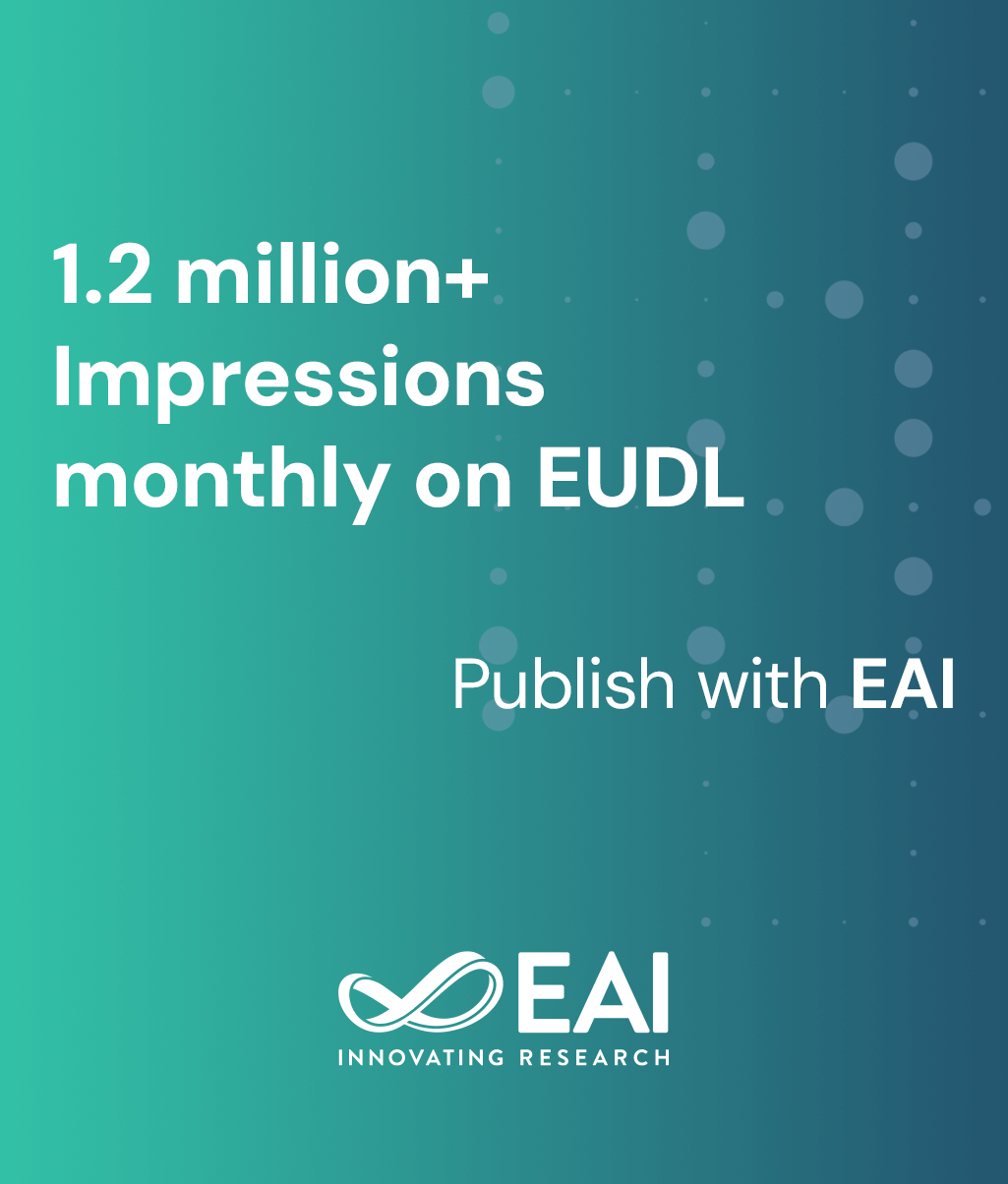
Research Article
Classification of Iris dataset using Bayesian Models
@INPROCEEDINGS{10.4108/eai.24-3-2022.2319063, author={Shruti Sharma and Khyati Chopra}, title={Classification of Iris dataset using Bayesian Models}, proceedings={Proceedings of the 3rd International Conference on ICT for Digital, Smart, and Sustainable Development, ICIDSSD 2022, 24-25 March 2022, New Delhi, India}, publisher={EAI}, proceedings_a={ICIDSSD}, year={2023}, month={5}, keywords={classification iris dataset bayesian model}, doi={10.4108/eai.24-3-2022.2319063} }
- Shruti Sharma
Khyati Chopra
Year: 2023
Classification of Iris dataset using Bayesian Models
ICIDSSD
EAI
DOI: 10.4108/eai.24-3-2022.2319063
Abstract
Classification of Iris flower dataset is the best known problem to be found in the pattern recognition literature. It contains four attributes of the flowers belonging to three different species. The objective is to design a model which can differentiate the species based on the attributes of the flowers. In this paper, we have used three different Bayesian models to perform this classification task viz. mixture model based method, hierarchical modeling based method and classical multinomial logistic regression. It was found out that mixture model based method was able to classify the data upto 83.33% accuracy without exploiting any prior knowledge except the number of components of mixtures (which is 3). Bayesian hierarchical model did use the knowledge of the membership of data but main advantage of using this technique was that we obtained the posterior predictive density which is not possible if we use logistic regression or mixture models. Logistic Regression significantly performed better as compared to mixture models with classification accuracy upto 98.33%.