Mong Goh
Year: 2020
Deep N-ary Error Correcting Output Codes
MOBIMEDIA
EAI
DOI: 10.4108/eai.27-8-2020.2299197
Abstract
Ensemble learning consistently improves the performance of multi-class classification through aggregating a series of base classifiers. To this end, data-independent ensemble methods like Error Correcting Output Codes (ECOC) attract a increasing attention due to its easiness of implementation and parallelization. Specifically, traditional ECOCs and its general extension N-ary ECOC decomposes the original multi-class classification problem into a series of independent simpler classification subproblems. Unfortunately, integrating ECOCs, especially N-ary ECOC with deep neural networks, termed as deep N-ary ECOC, is not straightforward and yet fully exploited in the literature, due to high expense of training base learners. To facilitate training of N-ary ECOC with deep learning base learners, we further propose three different variants of parameter sharing architectures for deep N-ary ECOC. To verify the generalization ability of deep N-ary ECOC, we conduct experiments by varying the backbone with different deep neural networks architectures for both image and text classification task. Furthermore, extensive ablation studies on deep N-ary ECOC show its superior performance over other deep data-independent ensemble methods.