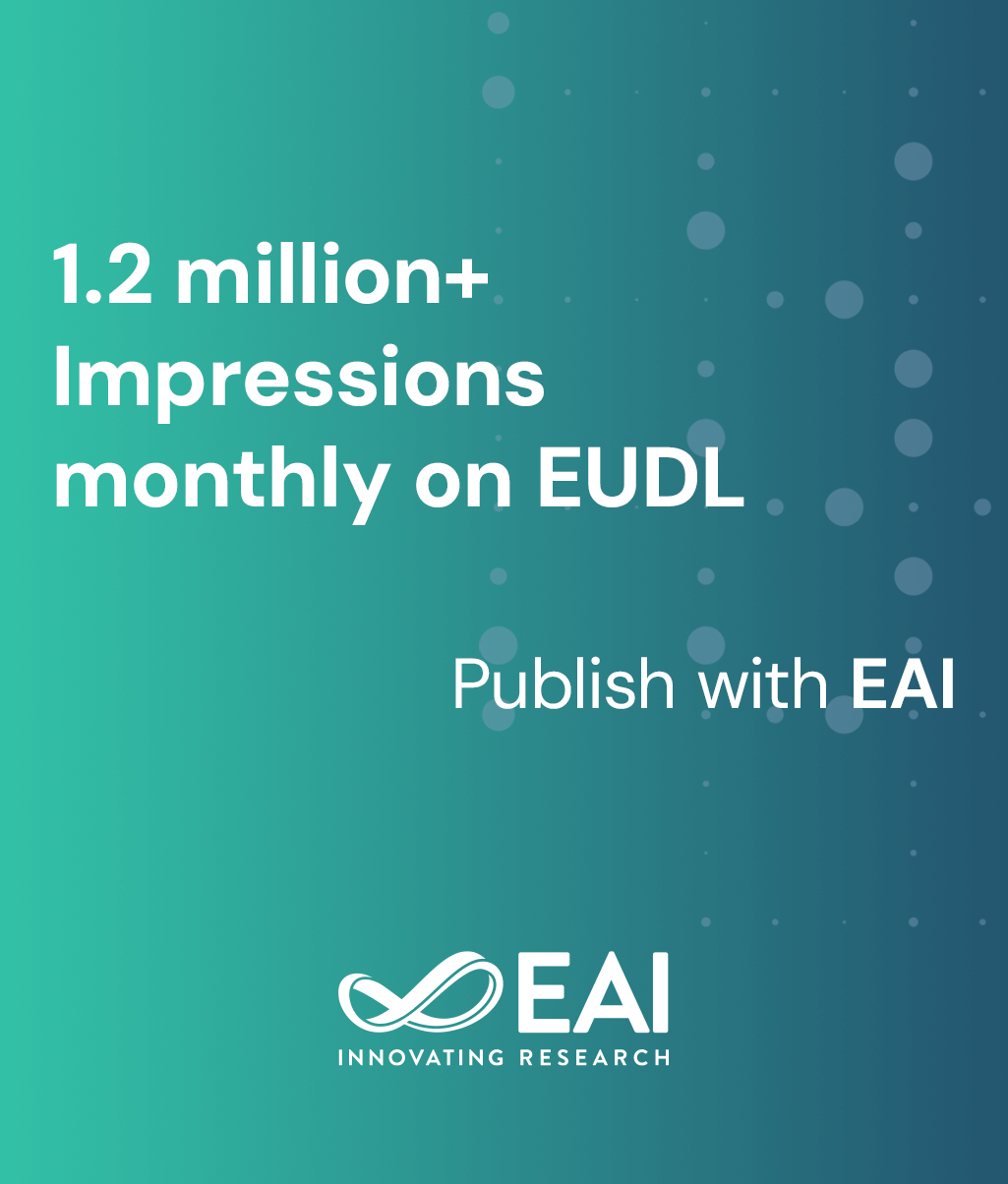
Research Article
Automatic Modulation Classification Using Hybrid Convolutional Neural Network
@INPROCEEDINGS{10.4108/eai.27-8-2020.2295027, author={Xiaofei Zhang and Li Anping and Chai Lu and Ma Xiaoying and Wei Meiying}, title={Automatic Modulation Classification Using Hybrid Convolutional Neural Network}, proceedings={Proceedings of the 13th EAI International Conference on Mobile Multimedia Communications, Mobimedia 2020, 27-28 August 2020, Cyberspace}, publisher={EAI}, proceedings_a={MOBIMEDIA}, year={2020}, month={11}, keywords={amc deep convolutional neural network hybrid convolutional neural networks spectral correlation features regular constellation images}, doi={10.4108/eai.27-8-2020.2295027} }
- Xiaofei Zhang
Li Anping
Chai Lu
Ma Xiaoying
Wei Meiying
Year: 2020
Automatic Modulation Classification Using Hybrid Convolutional Neural Network
MOBIMEDIA
EAI
DOI: 10.4108/eai.27-8-2020.2295027
Abstract
Automatic modulation classification (AMC) plays an essential role in signal demodulation and interference identifica-tion. In this paper, we propose a novel AMC method using the Hybrid Convolutional Neural Network (HCNN), which combines with two different convolutional neural networks (CNNs) jointly using various signal features. In the former CNN, spectral correlation features (SCFs) are generated as network input, to classify FSK and BPSK. In the latter CNN, the Attention-based Densely Convolutional Neural Network (AD-CNN), which is trained using regular constellation images (RCs), is proposed to identify the modulation formats that are hardly recognized by the former CNN, such as QPSK, 16-QAM and 64-QAM. The simulation results demonstrate that HCNN displays superior classification performance than existing AMC methods with lower computational complexity.