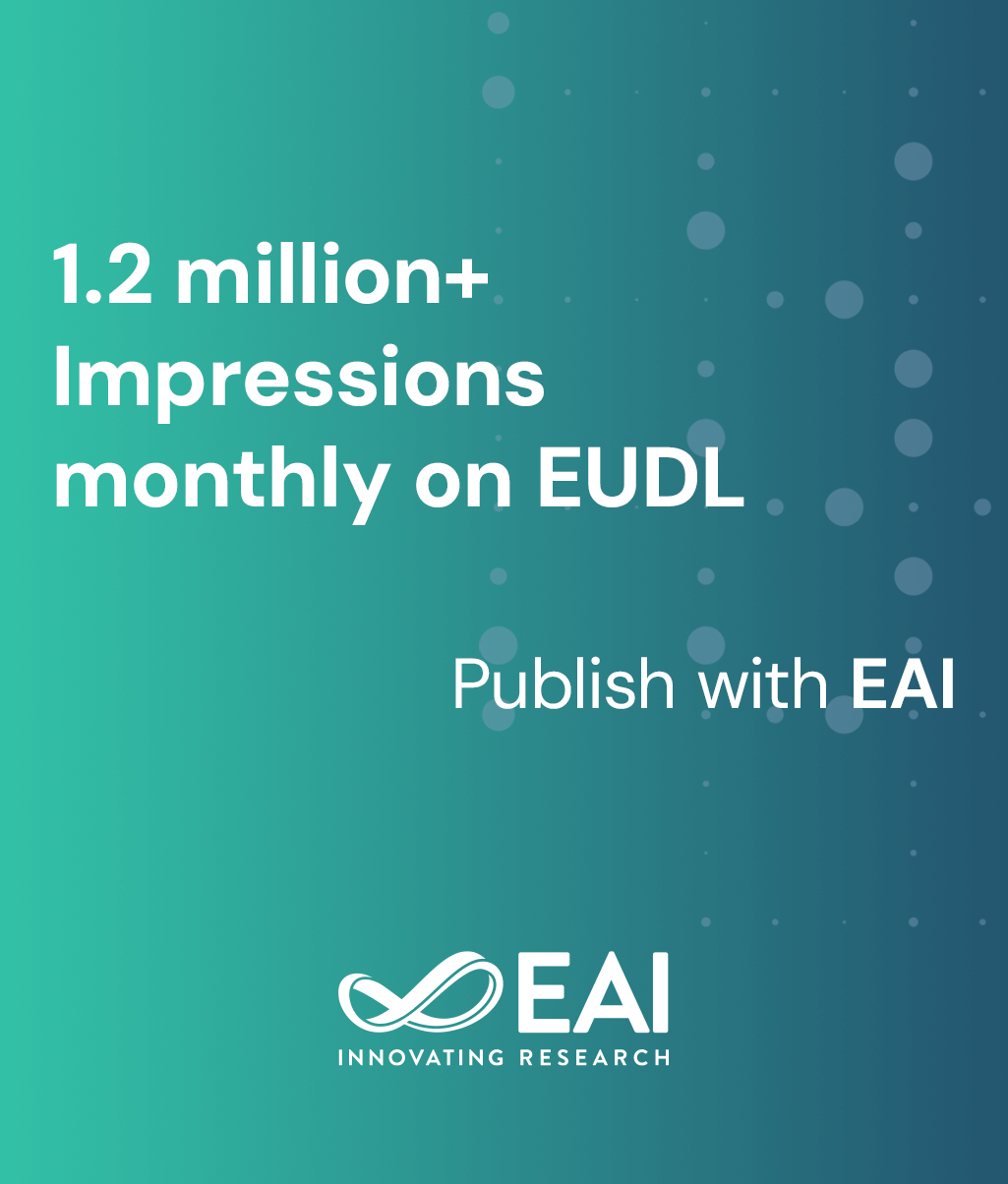
Research Article
Improved Loss Function for Defect Detection of Mobile Phone Screen
@INPROCEEDINGS{10.4108/eai.27-8-2020.2294669, author={wang zixuan and Li Chengyuan and Zhang Yifan and Zhang Fan and Chang Shuo and Li Yilei}, title={Improved Loss Function for Defect Detection of Mobile Phone Screen}, proceedings={Proceedings of the 13th EAI International Conference on Mobile Multimedia Communications, Mobimedia 2020, 27-28 August 2020, Cyberspace}, publisher={EAI}, proceedings_a={MOBIMEDIA}, year={2020}, month={11}, keywords={defect detection deep learning cross-entropy loss contrastive loss siamese network}, doi={10.4108/eai.27-8-2020.2294669} }
- wang zixuan
Li Chengyuan
Zhang Yifan
Zhang Fan
Chang Shuo
Li Yilei
Year: 2020
Improved Loss Function for Defect Detection of Mobile Phone Screen
MOBIMEDIA
EAI
DOI: 10.4108/eai.27-8-2020.2294669
Abstract
Due to the excellent feature learning and representation capabilities of deep learning, the method based on deep learning for mobile phone screen defect detection is gradually being applied to industrial detection. Nowadays, the cross-entropy loss commonly used in deep learning only focuses on the differences between different classes with less intra-class differences. It leads to poor discriminantive ability of the model when the similarity between training samples is high. The contrastive loss reduces the intra-class variations and can distinguish between similar objects from different classes. Given the above analysis, we propose a Siamese network for mobile phone screen defect detection (SMSDD) using combined contrastive loss and cross-entropy loss, thereby enhancing the discriminative ability of model. Numerical results show that SMSDD achieves comparable performance.