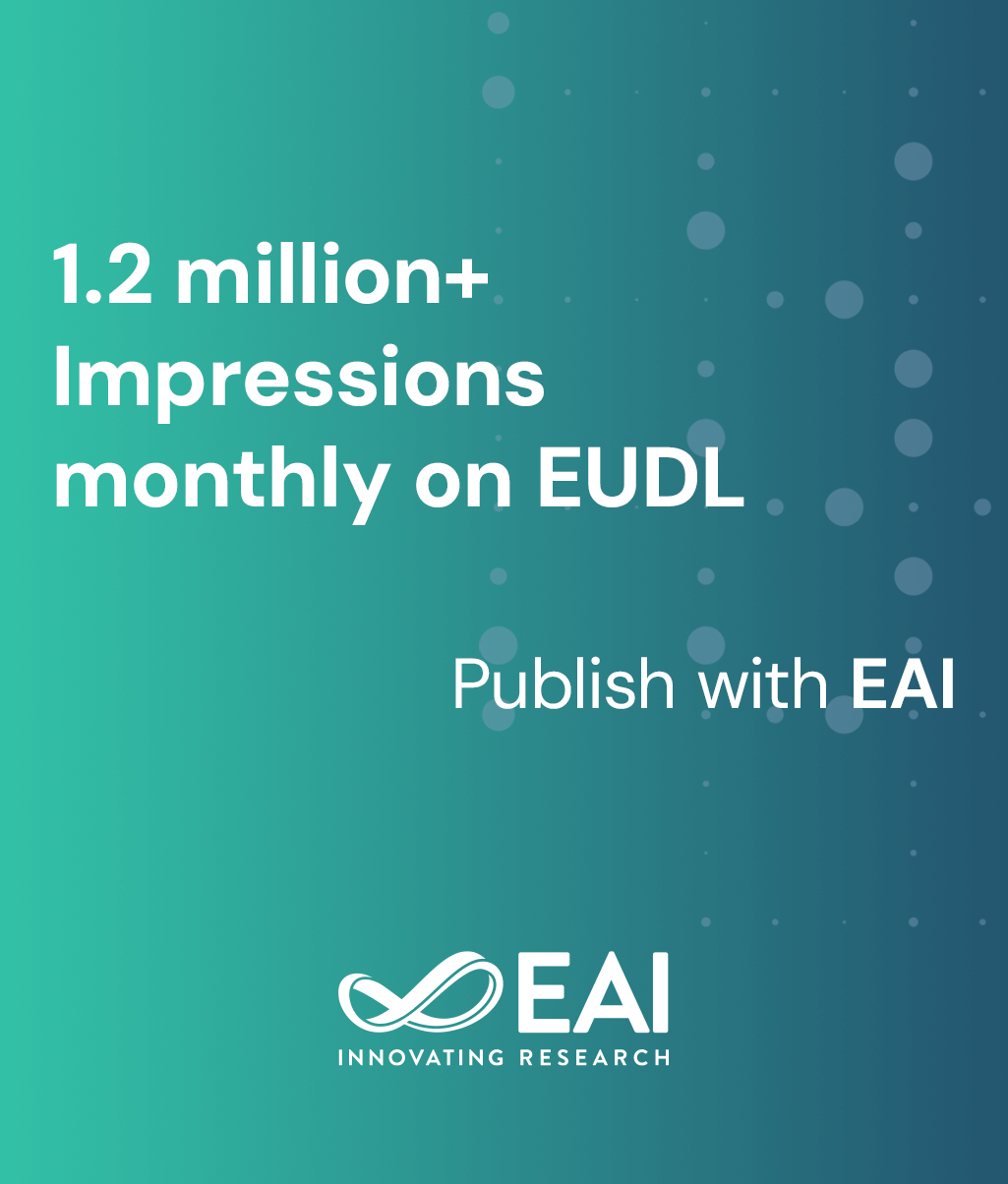
Research Article
Strong-weak Dual-branch Network with Hard-aware Loss for Long-tailed Classification
@INPROCEEDINGS{10.4108/eai.23-12-2022.2329170, author={Qingheng Zhang and Haibo Ye}, title={Strong-weak Dual-branch Network with Hard-aware Loss for Long-tailed Classification }, proceedings={Proceedings of the 2nd International Conference on Internet Technology and Educational Informatization, ITEI 2022, December 23-25, 2022, Harbin, China}, publisher={EAI}, proceedings_a={ITEI}, year={2023}, month={6}, keywords={long-tailed distribution; dual-branch network; hard-aware loss}, doi={10.4108/eai.23-12-2022.2329170} }
- Qingheng Zhang
Haibo Ye
Year: 2023
Strong-weak Dual-branch Network with Hard-aware Loss for Long-tailed Classification
ITEI
EAI
DOI: 10.4108/eai.23-12-2022.2329170
Abstract
Natural data usually exhibit a long-tailed distribution, with the minority classes occupying the majority of the data, while the majority classes have few samples. Although deep learning has made remarkable progress in visual recognition on large-scale balanced datasets, it remains challenging to model long-tailed distributions. Recent multi-branch methods have shown great potential to address long-tailed problems. We find that these methods work due to the difference between branches, so we also propose a new structure called Strong-weak Dual-branch Network (SDN) to enlarge the difference between branches. In particular, our SDN is equipped with a new Difference to Classification (D2C) learning strategy, designed to amplify the differences between the branches first, and then pay attention to classification. In addition, we propose a new Hard-aware Loss (HL) for the sake of handling hard examples. Our SDNHL method achieves SOTA on four long-tailed datasets: CIFAR-10-LT, CIFAR-100-LT, ImageNet-LT and iNaturalist 2018.