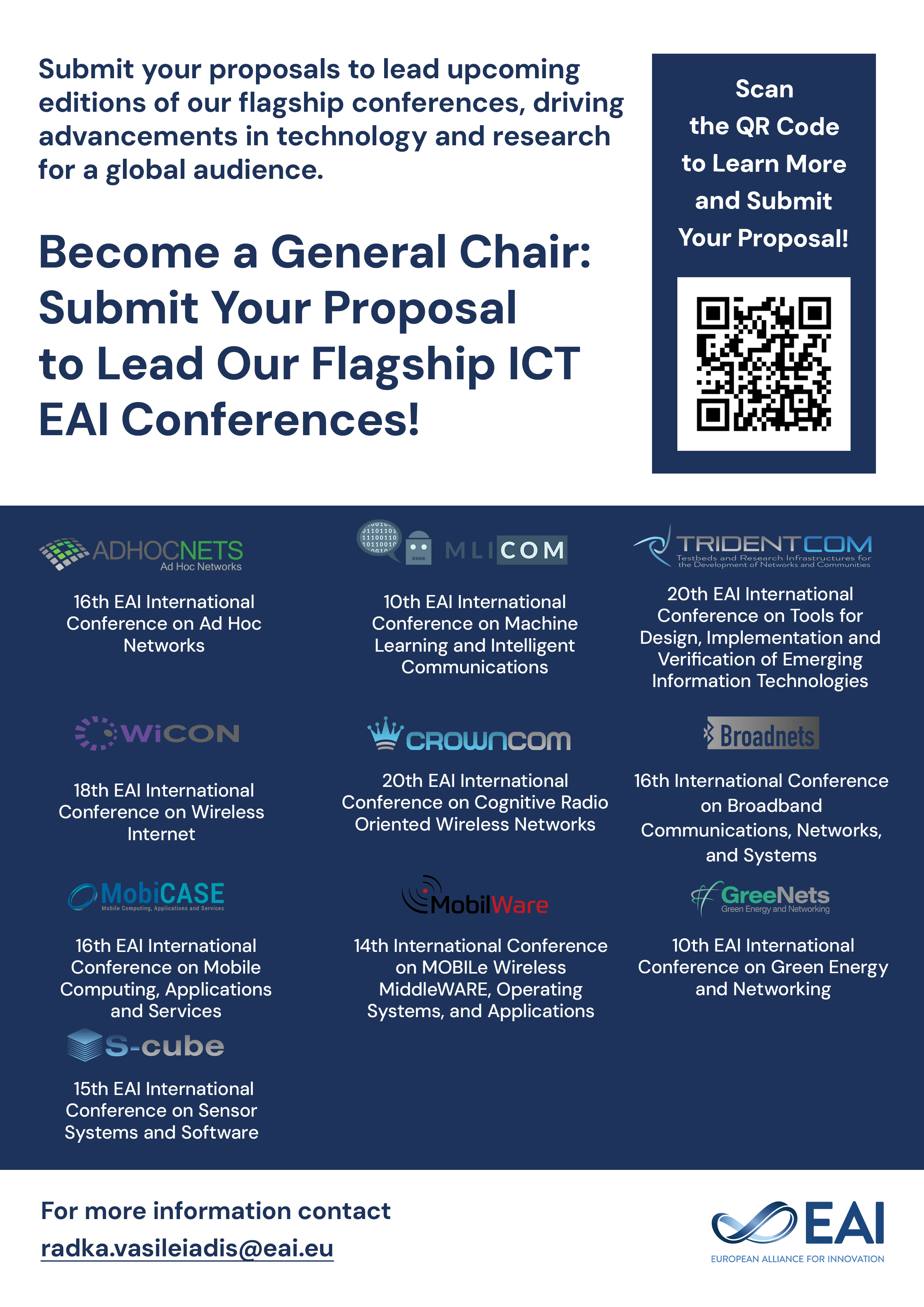
Research Article
Low Rank Sample Reconstruction-Based Semi-supervised Feature Subspace Learning
@INPROCEEDINGS{10.4108/eai.27-8-2020.2296557, author={Ruoqi An and AO LI}, title={Low Rank Sample Reconstruction-Based Semi-supervised Feature Subspace Learning }, proceedings={Proceedings of the 13th EAI International Conference on Mobile Multimedia Communications, Mobimedia 2020, 27-28 August 2020, Cyberspace}, publisher={EAI}, proceedings_a={MOBIMEDIA}, year={2020}, month={11}, keywords={image classification; subspace learning; graph semi-supervised learning; low rank sample reconstruction;}, doi={10.4108/eai.27-8-2020.2296557} }
- Ruoqi An
AO LI
Year: 2020
Low Rank Sample Reconstruction-Based Semi-supervised Feature Subspace Learning
MOBIMEDIA
EAI
DOI: 10.4108/eai.27-8-2020.2296557
Abstract
Feature subspaces have been widely valued and applied in image classification. However, majority conventional subspace learning approaches are supervised. The data containing only partial labeled samples will lead to unsatisfactory classification results in practical applications. To address the problems, in this paper, we design a graph semi-supervised feature subspace method named low rank sample reconstruction-based semi-supervised feature subspace learning, which combines graph-based semi-supervised learning and low lank sample reconstruction into the same model framework. The proposed method combines the low rank representation constraint into the reconstruction constraint, it then exploits the graph-based label propagation algorithm to predict the unlabeled labels to obtain the labels of all samples. The experimental results demonstrate that the robustness and the classification efficiency of our method.