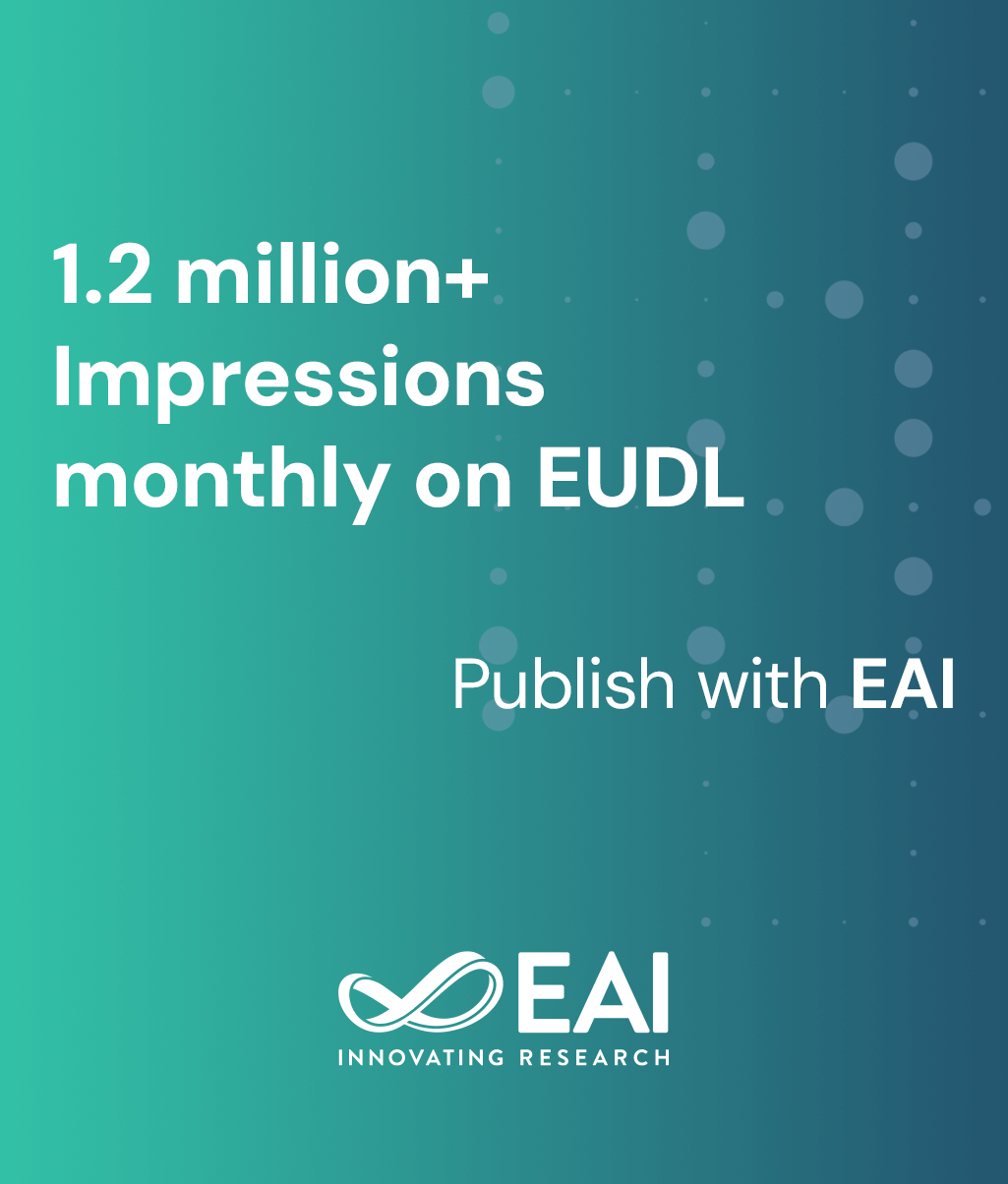
Research Article
Employment Information Recommendation Model Based on Improved Density Clustering
@INPROCEEDINGS{10.4108/eai.23-12-2022.2329117, author={Guiqin Duan and Yuxian Wang and Liying Guo and Chengsong Zou}, title={Employment Information Recommendation Model Based on Improved Density Clustering}, proceedings={Proceedings of the 2nd International Conference on Internet Technology and Educational Informatization, ITEI 2022, December 23-25, 2022, Harbin, China}, publisher={EAI}, proceedings_a={ITEI}, year={2023}, month={6}, keywords={cluster analysis; density clustering; selection of initial cluster center; employment recommendation; education informatization}, doi={10.4108/eai.23-12-2022.2329117} }
- Guiqin Duan
Yuxian Wang
Liying Guo
Chengsong Zou
Year: 2023
Employment Information Recommendation Model Based on Improved Density Clustering
ITEI
EAI
DOI: 10.4108/eai.23-12-2022.2329117
Abstract
Given employment difficulties and low employment quality of college students under the background of higher vocational enrollment expansion, an employment information recommendation model based on improved density clustering was designed. A weighted user similarity calculation method based on professional similarity, job intention similarity, and professional ability similarity was given, and the quantitative analysis process of professional ability was optimized. Considering that high-density samples are closely surrounded by low-density samples, a new density clustering algorithm was proposed to improve the accuracy of employment recommendations. The practice has proved that this model can effectively mine students’ employment information, and reduce the iteration times of the clustering algorithm, accompanied by high information retrieval integrity and good personalized employment recommendation performance.