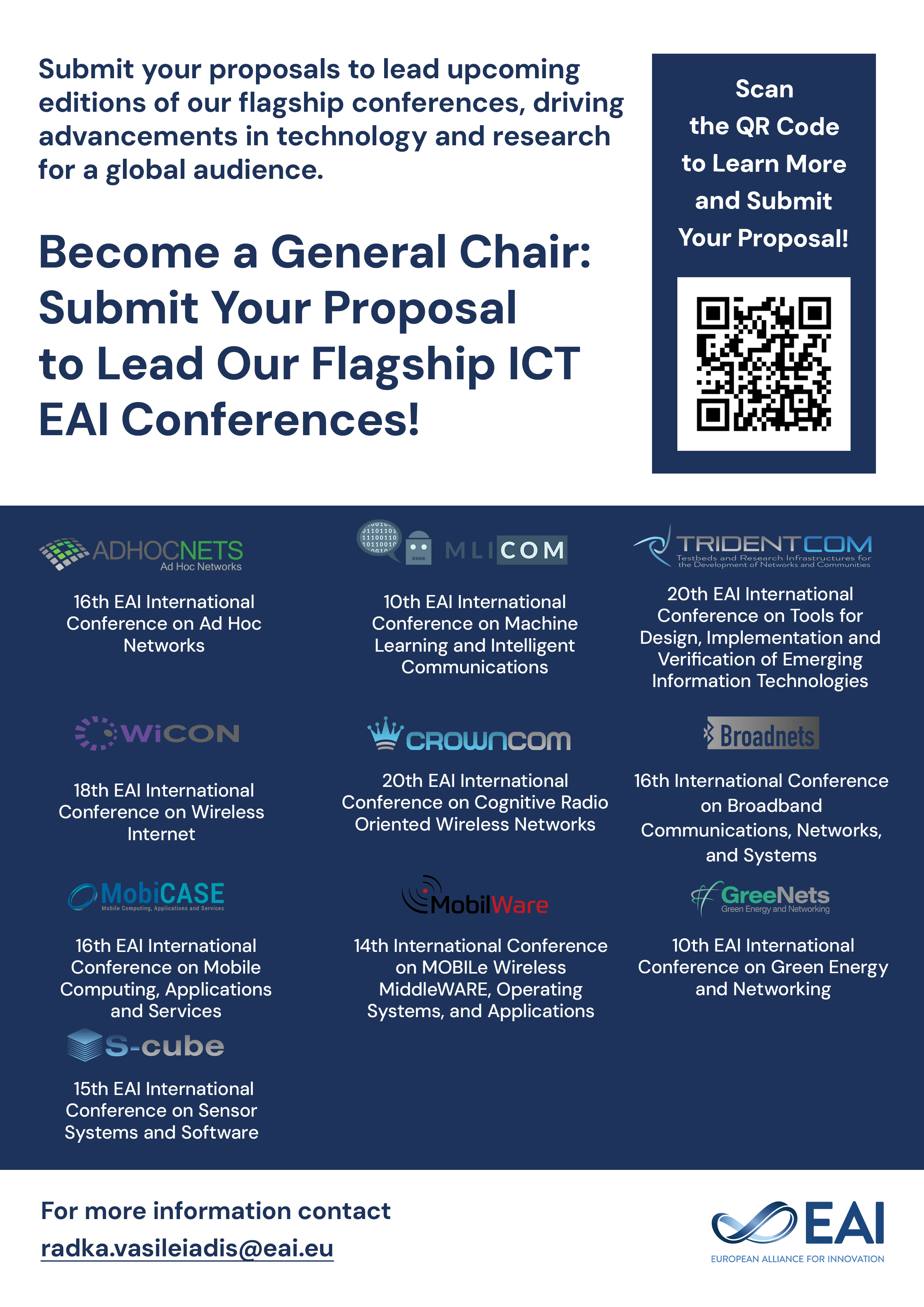
Research Article
Bayesian and LSTM Based Landfill Leakage Risk Study
@INPROCEEDINGS{10.4108/eai.24-11-2023.2343436, author={Zhaozhi Jia and Changxin Nai}, title={Bayesian and LSTM Based Landfill Leakage Risk Study}, proceedings={Proceedings of the International Conference on Industrial Design and Environmental Engineering, IDEE 2023, November 24--26, 2023, Zhengzhou, China}, publisher={EAI}, proceedings_a={IDEE}, year={2024}, month={2}, keywords={landfill leakage risk bayesian algorithm lstm model prediction}, doi={10.4108/eai.24-11-2023.2343436} }
- Zhaozhi Jia
Changxin Nai
Year: 2024
Bayesian and LSTM Based Landfill Leakage Risk Study
IDEE
EAI
DOI: 10.4108/eai.24-11-2023.2343436
Abstract
The problem of landfill leakage risk has garnered significant attention in the field of environmental protection. Accurate prediction of landfill leakage is crucial for environmental management and risk assessment. This thesis integrates traditional parametric rate-setting methods with deep learning, highlighting the application of modern machine learning in predictive modeling. Initially, a landfill leakage model is established. Subsequently, the Bayesian optimization algorithm is employed for parameter rate-setting to enhance the model's predictive accuracy. Following this, leakage prediction is conducted using an LSTM neural network based solely on measured data. Comparative analysis reveals that LSTM achieves higher prediction accuracy, unveiling the advantages and limitations of both methods. This provides a vital reference for landfill leakage risk assessment. The study analyzes the application of traditional intelligent optimization algorithm machine learning methods in environmental science and introduces new ideas for future research on similar problems.