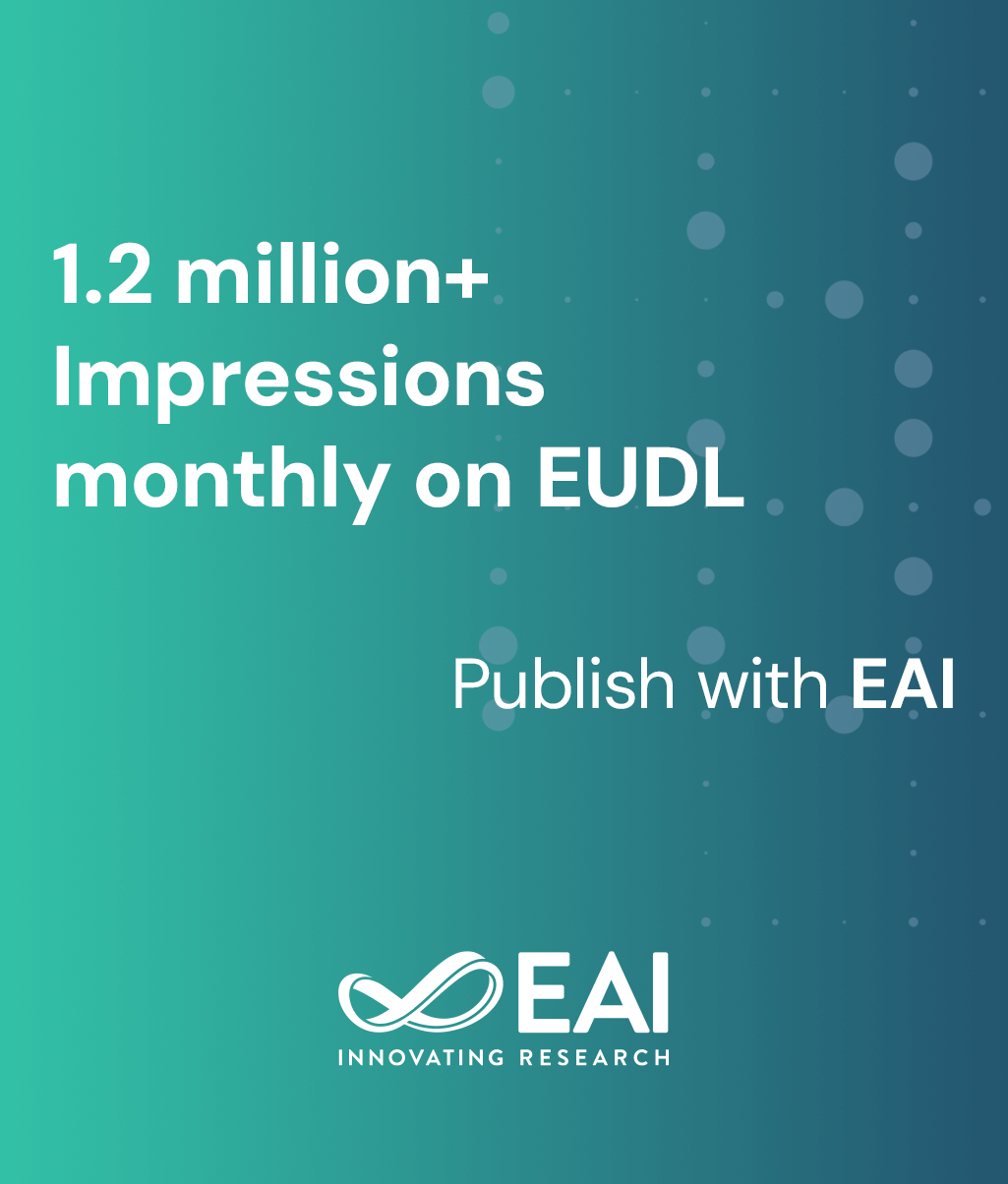
Research Article
Sequence Classification of Tweets with Transfer Learning via BERT in the Field of Disaster Management
@ARTICLE{10.4108/eai.23-3-2021.169071, author={Sumera Naaz and Zain Ul Abedin and Danish Raza Rizvi}, title={Sequence Classification of Tweets with Transfer Learning via BERT in the Field of Disaster Management}, journal={EAI Endorsed Transactions on Scalable Information Systems}, volume={8}, number={31}, publisher={EAI}, journal_a={SIS}, year={2021}, month={3}, keywords={BERT (Bidirectional Encoder Representation from Transformers), Tweet classification, Balanced Dataset, Imbalanced Dataset, Disaster Management, Natural Language Processing}, doi={10.4108/eai.23-3-2021.169071} }
- Sumera Naaz
Zain Ul Abedin
Danish Raza Rizvi
Year: 2021
Sequence Classification of Tweets with Transfer Learning via BERT in the Field of Disaster Management
SIS
EAI
DOI: 10.4108/eai.23-3-2021.169071
Abstract
Twitter is extensively used as an information-sharing platform during any kind of emergency like disasters etc. People tweet useful information about disaster-related events such as evacuations, volunteer need, help, warnings etc. This data is sometimes very useful for rescue teams, NGOs, military and various other government and private organisations who are tasked with responsibilities to save lives and provide volunteers. This data can also be used to analyze disaster behaviour. In this paper, we have collected labelled tweets from crisisLexT26 and crisisNLP and classified them into seven labels on the basis of information provided by them. The data was heavily skewed. So to improve the accuracy of classifiers, we have applied various techniques as a result of which we have created two datasets (Imbalanced and Balanced). We have compared the performance of various BERT-based models on these two datasets. For sequence classification, a balanced dataset performs better than an imbalanced dataset. We can improve accuracy of classifiers to great extent by adopting good data preprocessing and data splitting techniques.
Copyright © 2021 Sumera Naaz et al., licensed to EAI. This is an open access article distributed under the terms of the Creative Commons Attribution license (http://creativecommons.org/licenses/by/3.0/), which permits unlimited use, distribution and reproduction in any medium so long as the original work is properly cited.