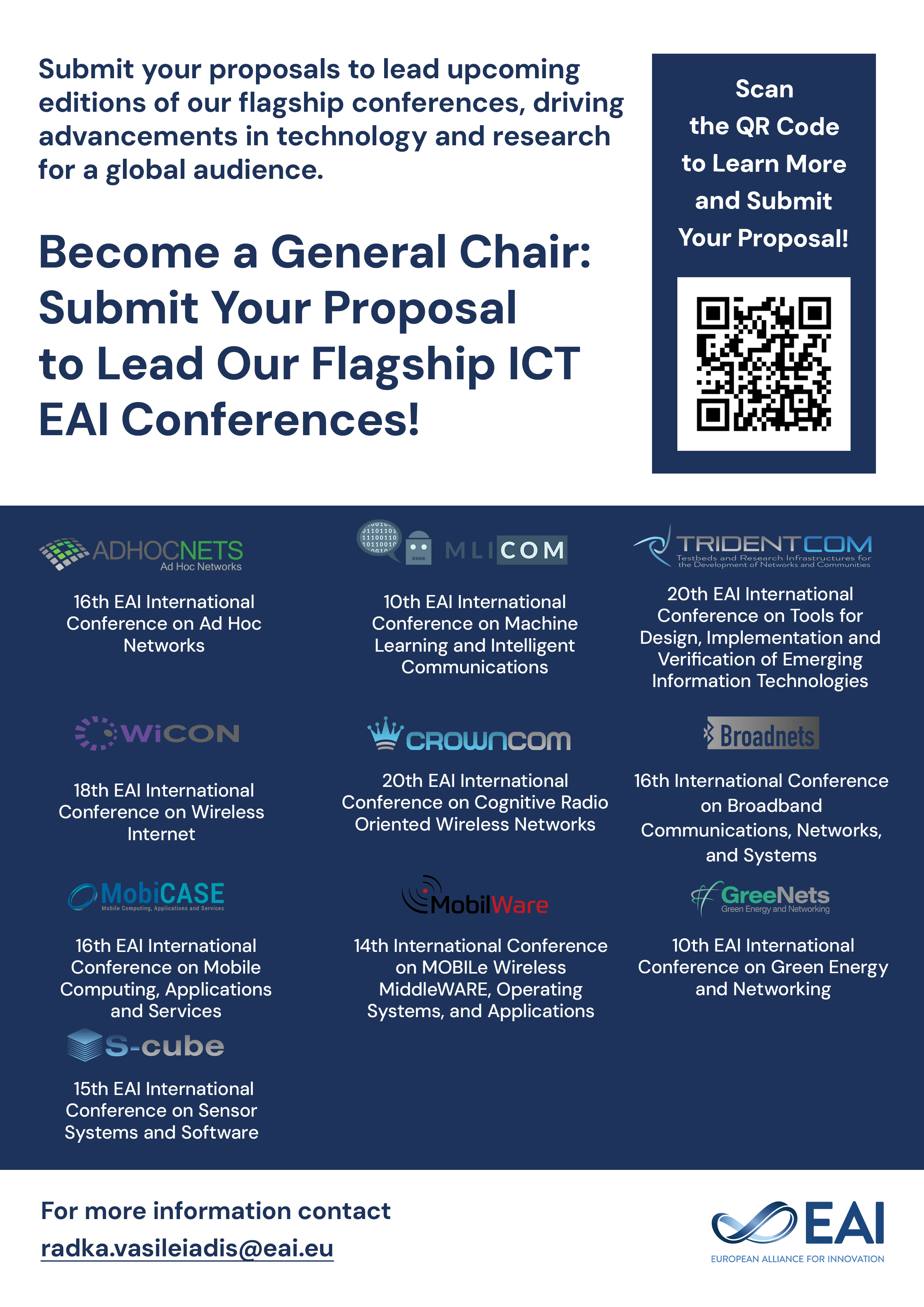
Research Article
The Step Construction of Copula Gaussian Multivariate and AR(1)-N.GARCH(1,1) Models
@INPROCEEDINGS{10.4108/eai.23-4-2018.2277604, author={Rezzy Eko Caraka and Hasbi Yasin and Toni Toharudin}, title={The Step Construction of Copula Gaussian Multivariate and AR(1)-N.GARCH(1,1) Models}, proceedings={Joint Workshop KO2PI and The 1st International Conference on Advance \& Scientific Innovation}, publisher={EAI}, proceedings_a={ICASI}, year={2018}, month={7}, keywords={copula multivariate garch time series}, doi={10.4108/eai.23-4-2018.2277604} }
- Rezzy Eko Caraka
Hasbi Yasin
Toni Toharudin
Year: 2018
The Step Construction of Copula Gaussian Multivariate and AR(1)-N.GARCH(1,1) Models
ICASI
EAI
DOI: 10.4108/eai.23-4-2018.2277604
Abstract
Copulas are a powerful tool in multivariate statistics. If copula functions used for modeling dependence between random variables, there is an immediate and obvious need to test whether the model can describe the data at hand accurately enough or not. Copulas involve several underlying functions: the marginal cumulative distribution functions (CDF) and a joint CDF. To estimating the copula functions, first issue consists in specifying how to estimate the margins separately. Moreover, some of these functions can be fully known. Depending on the assumptions made, some quantities have to be estimated parametric, semiparametric or even non-parametric. In this paper, we propose the step of parameter estimation in modeling Copula Gaussian Multivariate and AR (1)-N. GARCH (1,1) Models