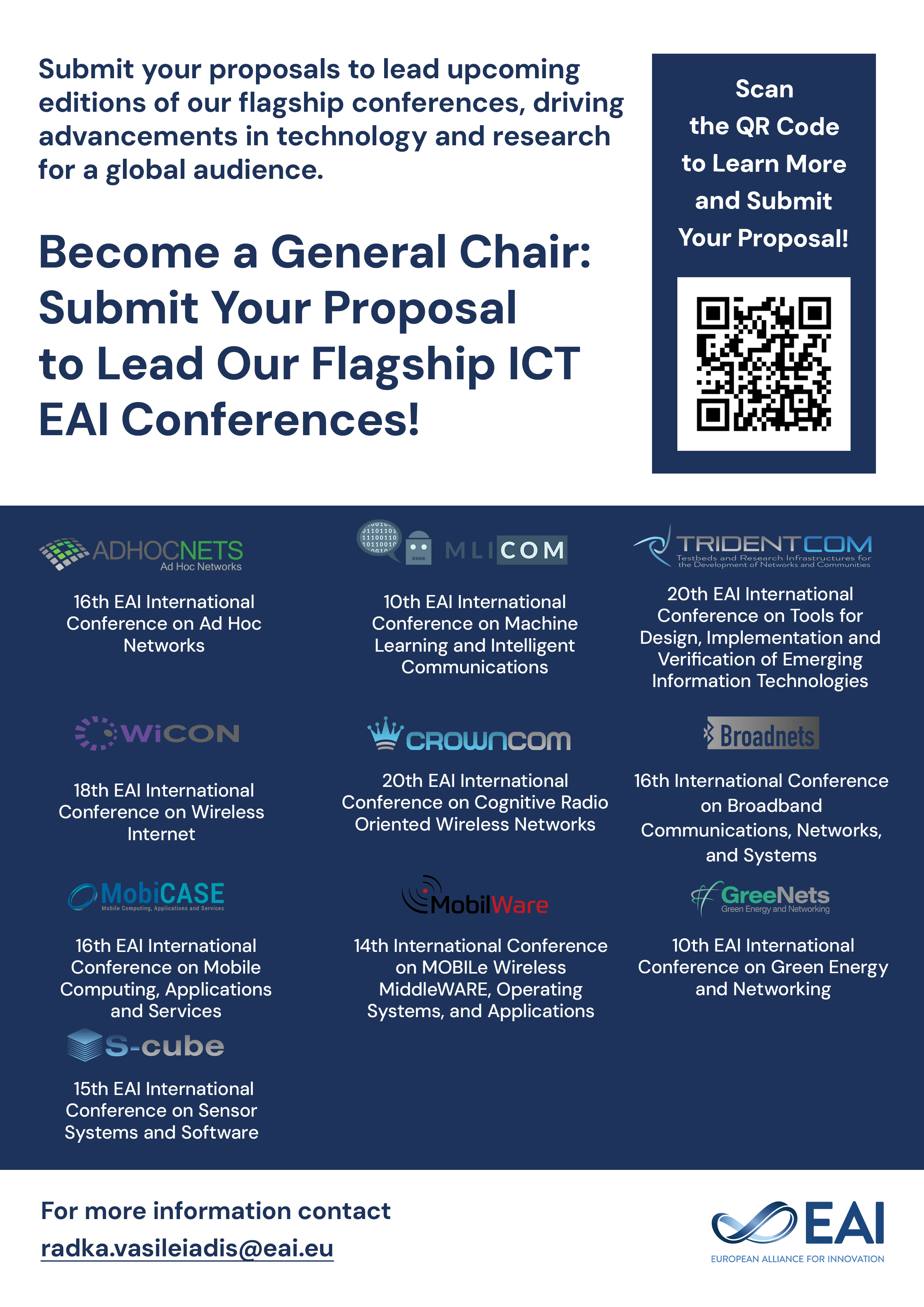
Research Article
The Detection of a Single Wet Rice Field Bund on Unmanned Aerial Vehicle Image Using a Convolutional Neural Network
@INPROCEEDINGS{10.4108/eai.27-11-2021.2315531, author={I Made Gede Sunarya and I Made Dendi Maysanjaya and Ida Bagus Made Yudha Wirawan and Gede Budi Setiawan}, title={The Detection of a Single Wet Rice Field Bund on Unmanned Aerial Vehicle Image Using a Convolutional Neural Network}, proceedings={Proceedings of the 4th International Conference on Vocational Education and Technology, IConVET 2021, 27 November 2021, Singaraja, Bali, Indonesia}, publisher={EAI}, proceedings_a={ICONVET}, year={2022}, month={2}, keywords={automatic detections bund rice field uav images deep learning cnn}, doi={10.4108/eai.27-11-2021.2315531} }
- I Made Gede Sunarya
I Made Dendi Maysanjaya
Ida Bagus Made Yudha Wirawan
Gede Budi Setiawan
Year: 2022
The Detection of a Single Wet Rice Field Bund on Unmanned Aerial Vehicle Image Using a Convolutional Neural Network
ICONVET
EAI
DOI: 10.4108/eai.27-11-2021.2315531
Abstract
This research is a preliminary study in the development of UAV in intelligent agriculture. This study aimed to detect Single Wet Rice Field Bund UAV Image Using CNN. The data used in this research were 170 images divided into Training (150), Validation (10), and Testing (10). The proposed stages were image acquisition, pre-processing, image labeling, training, and testing. The inputs were Wet Rice Field Bund UAV images, and the output was a bounding box as the result of rice bund detection. The training was carried out using Google Collaboratory and GPU features using CNN YOLO V5. In the training process, the best Recall, Precision, mAP0.5, mAP0.5:0.95 values were respectively 1, 1, 0.9952, 0.6358 with a total processing time of 0.858 hours. At the testing stage, Bounding boxes were detected at confidence thresholds of 0.1, 0.2, and at confidence 0.3, 0.4, 0.5 were able to detect a bounding box of 80%.