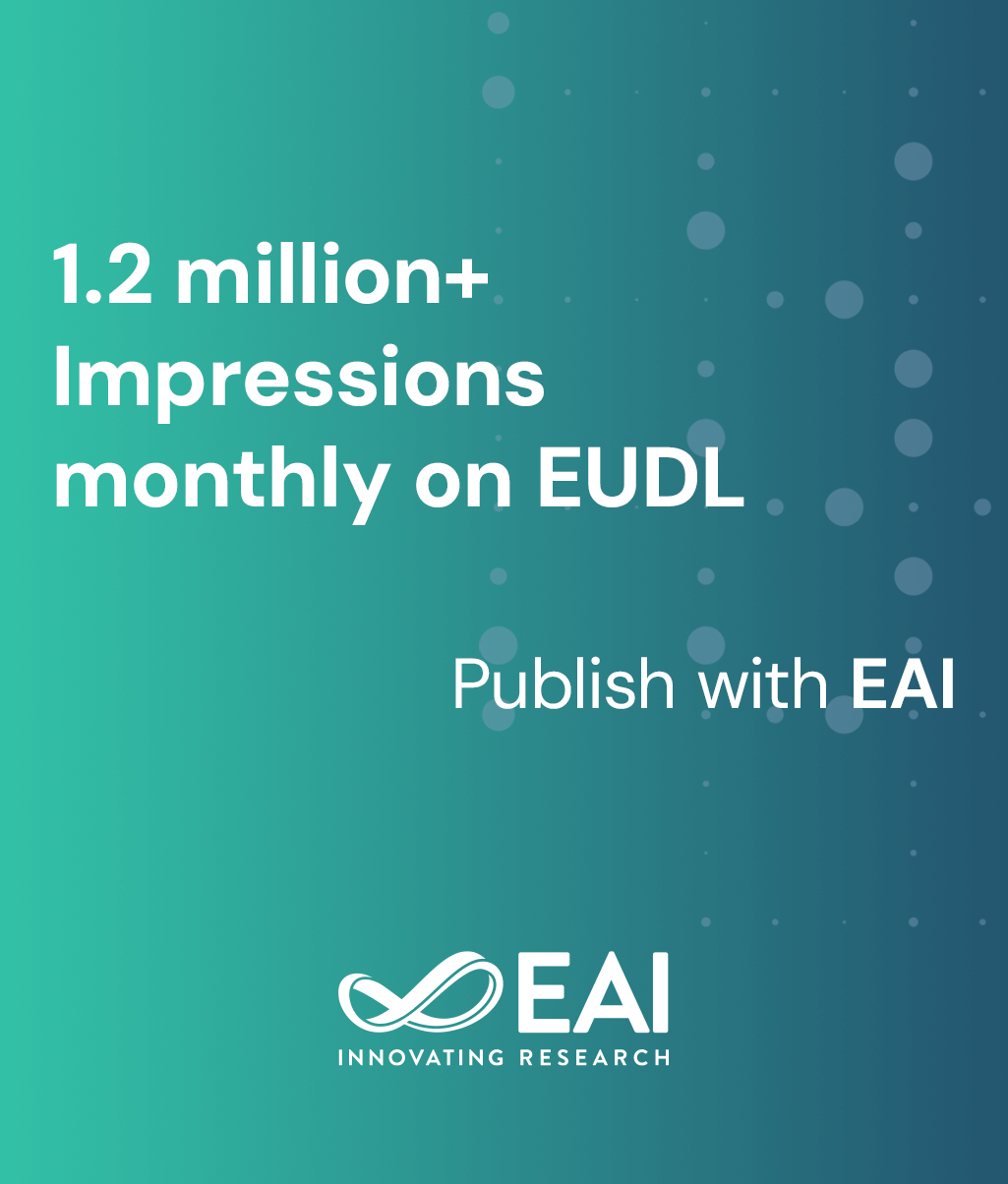
Research Article
Financial Asset Volatility Forecasting using LSTM with Intraday High-Low Price Information
@INPROCEEDINGS{10.4108/eai.26-5-2023.2334349, author={Yaohui Bai and Ping Bai and Xinyan Zhang and Huayang Li}, title={Financial Asset Volatility Forecasting using LSTM with Intraday High-Low Price Information}, proceedings={Proceedings of the 2nd International Conference on Mathematical Statistics and Economic Analysis, MSEA 2023, May 26--28, 2023, Nanjing, China}, publisher={EAI}, proceedings_a={MSEA}, year={2023}, month={7}, keywords={volatility forecasting garch lstm financial asset high-low price}, doi={10.4108/eai.26-5-2023.2334349} }
- Yaohui Bai
Ping Bai
Xinyan Zhang
Huayang Li
Year: 2023
Financial Asset Volatility Forecasting using LSTM with Intraday High-Low Price Information
MSEA
EAI
DOI: 10.4108/eai.26-5-2023.2334349
Abstract
In recent years, predicting the volatility of financial assets has received increasing attention due to the continuous development and increased volatility of financial markets. In this paper, we propose a volatility prediction model based on the Long Short-Term Memory (LSTM) model in deep learning, which considers the intraday high and low prices in financial asset sequences. To compare the performance of the proposed model, we also use the Generalized Autoregressive Conditional Heteroscedasticity (GARCH) model for comparison. By analyzing the data of the Shanghai Composite Index from January 1, 2015 to December 31, 2019, the results show that the proposed model outperforms the GARCH model, which is also reflected in the root-mean-square error of the two models. The proposed method exhibits promising results in predicting asset volatility and highlights the potential of LSTM models in the field of financial asset volatility prediction.