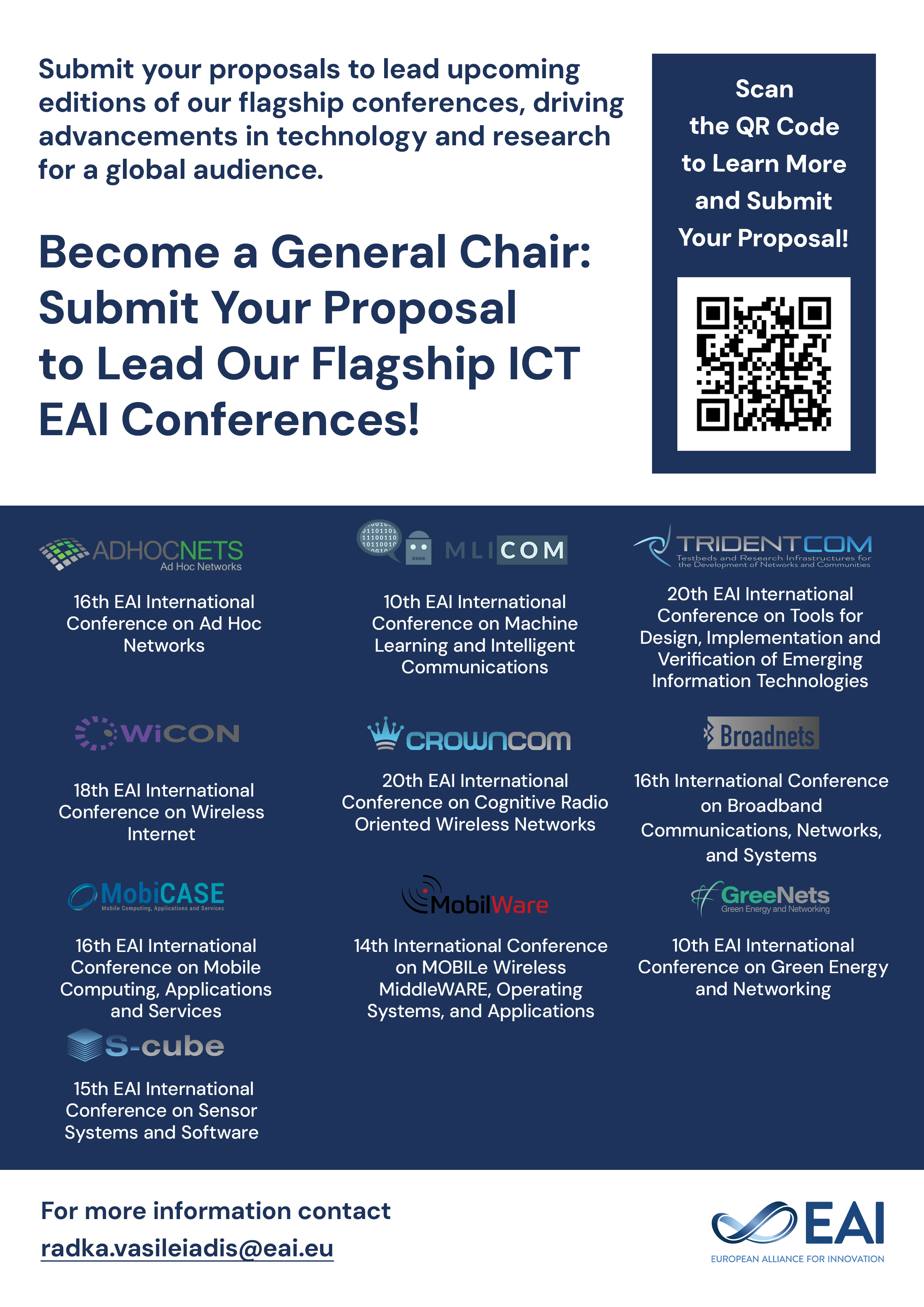
Research Article
Joint Cross-view Heterogeneous Discriminative Subspace Learning via Low-rank Representation
@INPROCEEDINGS{10.4108/eai.27-8-2020.2296558, author={Yu Ding and Ao Li}, title={Joint Cross-view Heterogeneous Discriminative Subspace Learning via Low-rank Representation}, proceedings={Proceedings of the 13th EAI International Conference on Mobile Multimedia Communications, Mobimedia 2020, 27-28 August 2020, Cyberspace}, publisher={EAI}, proceedings_a={MOBIMEDIA}, year={2020}, month={11}, keywords={multi-view discriminative analysis cross-view subspace learning low-rank representation}, doi={10.4108/eai.27-8-2020.2296558} }
- Yu Ding
Ao Li
Year: 2020
Joint Cross-view Heterogeneous Discriminative Subspace Learning via Low-rank Representation
MOBIMEDIA
EAI
DOI: 10.4108/eai.27-8-2020.2296558
Abstract
The cross-view data are very unforced to capture due to the face that different viewpoints or data collected from different sensors are already very common in recent years. However, cross-view data from different views present a significant difference, that is, cross-view data from different categories but in the same view have a higher similarity than the same category but within different views. To solve this problem, we have developed a dual low-rank representation framework to unbind these interleaved structures in a learning space. In addition, we consider that each cross-view sample of the same category is from isomorphic and heterogeneous information of two interlaced structures. Hence, we propose a powerful joint cross-view heterogeneous subspace feature learning model. In addition, the subspace learned by our algorithm contains more useful information and is more adaptable to cross-view data.